Private Distribution Testing with Heterogeneous Constraints: Your Epsilon Might Not Be Mine
CoRR(2023)
摘要
Private closeness testing asks to decide whether the underlying probability distributions of two sensitive datasets are identical or differ significantly in statistical distance, while guaranteeing (differential) privacy of the data. As in most (if not all) distribution testing questions studied under privacy constraints, however, previous work assumes that the two datasets are equally sensitive, i.e., must be provided the same privacy guarantees. This is often an unrealistic assumption, as different sources of data come with different privacy requirements; as a result, known closeness testing algorithms might be unnecessarily conservative, ``paying'' too high a privacy budget for half of the data. In this work, we initiate the study of the closeness testing problem under heterogeneous privacy constraints, where the two datasets come with distinct privacy requirements.
更多查看译文
AI 理解论文
溯源树
样例
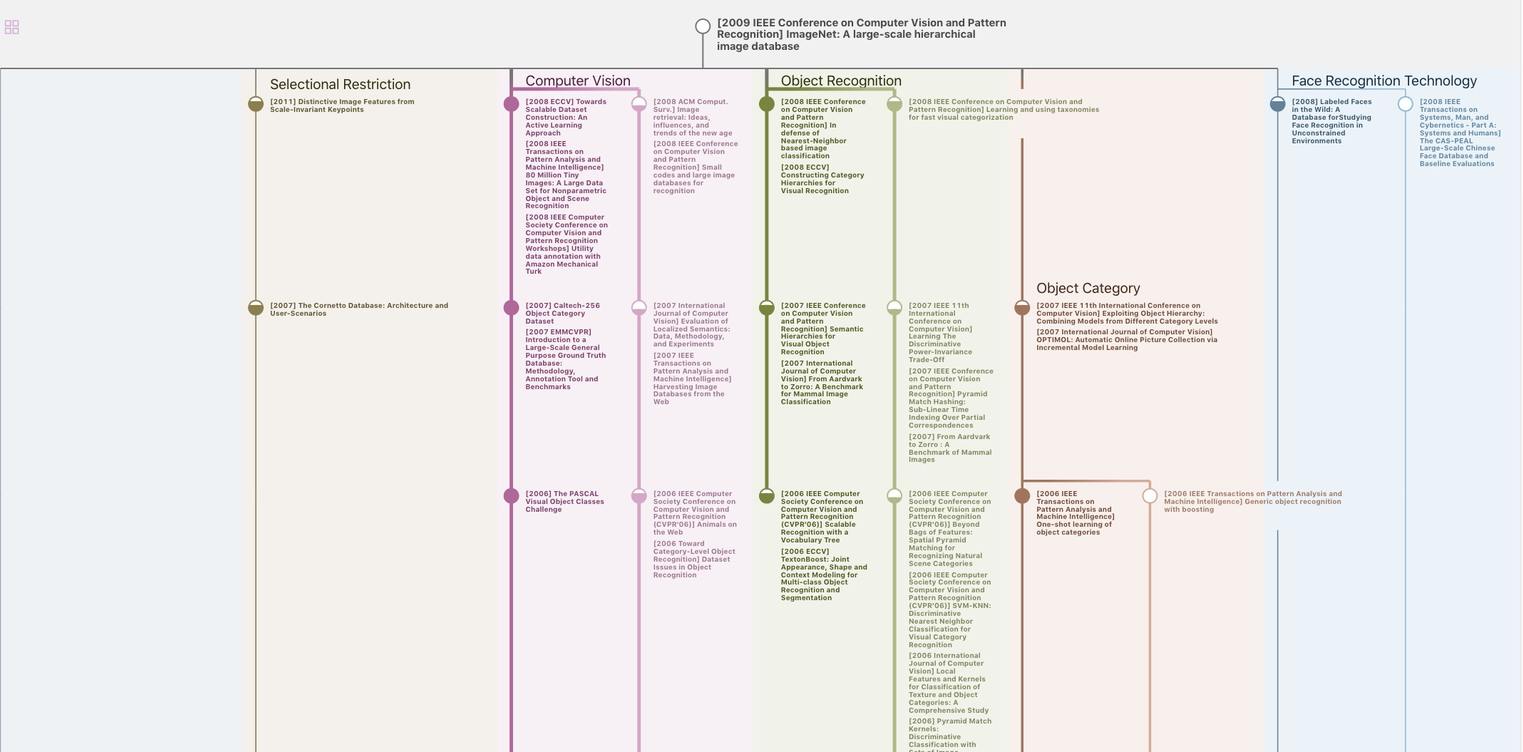
生成溯源树,研究论文发展脉络
Chat Paper
正在生成论文摘要