YOLO-G: Improved YOLO for cross-domain object detection.
PloS one(2023)
摘要
Cross-domain object detection is a key problem in the research of intelligent detection models. Different from lots of improved algorithms based on two-stage detection models, we try another way. A simple and efficient one-stage model is introduced in this paper, comprehensively considering the inference efficiency and detection precision, and expanding the scope of undertaking cross-domain object detection problems. We name this gradient reverse layer-based model YOLO-G, which greatly improves the object detection precision in cross-domain scenarios. Specifically, we add a feature alignment branch following the backbone, where the gradient reverse layer and a classifier are attached. With only a small increase in computational, the performance is higher enhanced. Experiments such as Cityscapes→Foggy Cityscapes, SIM10k→Cityscape, PASCAL VOC→Clipart, and so on, indicate that compared with most state-of-the-art (SOTA) algorithms, the proposed model achieves much better mean Average Precision (mAP). Furthermore, ablation experiments were also performed on 4 components to confirm the reliability of the model. The project is available at https://github.com/airy975924806/yolo-G.
更多查看译文
关键词
improved yolo-g,detection,object,cross-domain
AI 理解论文
溯源树
样例
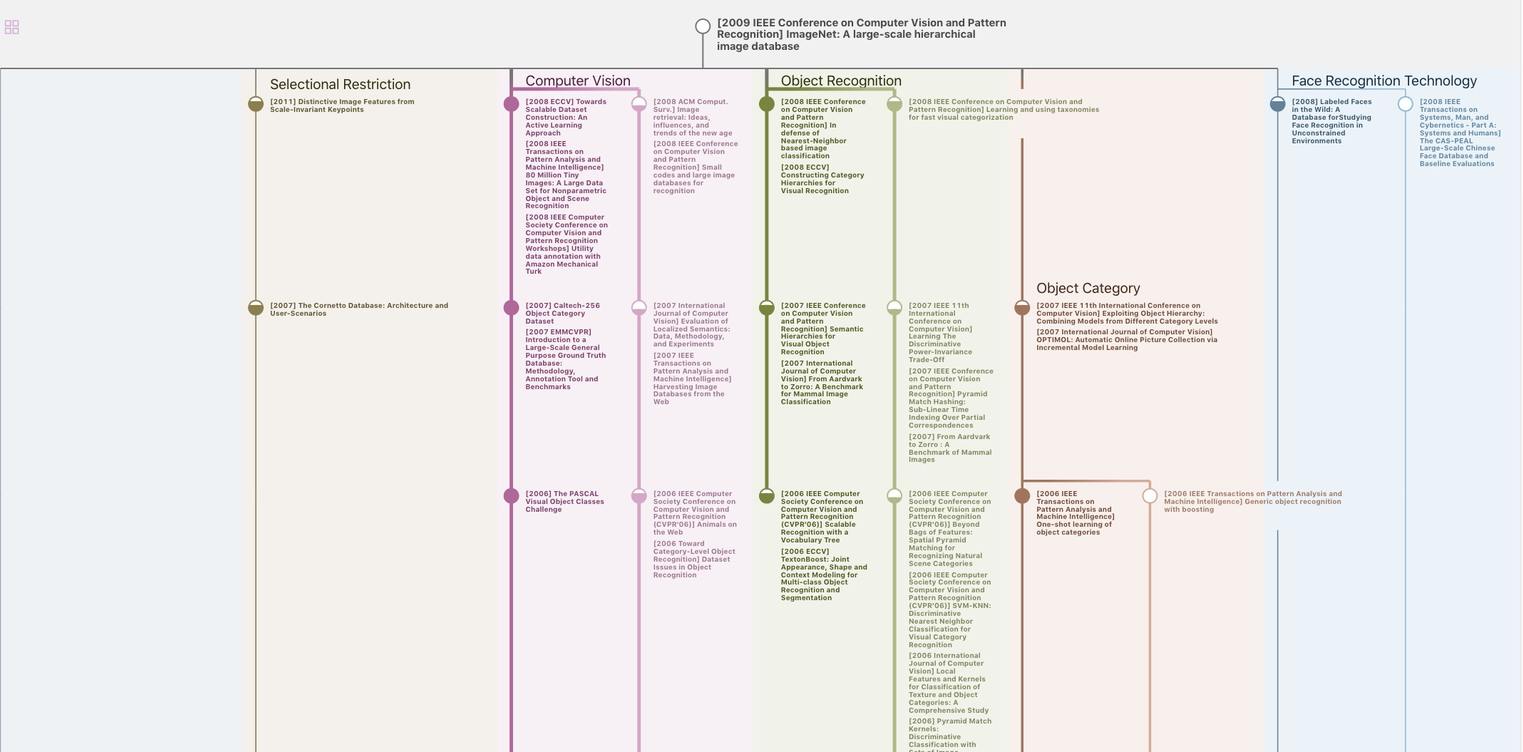
生成溯源树,研究论文发展脉络
Chat Paper
正在生成论文摘要