Equivariant Data Augmentation for Generalization in Offline Reinforcement Learning
CoRR(2023)
摘要
We present a novel approach to address the challenge of generalization in offline reinforcement learning (RL), where the agent learns from a fixed dataset without any additional interaction with the environment. Specifically, we aim to improve the agent's ability to generalize to out-of-distribution goals. To achieve this, we propose to learn a dynamics model and check if it is equivariant with respect to a fixed type of transformation, namely translations in the state space. We then use an entropy regularizer to increase the equivariant set and augment the dataset with the resulting transformed samples. Finally, we learn a new policy offline based on the augmented dataset, with an off-the-shelf offline RL algorithm. Our experimental results demonstrate that our approach can greatly improve the test performance of the policy on the considered environments.
更多查看译文
关键词
reinforcement,generalization,offline
AI 理解论文
溯源树
样例
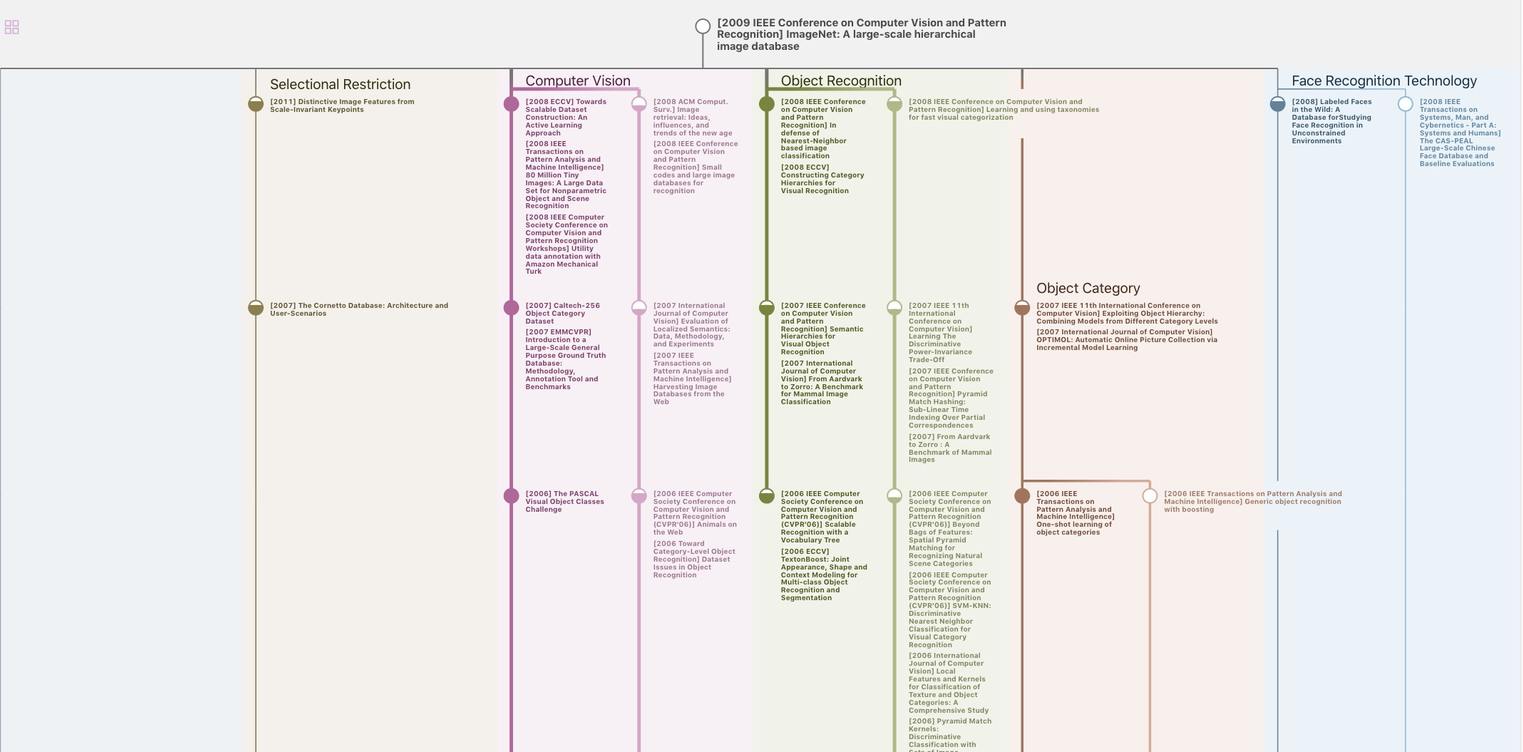
生成溯源树,研究论文发展脉络
Chat Paper
正在生成论文摘要