SHAPE: A Sample-Adaptive Hierarchical Prediction Network for Medication Recommendation
IEEE JOURNAL OF BIOMEDICAL AND HEALTH INFORMATICS(2023)
摘要
Effectively medication recommendation with complex multimorbidity conditions is a critical yet challenging task in healthcare. Most existing works predicted medications based on longitudinal records, which assumed the encoding format of intra-visit medical events are serialized and information transmitted patterns of learning longitudinal sequence data are stable. However, the following conditions may have been ignored: 1) A more compact encoder for intra-relationship in the intra-visit medical event is urgent; 2) Strategies for learning accurate representations of the variable longitudinal sequences of patients are different. In this article, we proposed a novel Sample-adaptive Hierarchical medicAtion Prediction nEtwork, termed SHAPE, to tackle the above challenges in the medication recommendation task. Specifically, we design a compact intra-visit set encoder to encode the relationship in the medical event for obtaining visit-level representation and then develop an inter-visit longitudinal encoder to learn the patient-level longitudinal representation efficiently. To endow the model with the capability of modeling the variable visit length, we introduce a soft curriculum learning method to assign the difficulty of each sample automatically by the visit length. Extensive experiments on a benchmark dataset verify the superiority of our model compared with several state-of-the-art baselines.
更多查看译文
关键词
Medication recommendation,curriculum learning,set encoder,electronic health record (EHR) datamining
AI 理解论文
溯源树
样例
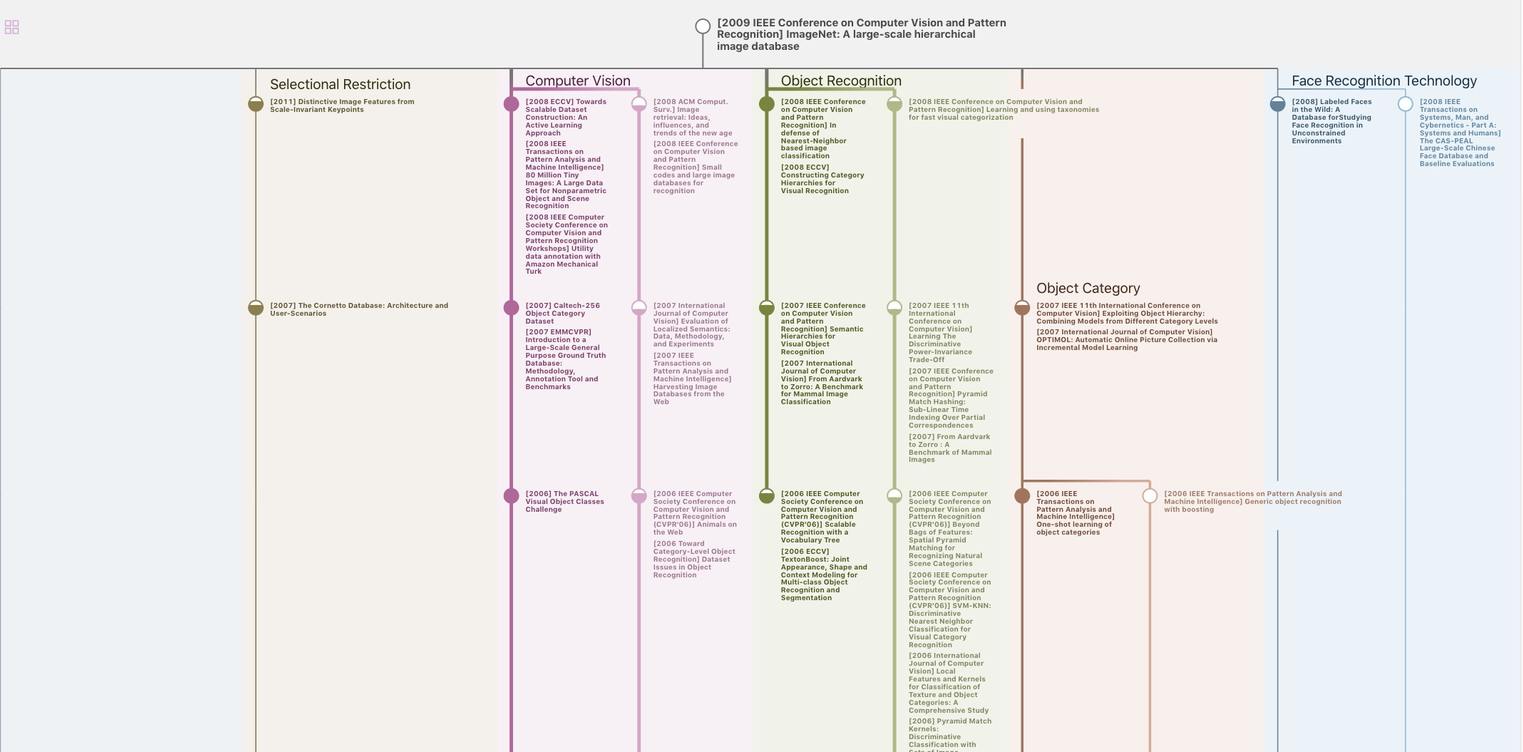
生成溯源树,研究论文发展脉络
Chat Paper
正在生成论文摘要