Unknown class feature transformation for open set domain adaptation without source data
2023 IEEE INTERNATIONAL CONFERENCE ON IMAGE PROCESSING, ICIP(2023)
摘要
Utilizing deep neural network for Domain Adaptation (DA) has made great progress on learning knowledge from source domain to solve tasks in other relevant target domains. However, conventional DA methods have several restrictions: first, the category set from the source and target domain should be identical; second, during the training process data from different domains are fed into the network simultaneously, which may not be practical in real-world applications. Consequently, we aim at tackling the Source Free Open Set Domain Adaptation scenario: source and target data cannot meet with each other and the target domain contains exclusive unknown classes. Specifically, we propose a method enhancing the unknown class identification ability by synthesizing unknown class data: a feature modifier with multiple Gated Recurrent Units (GRU) is designed to learn and modify key features from the input data in order that the modified data 'looks like' the real unknown class class data. Both real and synthesis data are used to train the classifier such that it can identify each class correctly. We evaluate our method in multiple benchmarks and the proposed framework outperforms other methods in comparison.
更多查看译文
关键词
Source Free Open Set Domain Adaptation,Feature Transformation,Unsupervised Learning
AI 理解论文
溯源树
样例
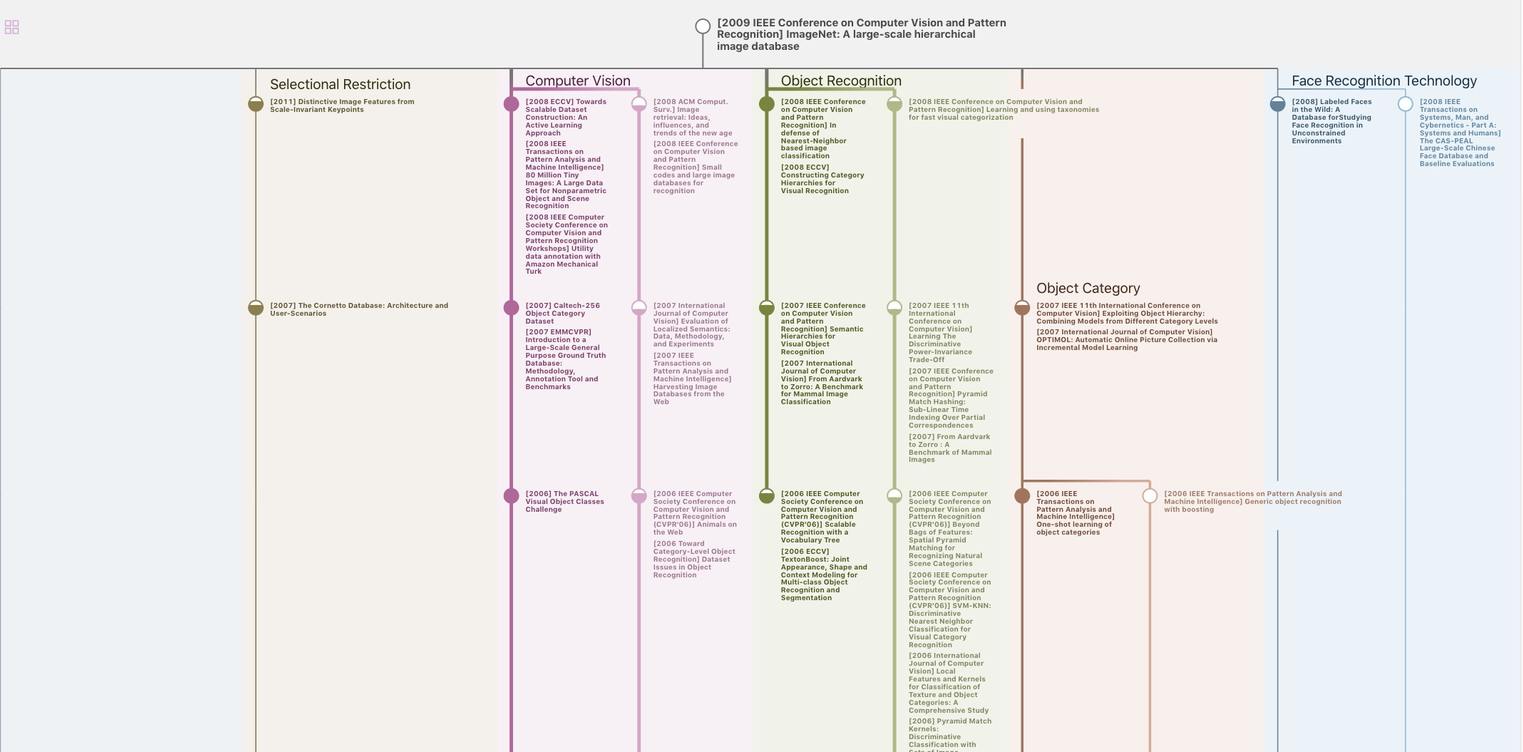
生成溯源树,研究论文发展脉络
Chat Paper
正在生成论文摘要