A Study of "Symbiosis Bias" in A/B Tests of Recommendation Algorithms
arXiv (Cornell University)(2023)
摘要
One assumption underlying the unbiasedness of global treatment effect estimates from randomized experiments is the stable unit treatment value assumption (SUTVA). Many experiments that compare the efficacy of different recommendation algorithms violate SUTVA, because each algorithm is trained on a pool of shared data, often coming from a mixture of recommendation algorithms in the experiment. We explore, through simulation, cluster randomized and data-diverted solutions to mitigating this bias, which we call "symbiosis bias."
更多查看译文
关键词
symbiosis bias,tests,algorithms
AI 理解论文
溯源树
样例
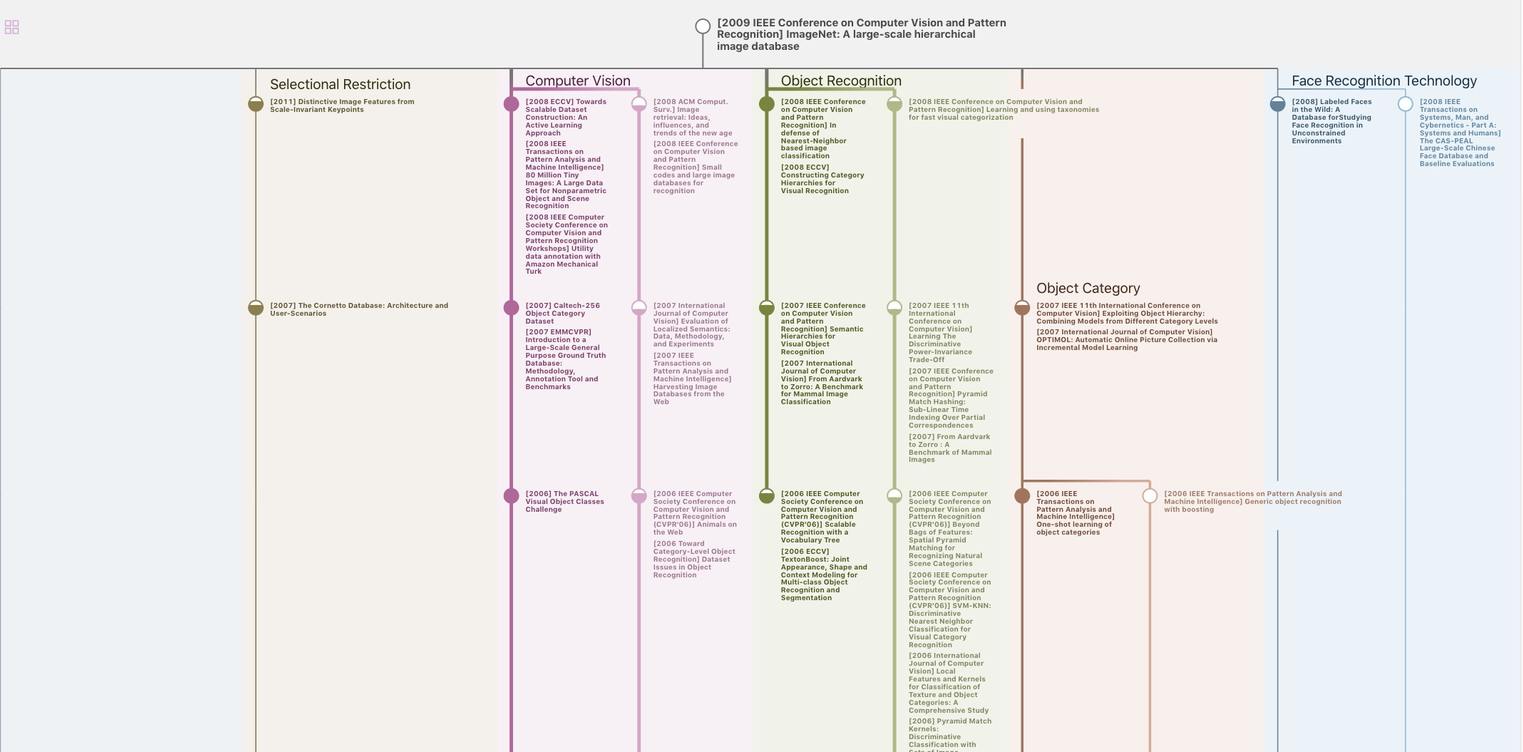
生成溯源树,研究论文发展脉络
Chat Paper
正在生成论文摘要