The impact of (simulated) resolution on breast cancer diagnosis based on high-resolution 3D micro-CT microcalcification images
MEDICAL PHYSICS(2024)
摘要
BackgroundBreast microcalcifications (MCs) are considered to be a robust marker of breast cancer. A machine learning model can provide breast cancer diagnosis based on properties of individual MCs - if their characteristics are captured at high resolution and in 3D.PurposeThe main purpose of the study was to explore the impact of image resolution (8 & mu;m, 16 & mu;m, 32 & mu;m, 64 & mu;m) when diagnosing breast cancer using radiomics features extracted from individual high resolution 3D micro-CT MC images.MethodsBreast MCs extracted from 86 female patients were analyzed at four different spatial resolutions: 8 & mu;m (original resolution) and 16 & mu;m, 32 & mu;m, 64 & mu;m (simulated image resolutions). Radiomic features were extracted at each image resolution in an attempt, to find a compact feature signature allowing to distinguish benign and malignant MCs. Machine learning algorithms were used for classifying individual MCs and samples (i.e., patients). For sample diagnosis, a custom-based thresholding approach was used to combine individual MC results into sample results. We conducted classification experiments when using (a) the same MCs visible in 8 & mu;m, 16 & mu;m, 32 & mu;m, and 64 & mu;m resolution; (b) the same MCs visible in 8 & mu;m, 16 & mu;m, and 32 & mu;m resolution; (c) the same MCs visible in 8 & mu;m and 16 & mu;m resolution; (d) all MCs visible in 8 & mu;m, 16 & mu;m, 32 & mu;m, and 64 & mu;m resolution. Accuracy, sensitivity, specificity, AUC, and F1 score were computed for each experiment.ResultsThe individual MC results yielded an accuracy of 77.27%, AUC of 83.83%, F1 score of 77.25%, sensitivity of 80.86%, and specificity of 72.2% at 8 & mu;m resolution. For the individual MC classifications we report for the F1 scores: a 2.29% drop when using 16 & mu;m instead of 8 & mu;m, a 4.01% drop when using 32 & mu;m instead of 8 & mu;m, a 10.69% drop when using 64 & mu;m instead of 8 & mu;m. The sample results yielded an accuracy and F1 score of 81.4%, sensitivity of 80.43%, and specificity value of 82.5% at 8 & mu;m. For the sample classifications we report for F1 score values: a 6.3% drop when using 16 & mu;m instead of 8 & mu;m, a 4.91% drop when using 32 & mu;m instead of 8 & mu;m, and a 6.3% drop when using 64 & mu;m instead of 8 & mu;m.ConclusionsThe highest classification results are obtained at the highest resolution (8 & mu;m). If breast MCs characteristics could be visualized/captured in 3D at a higher resolution compared to what is used nowadays in digital mammograms (approximately 70 & mu;m), breast cancer diagnosis will be improved.
更多查看译文
关键词
breast cancer,breast microcalcification,computer-aided detection and diagnosis systems,machine learning,micro-CT,radiomics,spatial resolution
AI 理解论文
溯源树
样例
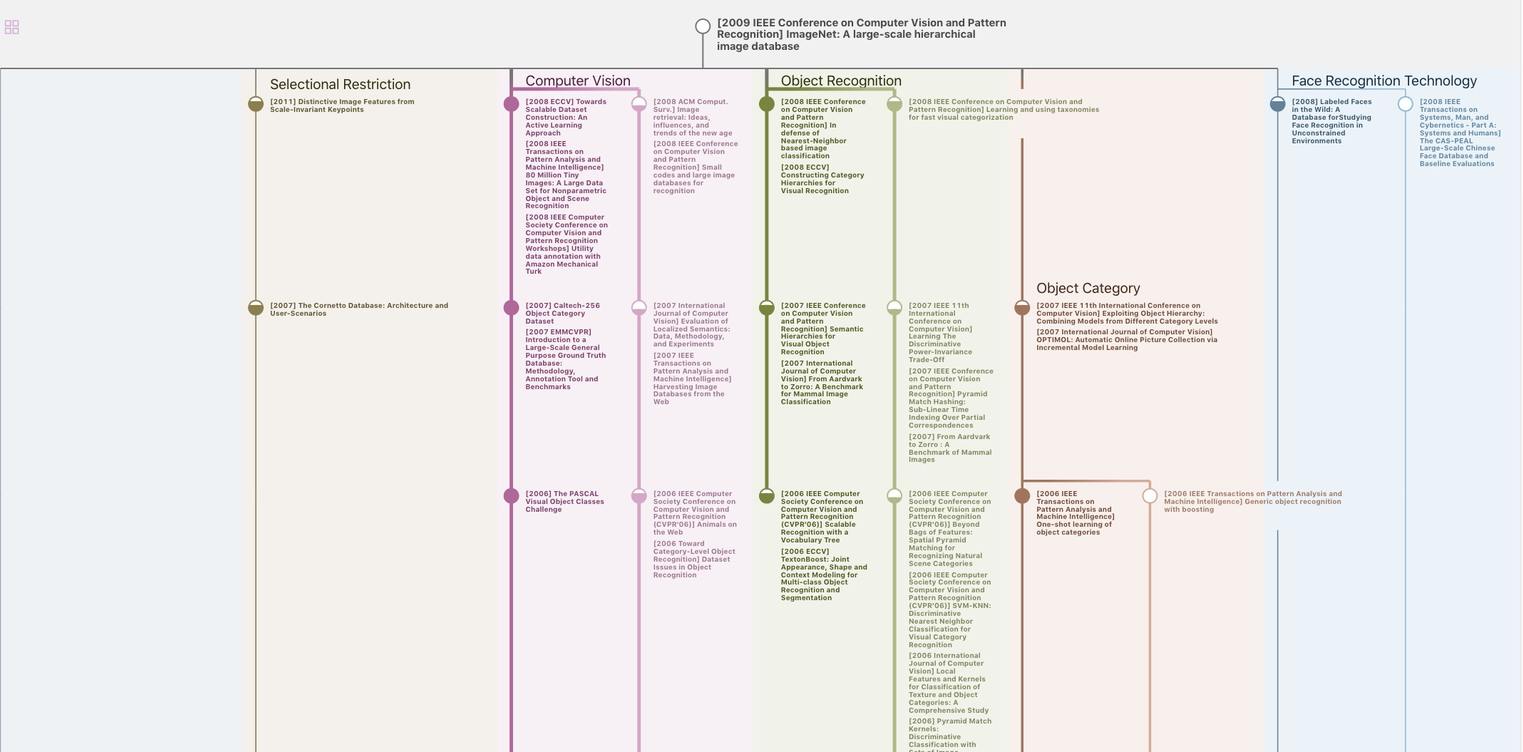
生成溯源树,研究论文发展脉络
Chat Paper
正在生成论文摘要