tSPM+; a high-performance algorithm for mining transitive sequential patterns from clinical data
CoRR(2023)
摘要
The increasing availability of large clinical datasets collected from patients can enable new avenues for computational characterization of complex diseases using different analytic algorithms. One of the promising new methods for extracting knowledge from large clinical datasets involves temporal pattern mining integrated with machine learning workflows. However, mining these temporal patterns is a computational intensive task and has memory repercussions. Current algorithms, such as the temporal sequence pattern mining (tSPM) algorithm, are already providing promising outcomes, but still leave room for optimization. In this paper, we present the tSPM+ algorithm, a high-performance implementation of the tSPM algorithm, which adds a new dimension by adding the duration to the temporal patterns. We show that the tSPM+ algorithm provides a speed up to factor 980 and a up to 48 fold improvement in memory consumption. Moreover, we present a docker container with an R-package, We also provide vignettes for an easy integration into already existing machine learning workflows and use the mined temporal sequences to identify Post COVID-19 patients and their symptoms according to the WHO definition.
更多查看译文
关键词
patterns,clinical,algorithm,high-performance
AI 理解论文
溯源树
样例
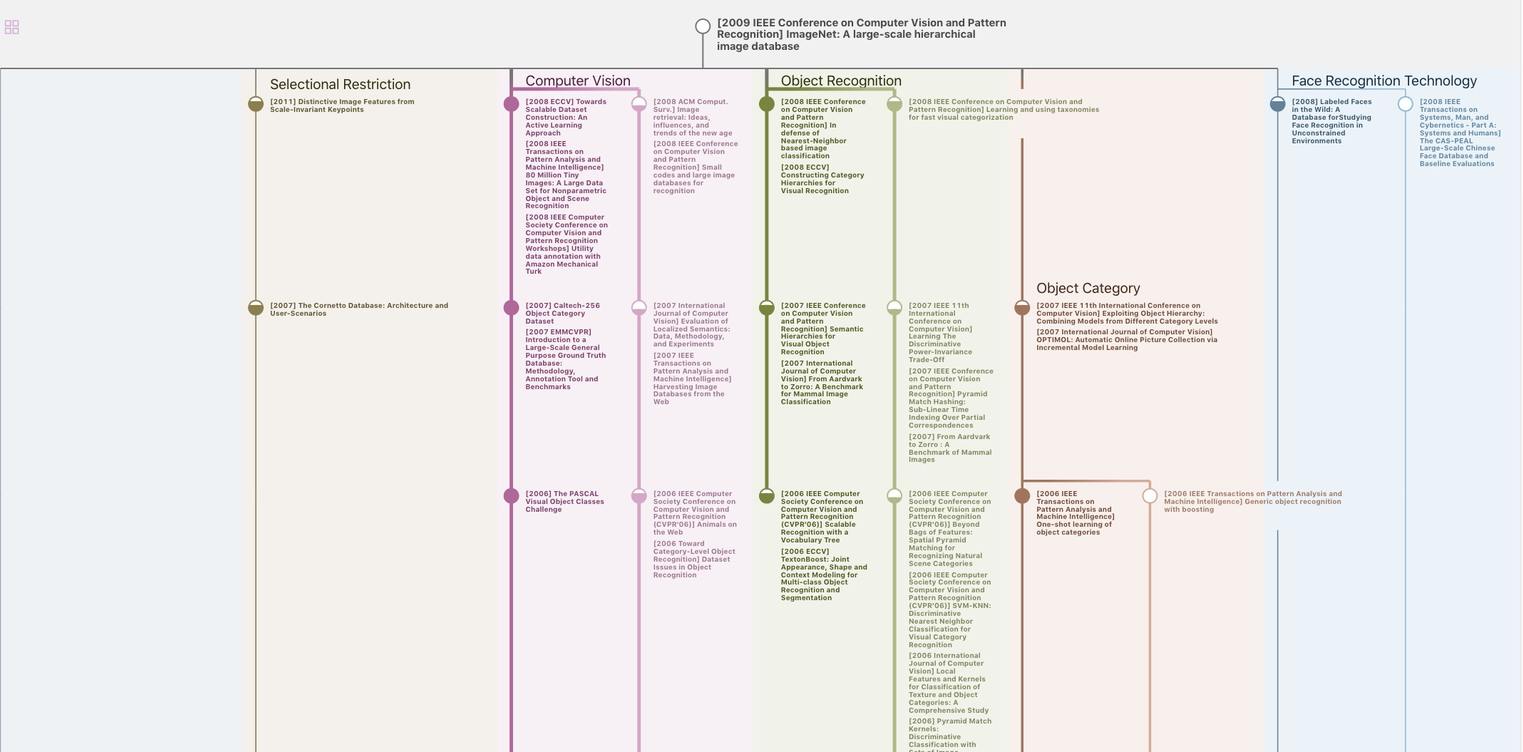
生成溯源树,研究论文发展脉络
Chat Paper
正在生成论文摘要