Transmission matrix parameter estimation of COVID-19 evolution with age compartments using ensemble-based data assimilation
arXiv (Cornell University)(2023)
摘要
The COVID-19 pandemic and its multiple outbreaks have challenged governments around the world. Much of the epidemiological modeling was based on pre-pandemic contact information of the population, which changed drastically due to governmental health measures, so called non-pharmaceutical interventions made to reduce transmission of the virus, like social distancing and complete lockdown. In this work, we evaluate an ensemble-based data assimilation framework applied to a meta-population model to infer the transmission of the disease between different population agegroups. We perform a set of idealized twin-experiments to investigate the performance of different possible parameterizations of the transmission matrix. These experiments show that it is not possible to unambiguously estimate all the independent parameters of the transmission matrix. However, under certain parameterizations, the transmission matrix in an age-compartmental model can be estimated. These estimated parameters lead to an increase of forecast accuracy in agegroups compartments assimilating age-dependent accumulated cases and deaths observed in Argentina compared to a single-compartment model, and reliable estimations of the effective reproduction number. The age-dependent data assimilation and forecasting of virus transmission may be important for an accurate prediction and diagnosis of health care demand.
更多查看译文
关键词
ensemble-based
AI 理解论文
溯源树
样例
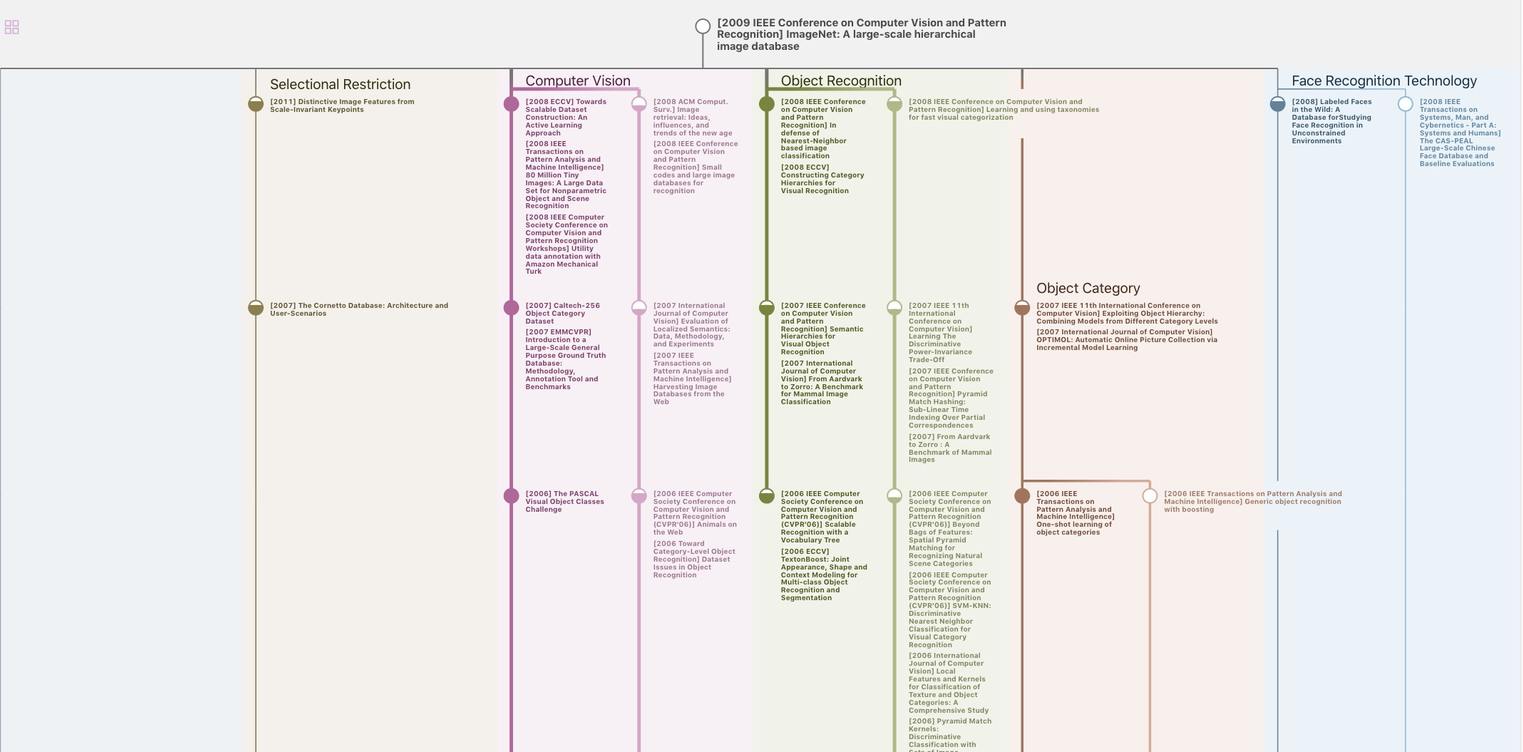
生成溯源树,研究论文发展脉络
Chat Paper
正在生成论文摘要