A Retrospective Cohort Study: Predicting 90-Day Mortality for ICU Trauma Patients with a Machine Learning Algorithm Using XGBoost Using MIMIC-III Database
Journal of multidisciplinary healthcare(2023)
摘要
Objective: The aim of this study was to develop and validate a machine learning-based predictive model that predicts 90-day mortality in ICU trauma patients.Methods: Data of patients with severe trauma were extracted from the Medical Information Mart for Intensive Care III (MIMIC-III) database. The performances of mortality prediction models generated using nine machine learning extreme gradient boosting (XGBoost), logistic regression, random forest, AdaBoost, multilayer perceptron (MLP) neural networks, support vector machine (SVM), light gradient boosting machine (GBM), k nearest neighbors (KNN) and gaussian naive bayes (GNB). The performance of the model was evaluated in terms of discrimination, calibration and clinical application.Results: We found that the accuracy, sensitivity, specificity, PPV, NPV and F1 score of our proposed XGBoost model were 82.8%, 79.7%, 77.6%, 51.2%, 91.5% and 0.624, respectively. Among the nine models, the XGBoost model performed best. Compared with traditional logistic regression, the calibration curves of the XGBoost model and decision curve analysis (DCA) performed well. Conclusion: Our study shows that the XGBoost model outperforms other machine learning models in predicting 90-day mortality in trauma patients. It can be used to assist clinicians in the early identification of mortality risk factors and early intervention to reduce mortality.
更多查看译文
关键词
MIMIC-III,severe trauma patient,intensive care unit,XGBoost,mortality,prediction model
AI 理解论文
溯源树
样例
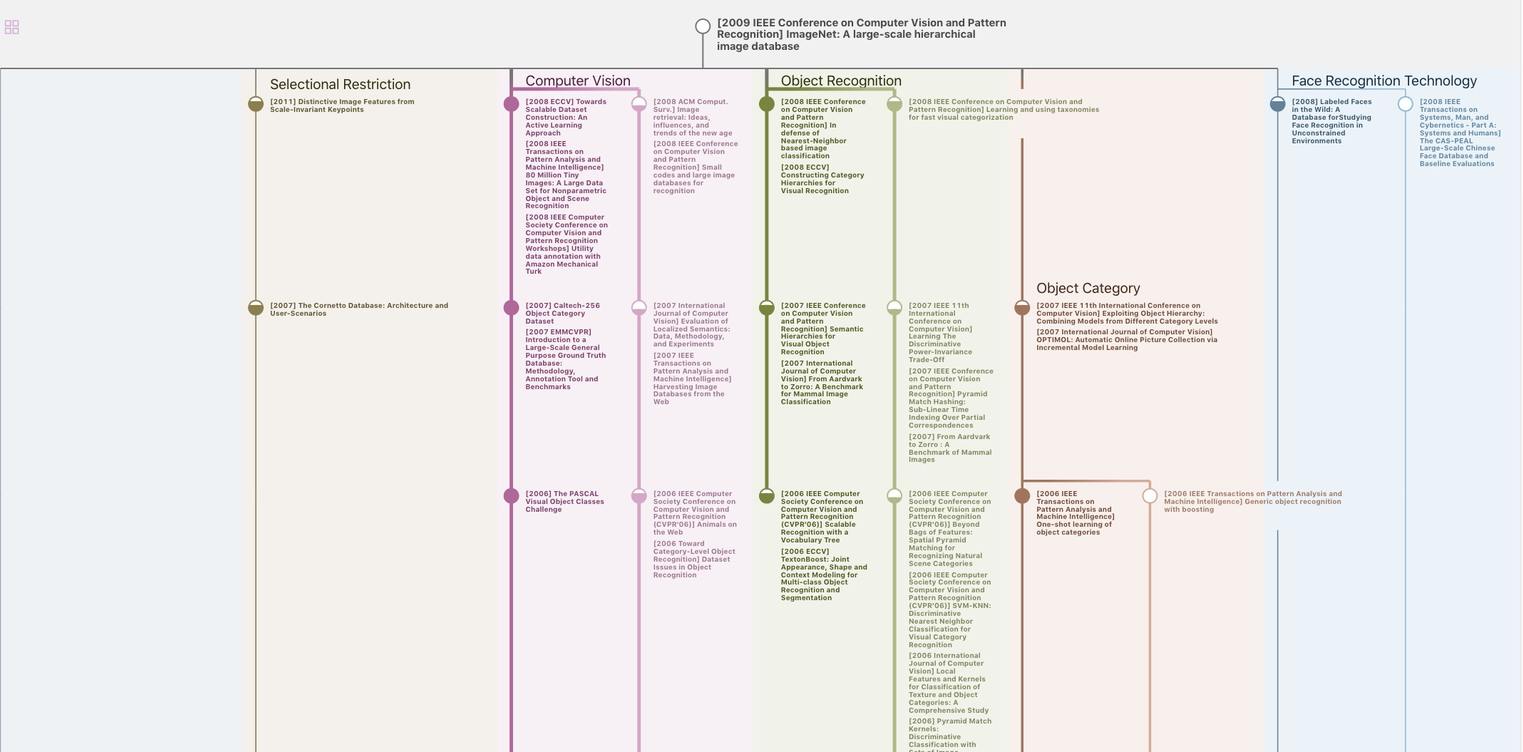
生成溯源树,研究论文发展脉络
Chat Paper
正在生成论文摘要