Rethinking long-tailed visual recognition with dynamic probability smoothing and frequency weighted focusing
2023 IEEE INTERNATIONAL CONFERENCE ON IMAGE PROCESSING, ICIP(2023)
摘要
Deep learning models trained on long-tailed (LT) datasets often exhibit bias towards head classes with high frequency. This paper highlights the limitations of existing solutions that combine class- and instance-level re-weighting loss in a naive manner. Specifically, we demonstrate that such solutions result in overfitting the training set, significantly impacting the rare classes. To address this issue, we propose a novel loss function that dynamically reduces the influence of outliers and assigns class-dependent focusing parameters. We also introduce a new long-tailed dataset, ICText-LT, featuring various image qualities and greater realism than artificially sampled datasets. Our method has proven effective, outperforming existing methods through superior quantitative results on CIFAR-LT, Tiny ImageNet-LT, and our new ICText-LT datasets. The source code and new dataset are available at https://github.com/nwjun/FFDS-Loss.
更多查看译文
关键词
Long-tailed Classification,Weighted-loss
AI 理解论文
溯源树
样例
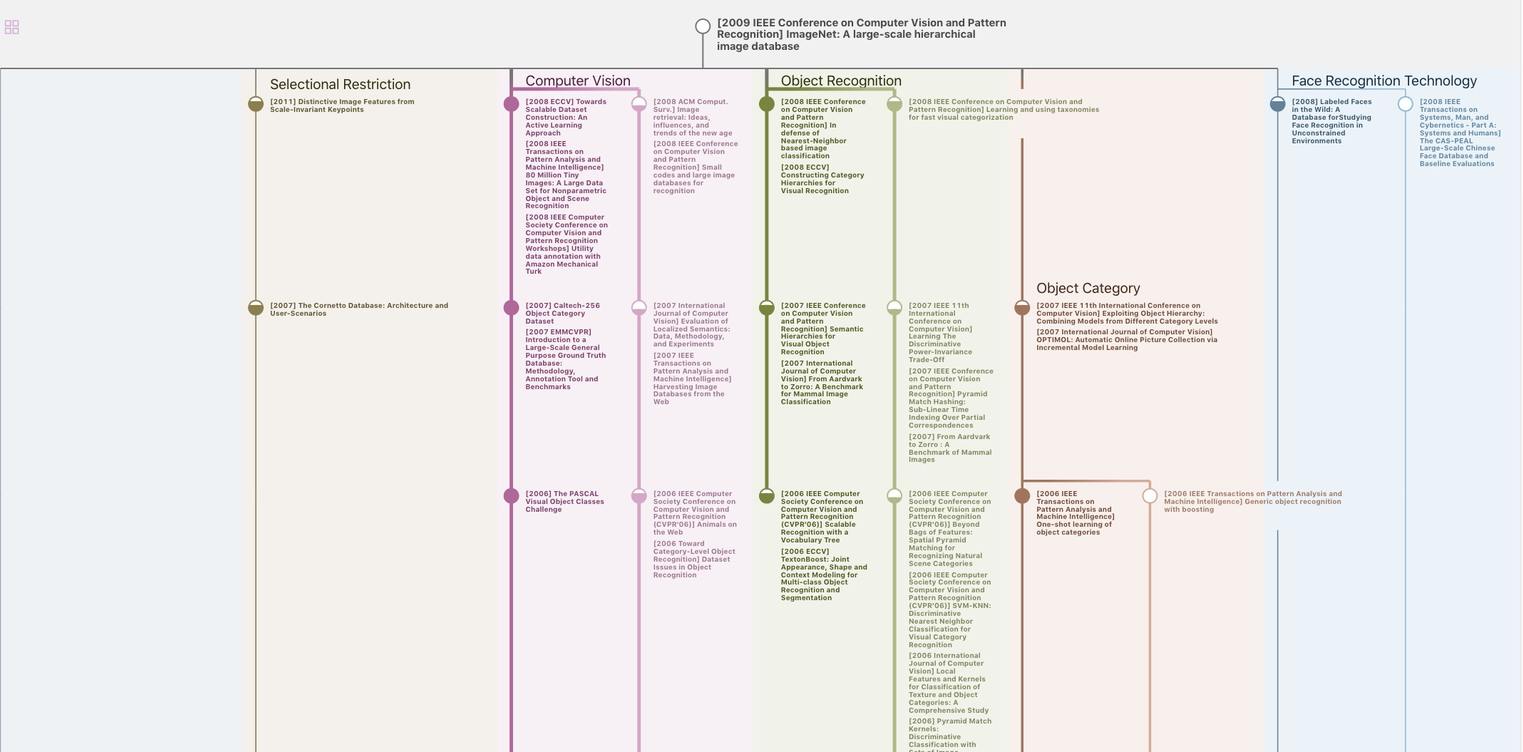
生成溯源树,研究论文发展脉络
Chat Paper
正在生成论文摘要