Self-training and multi-task learning for limited data: evaluation study on object detection
2023 IEEE/CVF INTERNATIONAL CONFERENCE ON COMPUTER VISION WORKSHOPS, ICCVW(2023)
摘要
Self-training allows a network to learn from the predictions of a more complicated model, thus often requires well-trained teacher models and mixture of teacher-student data while multi-task learning jointly optimizes different targets to learn salient interrelationship and requires multi-task annotations for each training example. These frameworks, despite being particularly data demanding have potentials for data exploitation if such assumptions can be relaxed. In this paper, we compare self-training object detection under the deficiency of teacher training data where students are trained on unseen examples by the teacher, and multi-task learning with partially annotated data, i.e. single-task annotation per training example. Both scenarios have their own limitation but potentially helpful with limited annotated data. Experimental results show the improvement of performance when using a weak teacher with unseen data for training a multi-task student. Despite the limited setup we believe the experimental results show the potential of multi-task knowledge distillation and self-training, which could be beneficial for future study. Source code and data splits are at https://lhoangan.github.io/multas
更多查看译文
关键词
object detection,learning,self-training,multi-task
AI 理解论文
溯源树
样例
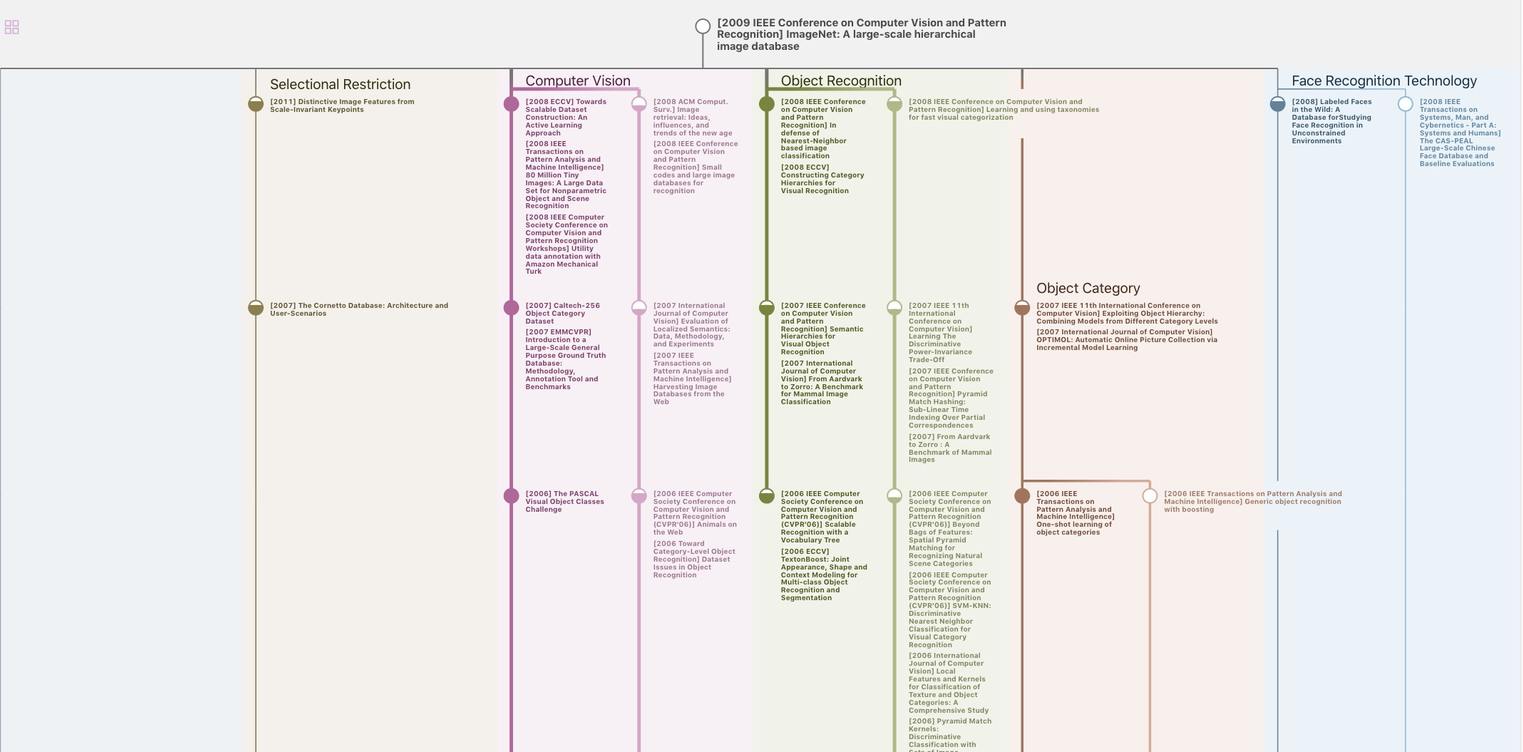
生成溯源树,研究论文发展脉络
Chat Paper
正在生成论文摘要