Single image super-resolution approaches in medical images based-deep learning: a survey
MULTIMEDIA TOOLS AND APPLICATIONS(2023)
摘要
Medical image Super-Resolution (SR) reconstruction refers to the process of regenerating a High-Resolution (HR) image from a degraded Low-Resolution (LR) image or images. Due to the breakthrough in Deep Learning (DL) for medical computer vision applications, researchers are attempting to introduce deep neural networks and tackle the challenge of image SR reconstruction by establishing an end-to-end deep-level network. Recently, the medical field has gained the attention of researchers, especially the field of computer vision. An intuitive grasp of the fundamental concepts underlying neural network applications in medical imaging and a working knowledge of technical jargon will significantly assist the reader in comprehending current and future applications. This paper aims to provide a complete overview of current improvements in Single Image Super-Resolution (SISR) in the medical field through the use of DL techniques. We can generally classify extant research on SR techniques into four major groups: traditional SR, LR network SR, HR network SR, and perceptual quality SR. Additionally, we discuss several other critical topics, including publicly available benchmark medical datasets, performance evaluation metrics, and the implementation of the famous SR models. Finally, we end this survey by identifying several potential paths and open issues that the community should address in the future.
更多查看译文
关键词
Medical images,Deep learning,Image super-resolution,SISR,GAN
AI 理解论文
溯源树
样例
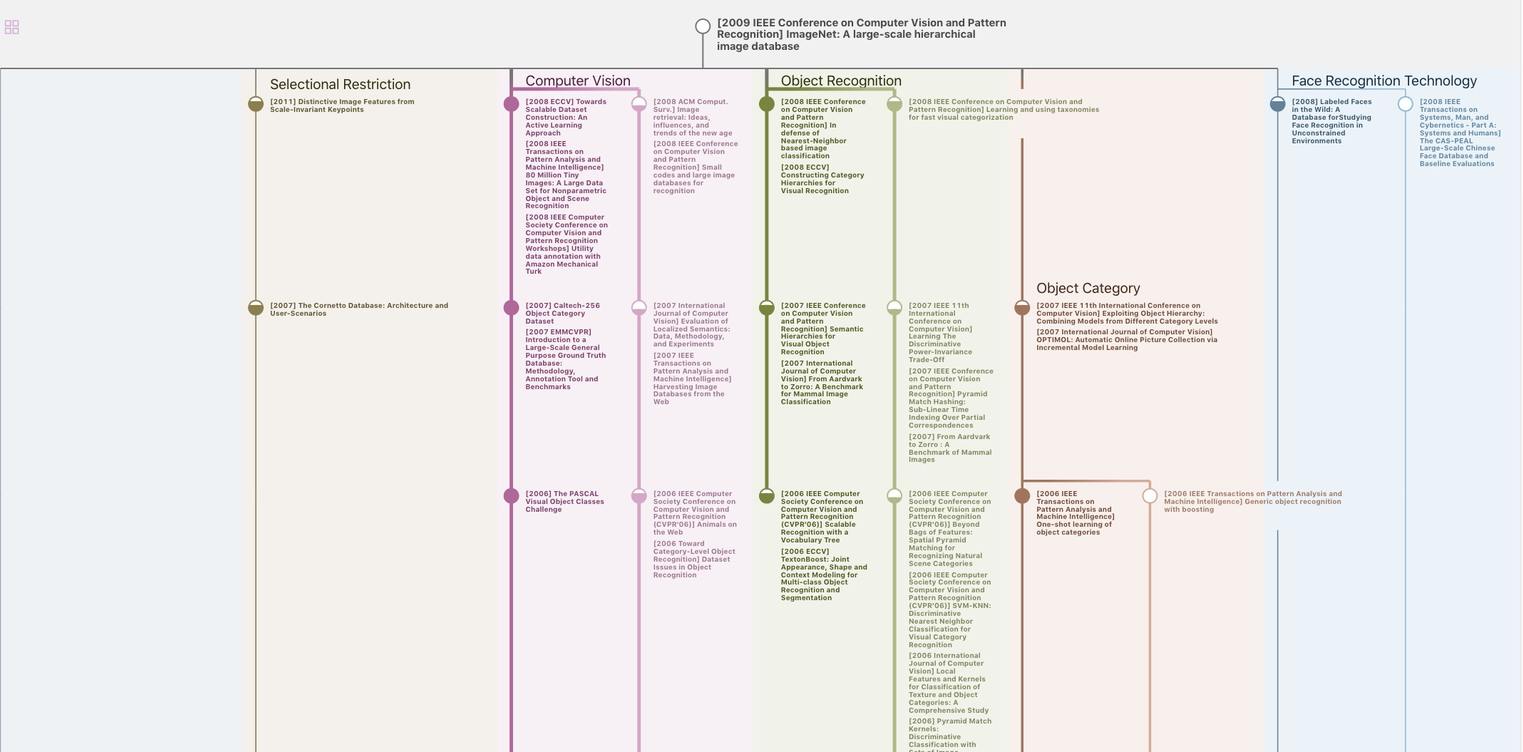
生成溯源树,研究论文发展脉络
Chat Paper
正在生成论文摘要