Leveraging Large Language Models and Weak Supervision for Social Media Data Annotation: An Evaluation Using COVID-19 Self-reported Vaccination Tweets
HCI INTERNATIONAL 2023 LATE BREAKING PAPERS, HCII 2023, PT III(2023)
摘要
The COVID-19 pandemic has presented significant challenges to the healthcare industry and society as a whole. With the rapid development of COVID-19 vaccines, social media platforms have become a popular medium for discussions on vaccine-related topics. Identifying vaccine-related tweets and analyzing them can provide valuable insights for public health researchers and policymakers. However, manual annotation of a large number of tweets is time-consuming and expensive. In this study, we evaluate the usage of Large Language Models, in this case GPT-4 (March 23 version), and weak supervision, to identify COVID-19 vaccine-related tweets, with the purpose of comparing performance against human annotators. We leveraged a manually curated gold-standard dataset and used GPT-4 to provide labels without any additional fine-tuning or instructing, in a single-shot mode (no additional prompting).
更多查看译文
关键词
Large language models,GPT,weak supervision,social media data
AI 理解论文
溯源树
样例
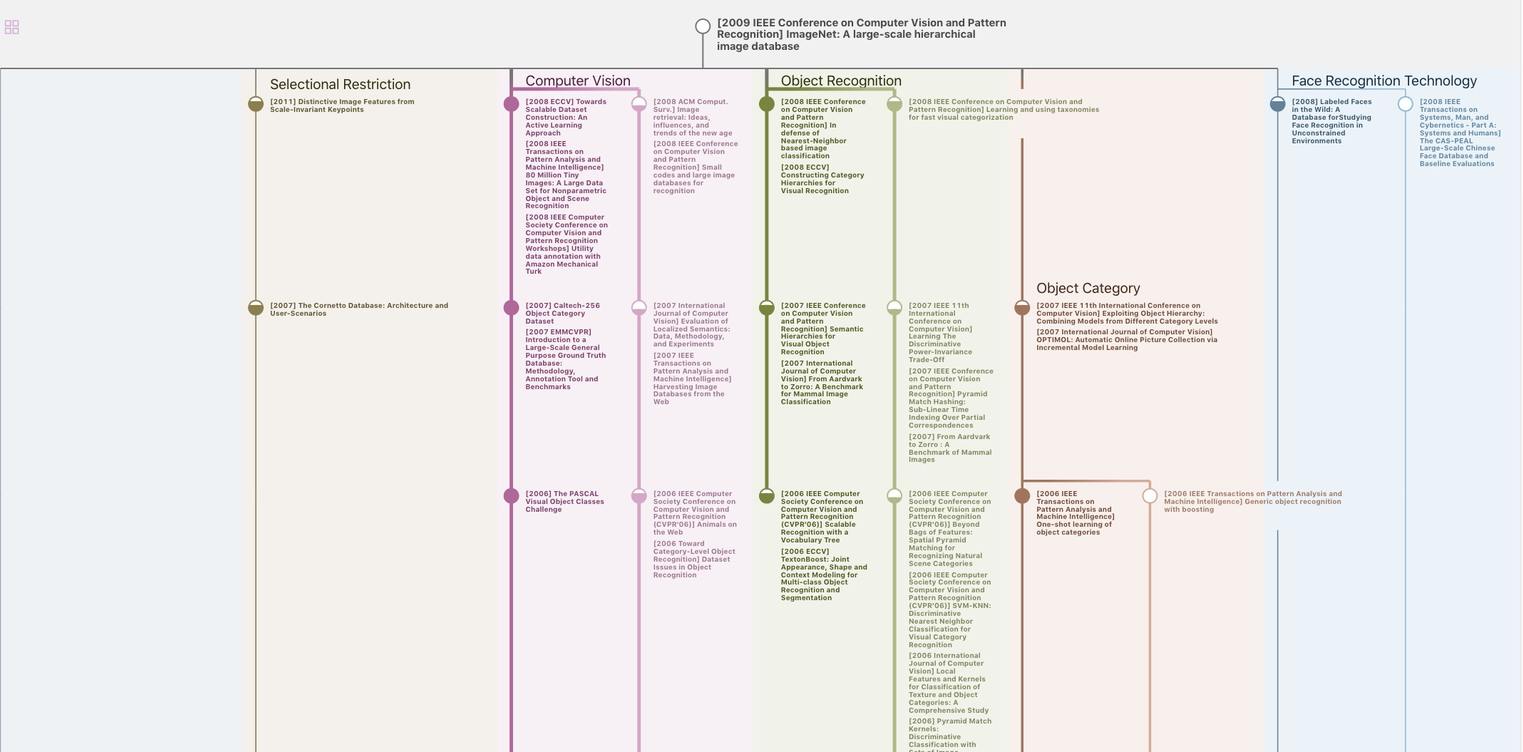
生成溯源树,研究论文发展脉络
Chat Paper
正在生成论文摘要