Novel Wearable HD-EMG Sensor With Shift-Robust Gesture Recognition Using Deep Learning
IEEE TRANSACTIONS ON BIOMEDICAL CIRCUITS AND SYSTEMS(2023)
摘要
In this work, we present a hardware-software solution to improve the robustness of hand gesture recognition to confounding factors in myoelectric control. The solution includes a novel, full-circumference, flexible, 64-channel high-density electromyography (HD-EMG) sensor called EMaGer. The stretchable, wearable sensor adapts to different forearm sizes while maintaining uniform electrode density around the limb. Leveraging this uniformity, we propose novel array barrel-shifting data augmentation (ABSDA) approach used with a convolutional neural network (CNN), and an anti-aliased CNN (AA-CNN), that provides shift invariance around the limb for improved classification robustness to electrode movement, forearm orientation, and inter-session variability. Signals are sampled from a 4x16 HD-EMG array of electrodes at a frequency of 1 kHz and 16-bit resolution. Using data from 12 non-amputated participants, the approach is tested in response to sensor rotation, forearm rotation, and inter-session scenarios. The proposed ABSDA-CNN method improves inter-session accuracy by 25.67% on average across users for 6 gesture classes compared to conventional CNN classification. A comparison with other devices shows that this benefit is enabled by the unique design of the EMaGer array. The AA-CNN yields improvements of up to 63.05% accuracy over non-augmented methods when tested with electrode displacements ranging from -45(degrees) to +45(degrees) around the limb. Overall, this article demonstrates the benefits of co-designing sensor systems, processing methods, and inference algorithms to leverage synergistic and interdependent properties to solve state-of-the-art problems.
更多查看译文
关键词
Electromyography,Electrodes,Convolutional neural networks,Robustness,Muscles,Gesture recognition,Deep learning,Artificial intelligence (AI),biomedical,data augmentation,deep learning,flexible PCB,hand gesture recognition (HGR),electromyography (EMG),HD-EMG,prosthesis control
AI 理解论文
溯源树
样例
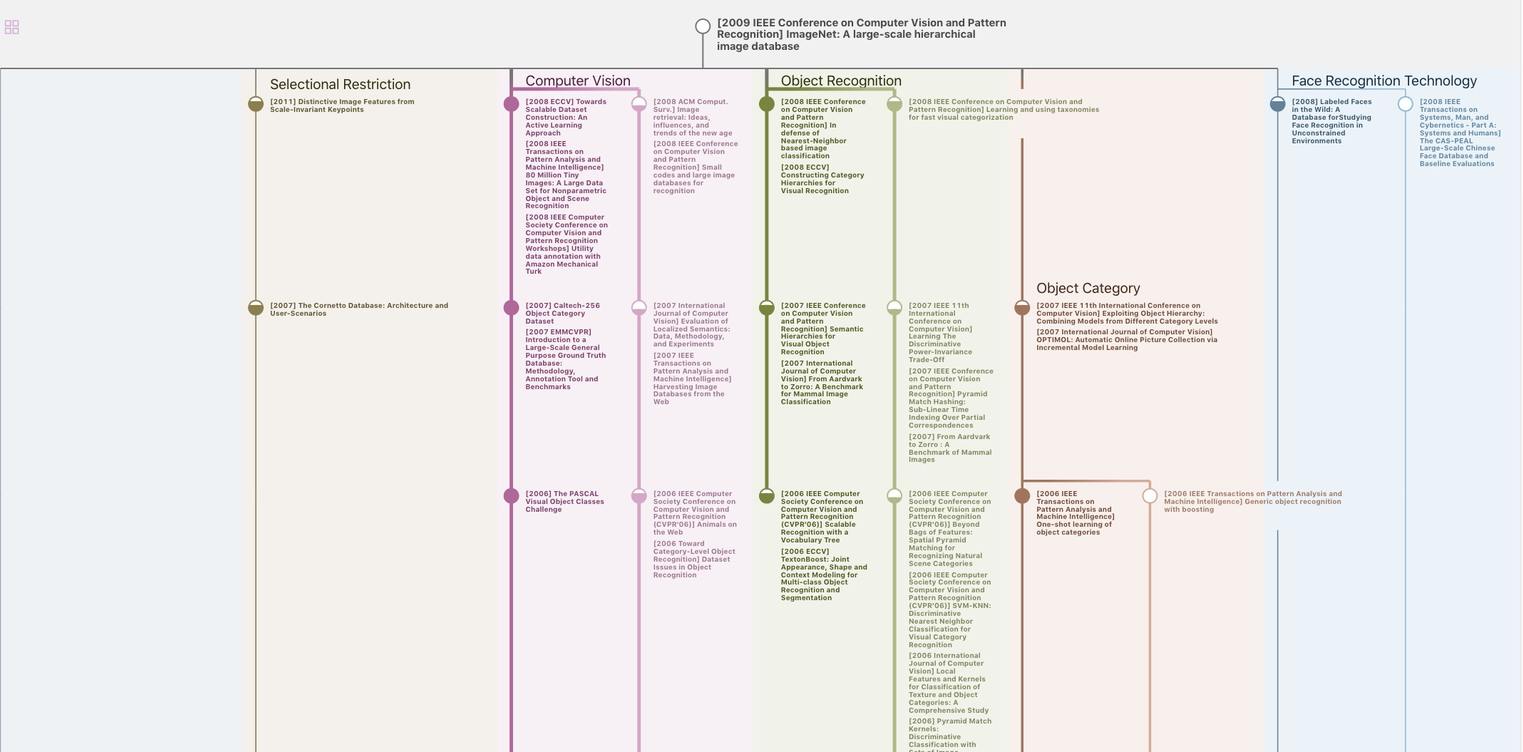
生成溯源树,研究论文发展脉络
Chat Paper
正在生成论文摘要