A fusion framework of deep learning and machine learning for predicting sgRNA cleavage efficiency.
Computers in biology and medicine(2023)
摘要
CRISPR/Cas9 system is a powerful tool for genome editing. Numerous studies have shown that sgRNAs can strongly affect the efficiency of editing. However, it is still not clear what rules should be followed for designing sgRNA with high cleavage efficiency. At present, several machine learning or deep learning methods have been developed to predict the cleavage efficiency of sgRNAs, however, the prediction accuracy of these tools is still not satisfactory. Here we propose a fusion framework of deep learning and machine learning, which first deals with the primary sequence and secondary structure features of the sgRNAs using both convolutional neural network (CNN) and recurrent neural network (RNN), and then uses the features extracted by the deep neural network to train a conventional machine learning model with LGBM. As a result, the new approach overwhelmed previous methods. The Spearman's correlation coefficient between predicted and measured sgRNA cleavage efficiency of our model (0.917) is improved by over 5% compared with the most advanced method (0.865), and the mean square error reduces from 7.89 × 10 to 4.75 × 10. Finally, we developed an online tool, CRISep (http://www.cuilab.cn/CRISep), to evaluate the availability of sgRNAs based on our models.
更多查看译文
关键词
CRISPR/Cas9,sgRNA,Machine learning,Efficiency prediction
AI 理解论文
溯源树
样例
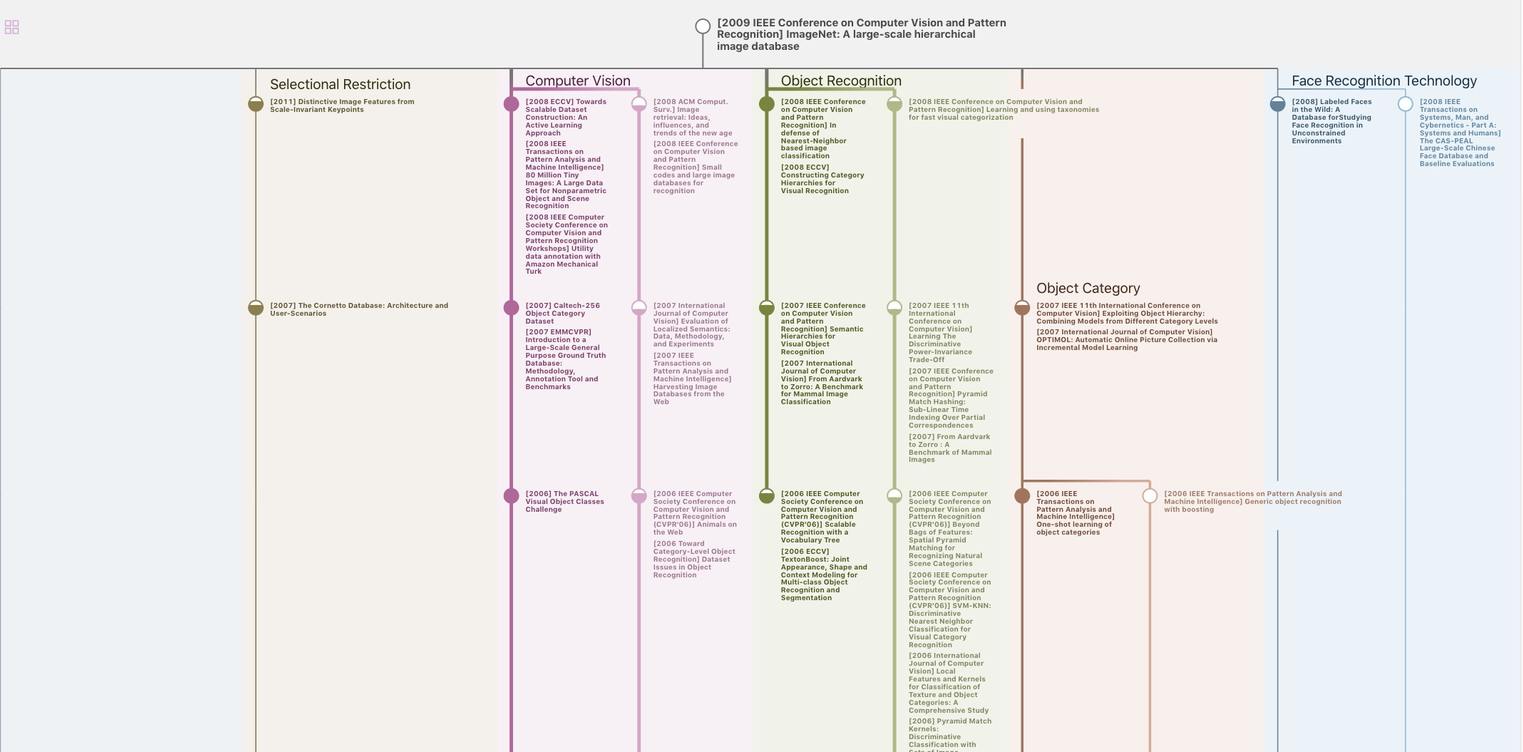
生成溯源树,研究论文发展脉络
Chat Paper
正在生成论文摘要