Zero-Shot Visual Classification with Guided Cropping
CoRR(2023)
摘要
Pretrained vision-language models, such as CLIP, show promising zero-shot performance across a wide variety of datasets. For closed-set classification tasks, however, there is an inherent limitation: CLIP image encoders are typically designed to extract generic image-level features that summarize superfluous or confounding information for the target tasks. This results in degradation of classification performance, especially when objects of interest cover small areas of input images. In this work, we propose CLIP with Guided Cropping (GC-CLIP), where we use an off-the-shelf zero-shot object detection model in a preprocessing step to increase focus of zero-shot classifier to the object of interest and minimize influence of extraneous image regions. We empirically show that our approach improves zero-shot classification results across architectures and datasets, favorably for small objects.
更多查看译文
关键词
visual,classification,zero-shot
AI 理解论文
溯源树
样例
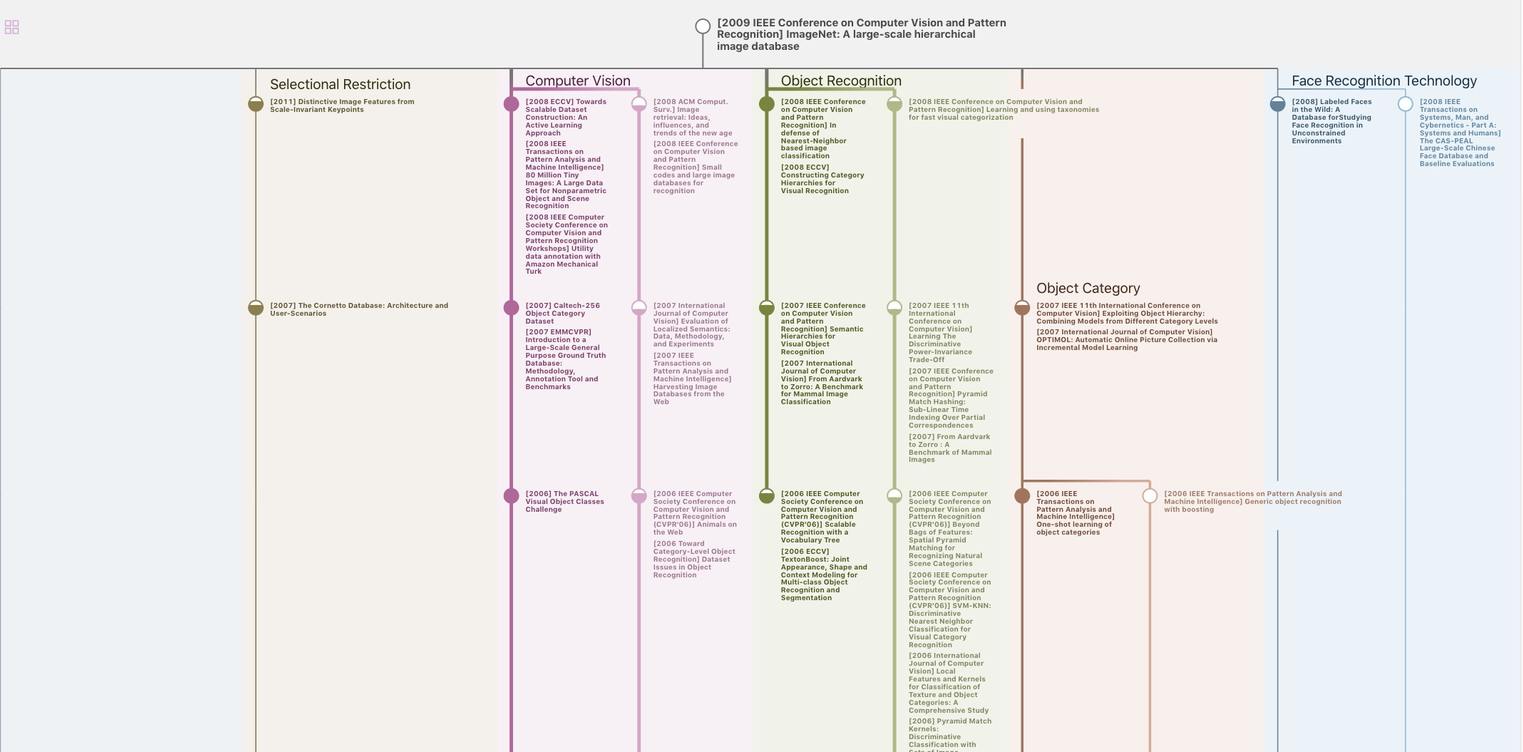
生成溯源树,研究论文发展脉络
Chat Paper
正在生成论文摘要