HAMUR: Hyper Adapter for Multi-Domain Recommendation
PROCEEDINGS OF THE 32ND ACM INTERNATIONAL CONFERENCE ON INFORMATION AND KNOWLEDGE MANAGEMENT, CIKM 2023(2023)
摘要
Multi-Domain Recommendation (MDR) has gained significant attention in recent years, which leverages data from multiple domains to enhance their performance concurrently. However, current MDR models are confronted with two limitations. Firstly, the majority of these models adopt an approach that explicitly shares parameters between domains, leading to mutual interference among them. Secondly, due to the distribution differences among domains, the utilization of static parameters in existing methods limits their flexibility to adapt to diverse domains. To address these challenges, we propose a novel model Hyper Adapter for MUlti-Domain Recommendation (HAMUR). Specifically, HAMUR consists of two components: (1). Domain-specific adapter, designed as a pluggable module that can be seamlessly integrated into various existing multidomain backbone models, and (2). Domain-shared hyper-network, which implicitly captures shared information among domains and dynamically generates the parameters for the adapter. We conduct extensive experiments on two public datasets using various backbone networks. The experimental results validate the effectiveness and scalability of the proposed model. And we release our code implementation publicly (12).
更多查看译文
关键词
Multi-Domain Recommendation,Recommendation Systems,CTR Prediction
AI 理解论文
溯源树
样例
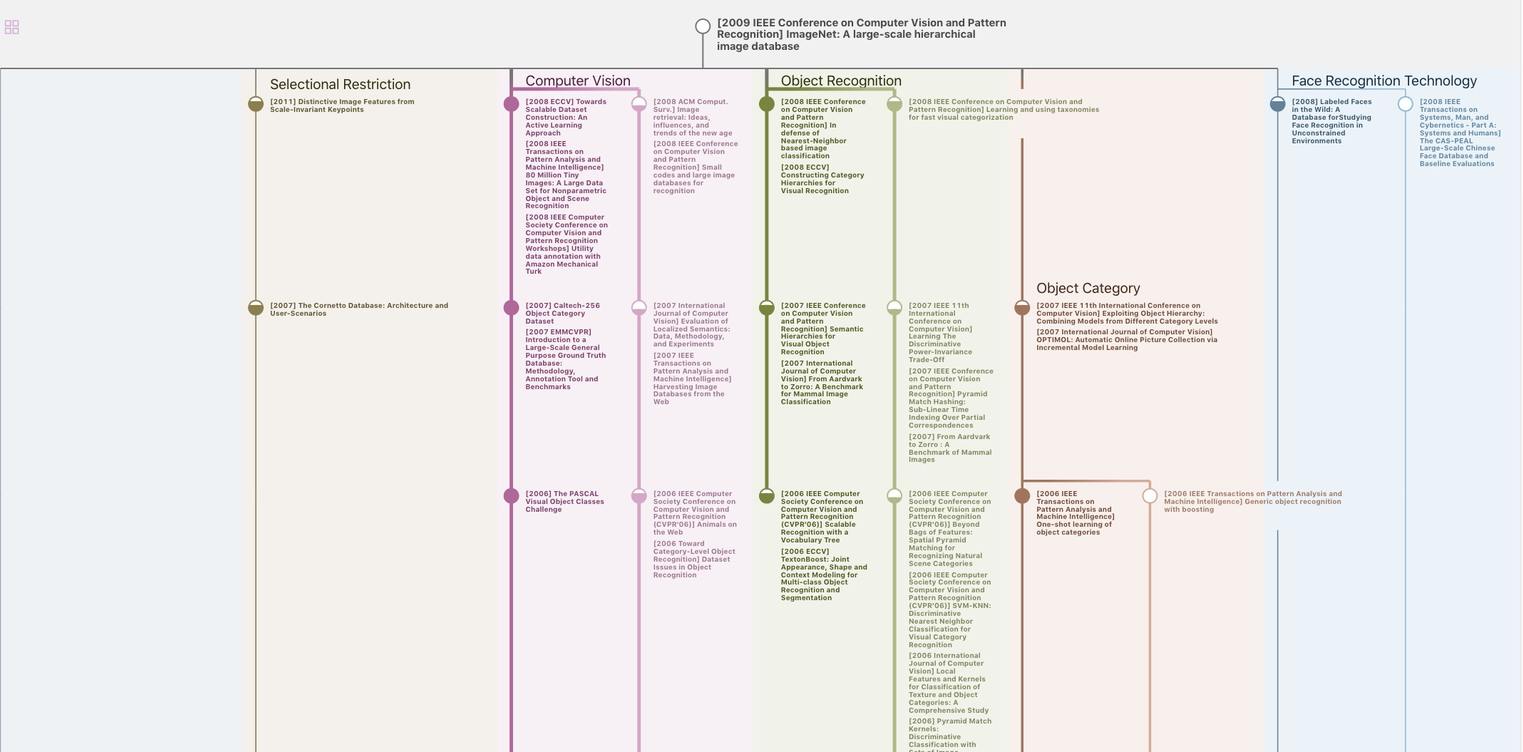
生成溯源树,研究论文发展脉络
Chat Paper
正在生成论文摘要