HDTR-Net: A Real-Time High-Definition Teeth Restoration Network for Arbitrary Talking Face Generation Methods
PATTERN RECOGNITION AND COMPUTER VISION, PRCV 2023, PT XI(2024)
摘要
Talking Face Generation (TFG) aims to reconstruct facial movements to achieve high natural lip movements from audio and facial features that are under potential connections. Existing TFG methods have made significant advancements to produce natural and realistic images. However, most work rarely takes visual quality into consideration. It is challenging to ensure lip synchronization while avoiding visual quality degradation in cross-modal generation methods. To address this issue, we propose a universal High-Definition Teeth Restoration Network, dubbed HDTR-Net, for arbitrary TFG methods. HDTR-Net can enhance teeth regions at an extremely fast speed while maintaining synchronization, and temporal consistency. In particular, we propose a Fine-Grained Feature Fusion (FGFF) module to effectively capture fine texture feature information around teeth and surrounding regions, and use these features to fine-grain the feature map to enhance the clarity of teeth. Extensive experiments show that our method can be adapted to arbitrary TFG methods without suffering from lip synchronization and frame coherence. Another advantage of HDTR-Net is its real-time generation ability. Also under the condition of high-definition restoration of talking face video synthesis, its inference speed is 300% faster than the current state-of-the-art face restoration based on super-resolution. Our code and trained models are released at https://github.com/yylgoodlucky/HDTR.
更多查看译文
关键词
High-Definition Teeth Restoration Network,Talking Face Generation,Teeth Restoration,Visual Quality
AI 理解论文
溯源树
样例
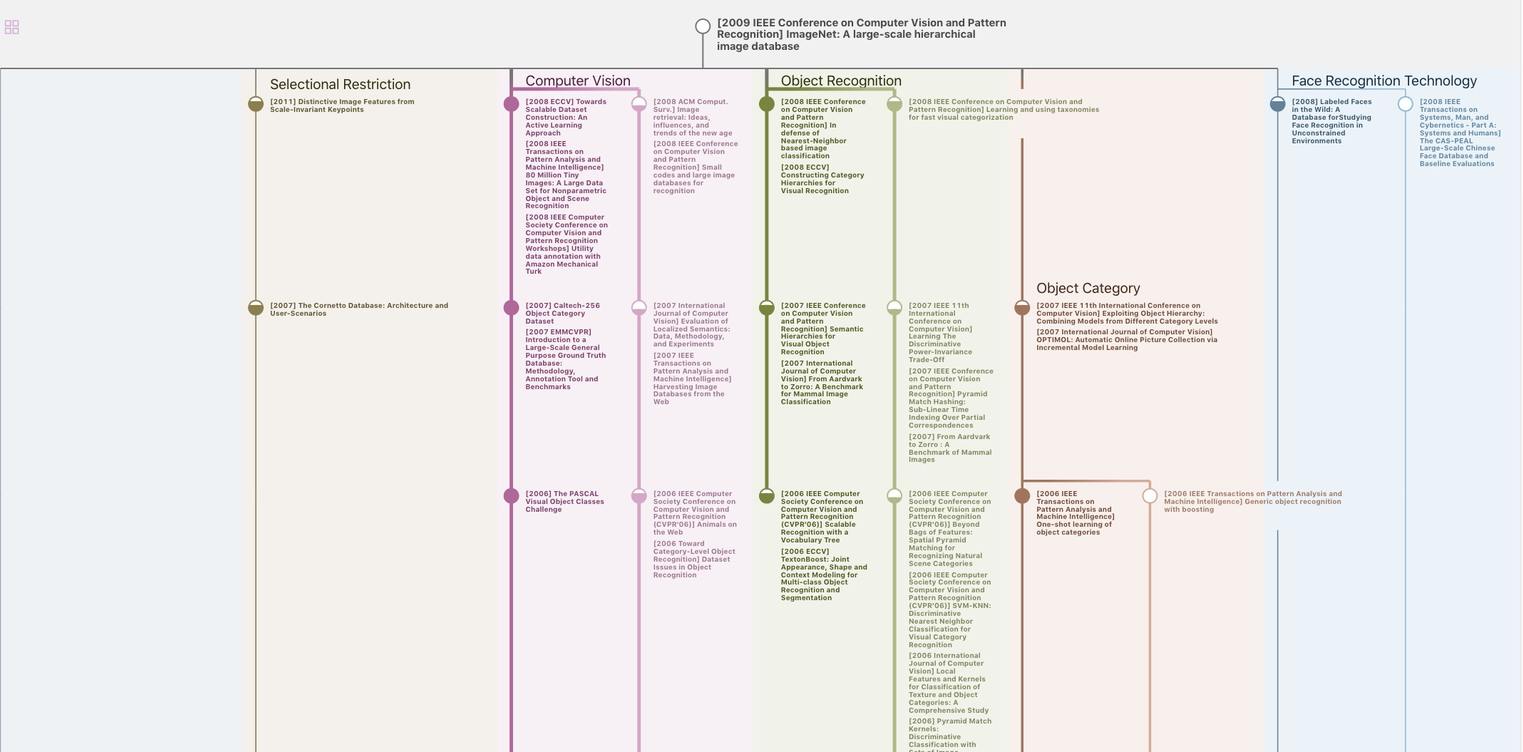
生成溯源树,研究论文发展脉络
Chat Paper
正在生成论文摘要