ETP: Learning Transferable ECG Representations via ECG-Text Pre-training
ICASSP 2024 - 2024 IEEE International Conference on Acoustics, Speech and Signal Processing (ICASSP)(2023)
摘要
In the domain of cardiovascular healthcare, the Electrocardiogram (ECG) serves as a critical, non-invasive diagnostic tool. Although recent strides in self-supervised learning (SSL) have been promising for ECG representation learning, these techniques often require annotated samples and struggle with classes not present in the fine-tuning stages. To address these limitations, we introduce ECG-Text Pre-training (ETP), an innovative framework designed to learn cross-modal representations that link ECG signals with textual reports. For the first time, this framework leverages the zero-shot classification task in the ECG domain. ETP employs an ECG encoder along with a pre-trained language model to align ECG signals with their corresponding textual reports. The proposed framework excels in both linear evaluation and zero-shot classification tasks, as demonstrated on the PTB-XL and CPSC2018 datasets, showcasing its ability for robust and generalizable cross-modal ECG feature learning.
更多查看译文
关键词
Electrocardiogram,ECG-Text Pre-training,Self-supervised Learning
AI 理解论文
溯源树
样例
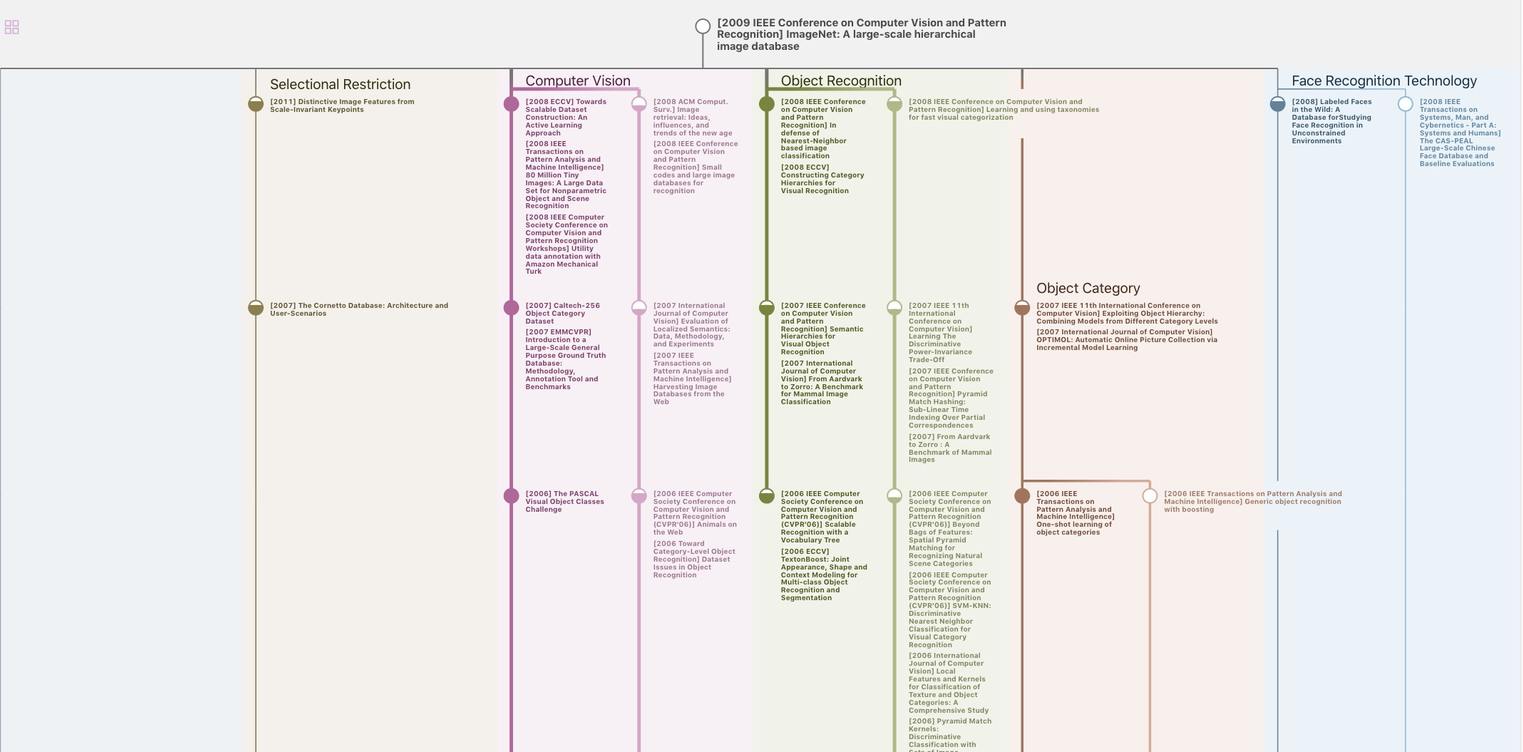
生成溯源树,研究论文发展脉络
Chat Paper
正在生成论文摘要