CONVERSER: Few-Shot Conversational Dense Retrieval with Synthetic Data Generation
CoRR(2023)
摘要
Conversational search provides a natural interface for information retrieval (IR). Recent approaches have demonstrated promising results in applying dense retrieval to conversational IR. However, training dense retrievers requires large amounts of in-domain paired data. This hinders the development of conversational dense retrievers, as abundant in-domain conversations are expensive to collect. In this paper, we propose CONVERSER, a framework for training conversational dense retrievers with at most 6 examples of in-domain dialogues. Specifically, we utilize the in-context learning capability of large language models to generate conversational queries given a passage in the retrieval corpus. Experimental results on conversational retrieval benchmarks OR-QuAC and TREC CAsT 19 show that the proposed CONVERSER achieves comparable performance to fully-supervised models, demonstrating the effectiveness of our proposed framework in few-shot conversational dense retrieval. All source code and generated datasets are available at https://github.com/MiuLab/CONVERSER
更多查看译文
关键词
retrieval,synthetic data
AI 理解论文
溯源树
样例
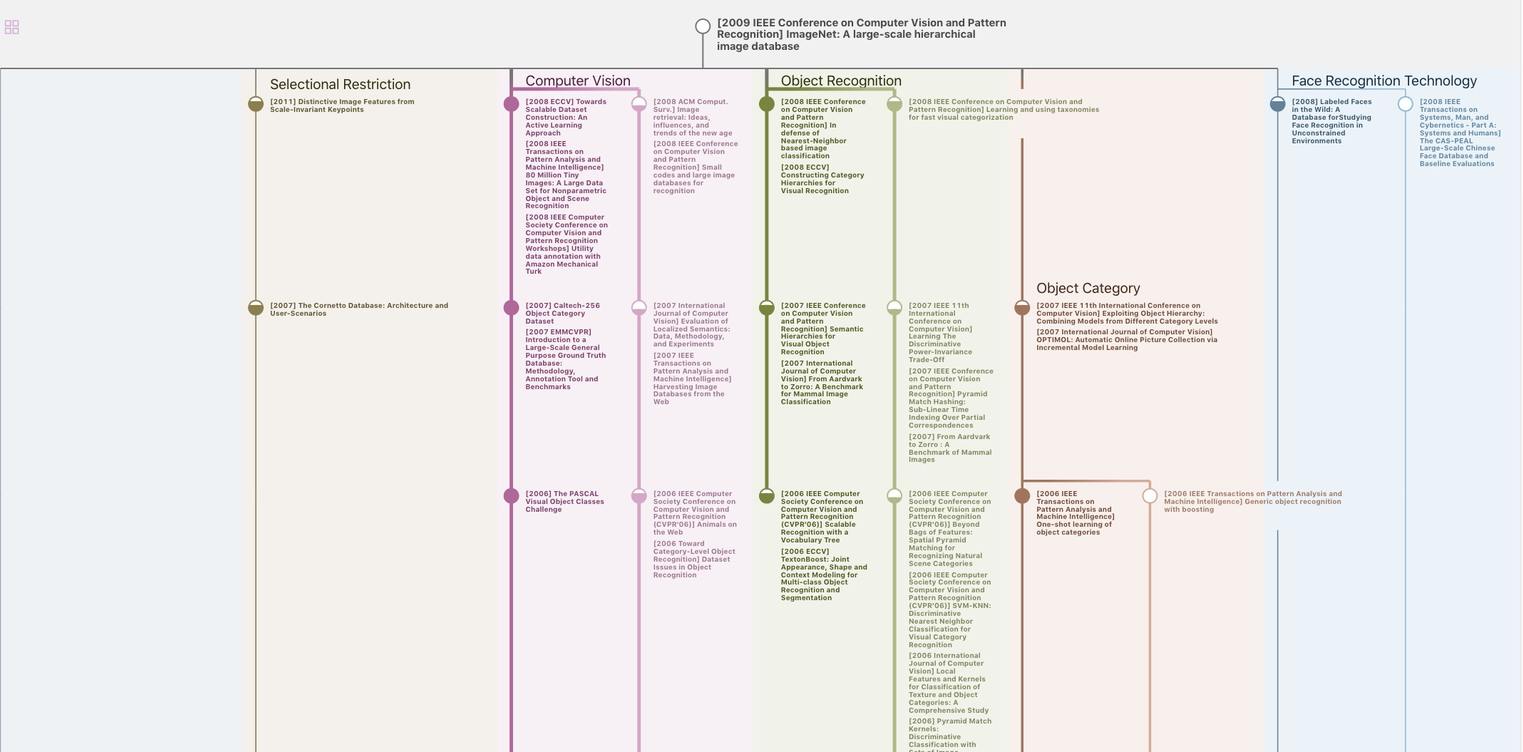
生成溯源树,研究论文发展脉络
Chat Paper
正在生成论文摘要