Quadratic Prediction of Time Series via Auto-Cumulants
SANKHYA-SERIES A-MATHEMATICAL STATISTICS AND PROBABILITY(2024)
摘要
Nonlinear prediction of time series can offer potential accuracy gains over linear methods when the process is nonlinear. As there are numerous examples of nonlinearity in time series data (e.g., finance, macroeconomics, image, and speech processing), there seems to be merit in developing a general theory and methodology. We explore the class of quadratic predictors, which directly generalize linear predictors, and show that they can be computed in terms of the second, third, and fourth auto-cumulant functions when the time series is stationary. The new formulas for quadratic predictors generalize the normal equations for linear prediction of stationary time series, and hence we obtain quadratic generalizations of the Yule-Walker equations; we explicitly quantify the prediction gains in quadratic over linear methods. We say a stochastic process is second order forecastable if quadratic prediction provides an advantage over linear prediction. One of the key results of the paper provides a characterization of second order forecastable processes in terms of the spectral and bi-spectral densities. We verify these conditions for some popular nonlinear time series models.
更多查看译文
关键词
Bi-spectral density,Nonlinear prediction,Nonlinear processes,Quadratic prediction,Polyspectra
AI 理解论文
溯源树
样例
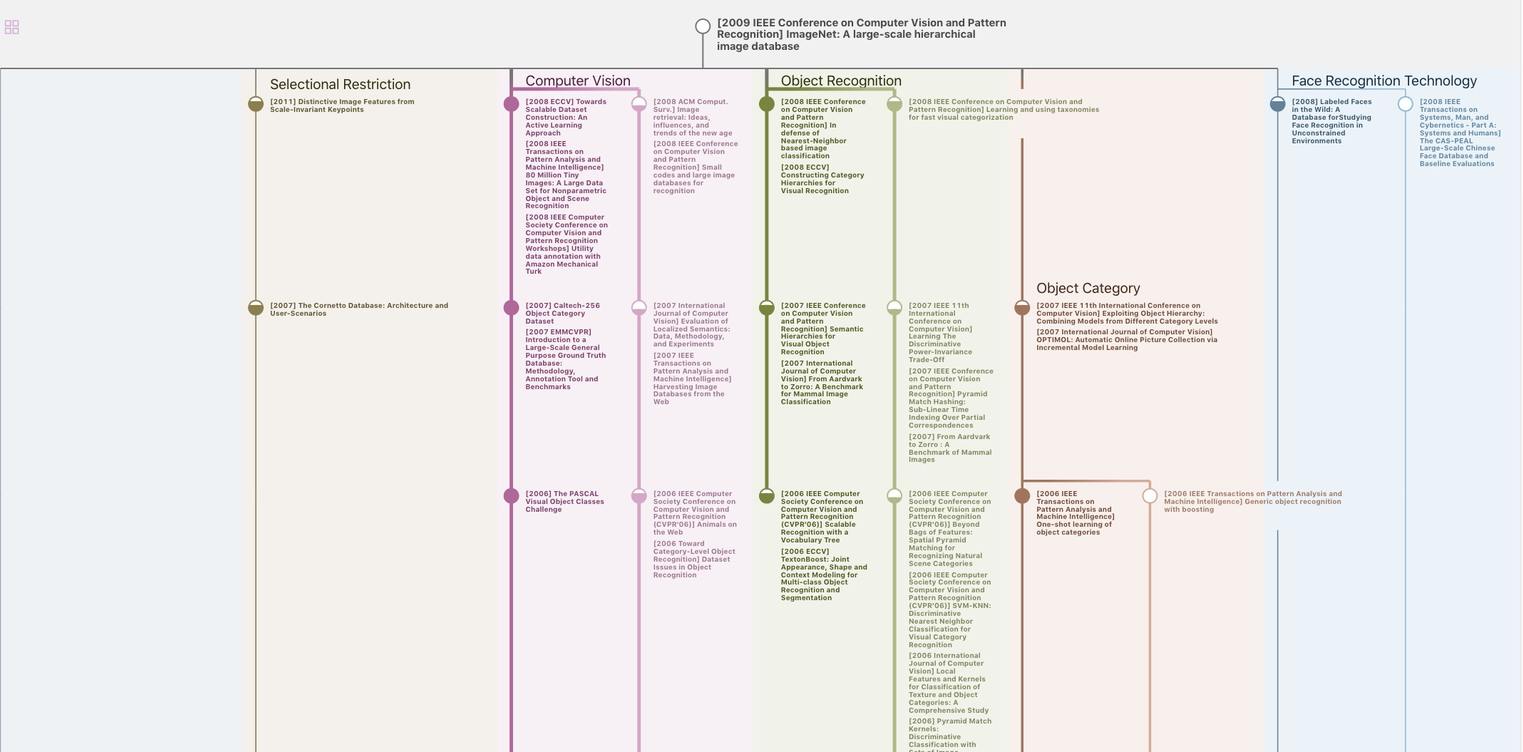
生成溯源树,研究论文发展脉络
Chat Paper
正在生成论文摘要