EANet: Expert Attention Network for Online Trajectory Prediction
CoRR(2023)
摘要
Trajectory prediction plays a crucial role in autonomous driving. Existing mainstream research and continuoual learning-based methods all require training on complete datasets, leading to poor prediction accuracy when sudden changes in scenarios occur and failing to promptly respond and update the model. Whether these methods can make a prediction in real-time and use data instances to update the model immediately(i.e., online learning settings) remains a question. The problem of gradient explosion or vanishing caused by data instance streams also needs to be addressed. Inspired by Hedge Propagation algorithm, we propose Expert Attention Network, a complete online learning framework for trajectory prediction. We introduce expert attention, which adjusts the weights of different depths of network layers, avoiding the model updated slowly due to gradient problem and enabling fast learning of new scenario's knowledge to restore prediction accuracy. Furthermore, we propose a short-term motion trend kernel function which is sensitive to scenario change, allowing the model to respond quickly. To the best of our knowledge, this work is the first attempt to address the online learning problem in trajectory prediction. The experimental results indicate that traditional methods suffer from gradient problems and that our method can quickly reduce prediction errors and reach the state-of-the-art prediction accuracy.
更多查看译文
关键词
online trajectory prediction,expert attention network,eanet
AI 理解论文
溯源树
样例
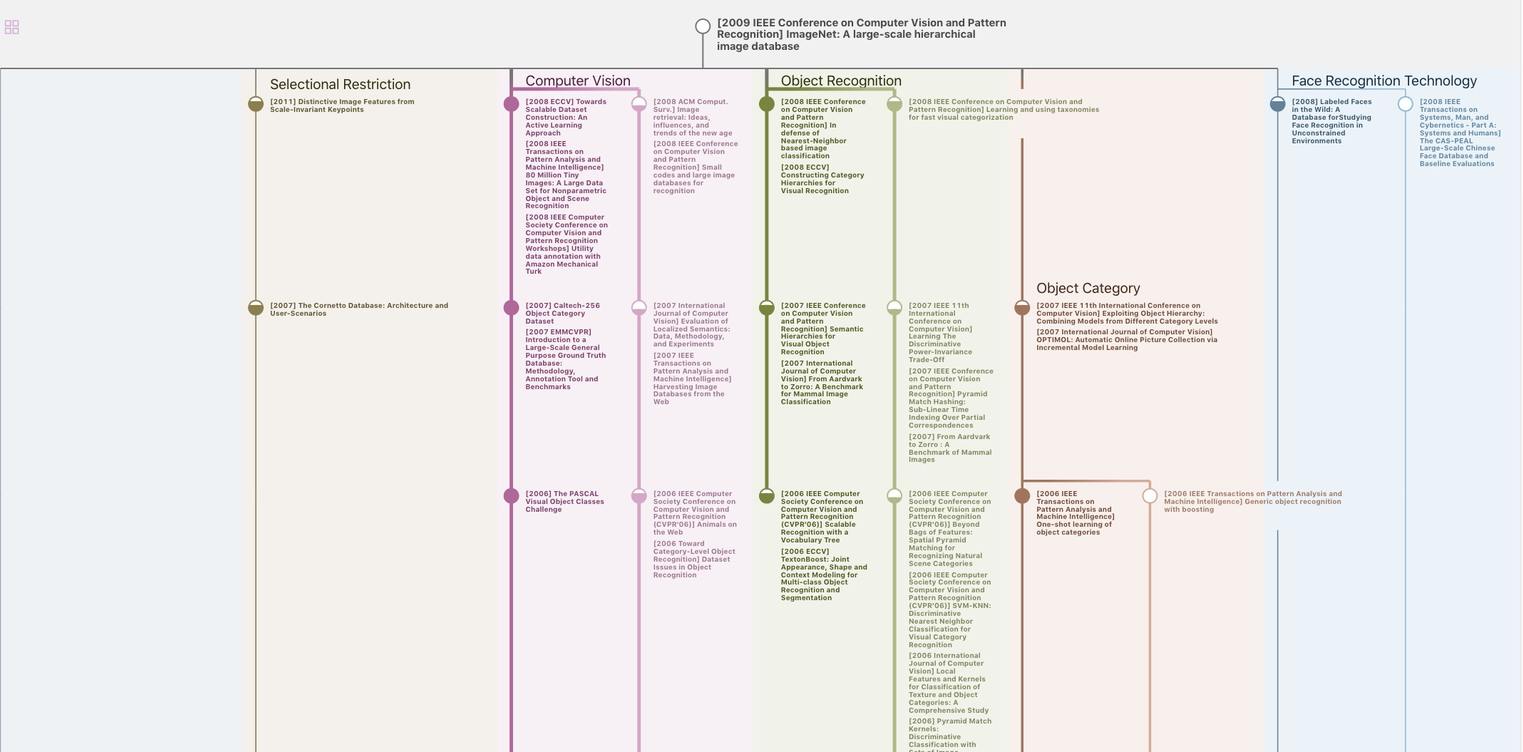
生成溯源树,研究论文发展脉络
Chat Paper
正在生成论文摘要