Leveraging Foundation models for Unsupervised Audio-Visual Segmentation
CoRR(2023)
摘要
Audio-Visual Segmentation (AVS) aims to precisely outline audible objects in a visual scene at the pixel level. Existing AVS methods require fine-grained annotations of audio-mask pairs in supervised learning fashion. This limits their scalability since it is time consuming and tedious to acquire such cross-modality pixel level labels. To overcome this obstacle, in this work we introduce unsupervised audio-visual segmentation with no need for task-specific data annotations and model training. For tackling this newly proposed problem, we formulate a novel Cross-Modality Semantic Filtering (CMSF) approach to accurately associate the underlying audio-mask pairs by leveraging the off-the-shelf multi-modal foundation models (e.g., detection [1], open-world segmentation [2] and multi-modal alignment [3]). Guiding the proposal generation by either audio or visual cues, we design two training-free variants: AT-GDINO-SAM and OWOD-BIND. Extensive experiments on the AVS-Bench dataset show that our unsupervised approach can perform well in comparison to prior art supervised counterparts across complex scenarios with multiple auditory objects. Particularly, in situations where existing supervised AVS methods struggle with overlapping foreground objects, our models still excel in accurately segmenting overlapped auditory objects. Our code will be publicly released.
更多查看译文
关键词
segmentation,foundation models,audio-visual
AI 理解论文
溯源树
样例
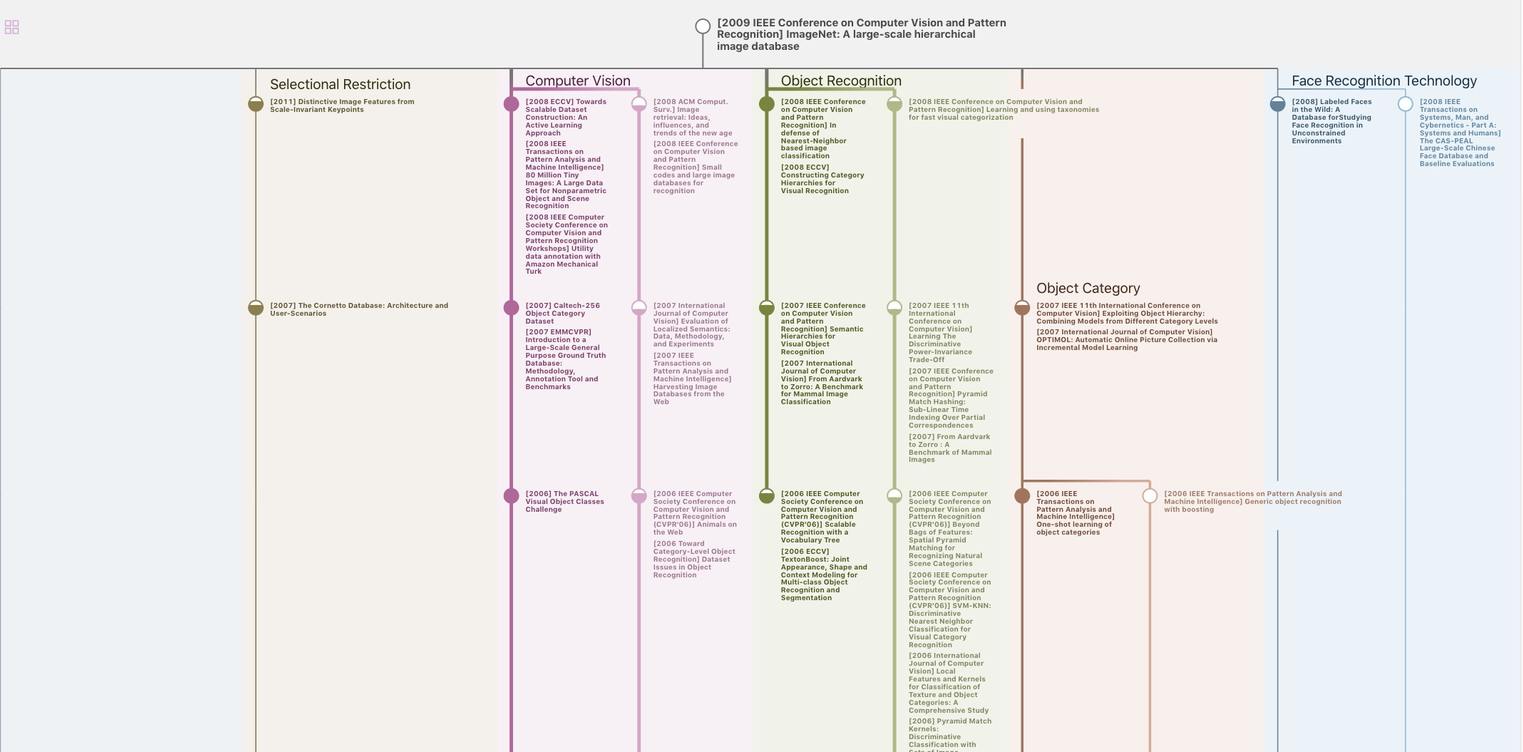
生成溯源树,研究论文发展脉络
Chat Paper
正在生成论文摘要