Coffee authentication via targeted metabolomics and machine learning: Unveiling origins and their discriminating biochemicals
FOOD BIOSCIENCE(2023)
摘要
Coffee is an export commodity that is prone to fraudulent practices. Therefore, this study presents a novel approach to authenticate coffee origins using targeted metabolomics with gas chromatography-tandem mass spectrometry (GC-MS/MS) and machine learning models. A total of 200 coffee samples from different harvest years and areas from Indonesia were extracted using the derivatisation method and then analysed for their metabolite profiles. Several supervised machine-learning models were tested to classify coffee origins and discover their potential markers. The study found various metabolite features spanning diverse chemical classes, encompassing sugar alcohols, carbohydrates, amino acids, organic acids, fatty acids, and phenols. Random forest (RF) and partial least squares discriminant analysis (PLS-DA) were among the most accurate models in predicting the origin of coffee from several classes in the validation dataset. The accuracy of both models is in the range of 91%-100%. Furthermore, this study proposes a new strategy for determining "intersection features" as the set of features that are important and common to both RF and PLS-DA models, thereby providing a robust selection of coffee origin markers. Overall, the approach and findings of this study have far-reaching implications for coffee authentication.
更多查看译文
关键词
Coffee,Authentication,Metabolomics,GC-MS/MS,Machine learning,Random forest,PLS-DA
AI 理解论文
溯源树
样例
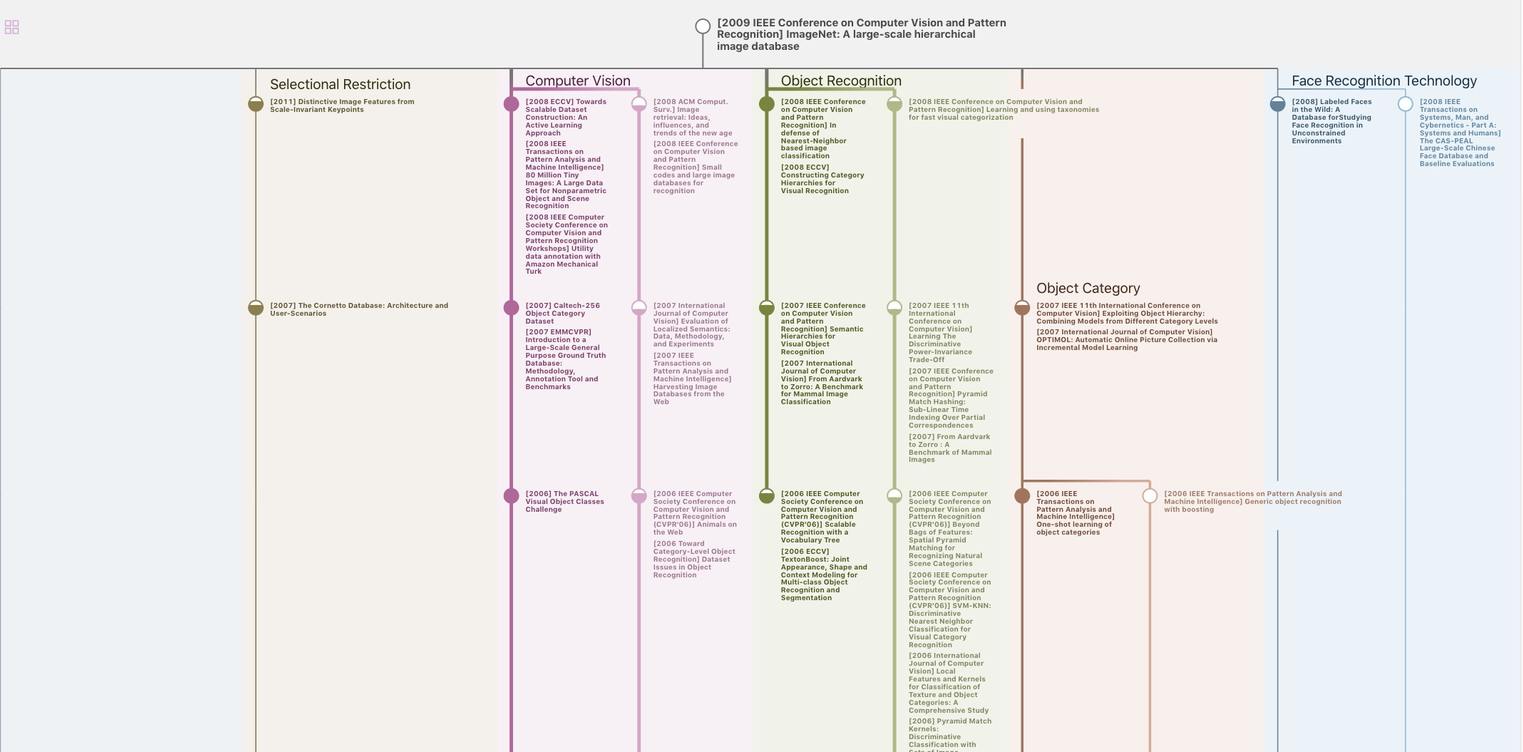
生成溯源树,研究论文发展脉络
Chat Paper
正在生成论文摘要