LogADCH: Hyperspheric-Based Log Message Anomaly Detection And Classification
2023 International Conference on Frontiers of Robotics and Software Engineering (FRSE)(2023)
摘要
Web services have been integrated with all walks of life in society. Abnormalities in the network and services seriously affect user experience and company revenue. The system log records various information of the system, and the information-rich log messages usually show different anomalies. It is particularly important to accurately and timely identify the anomalies of the log messages and classify the anomalies. In addition, most of the current existing work only detects anomalies and does not classify them, and a few existing works require anomaly labels to classify anomalies. Based on these potential challenges, we propose an unsupervised hypersphere-based log message anomaly detection and classification method, LogADCH. We use the hypersphere loss function to let the BERT model learn compact representations of log messages and perform anomaly detection. Finally, K-Means clustering is performed on the semantic feature vectors detected as anomalies to form clusters of different types of anomalies. Compared with other works, LogADCH further classifies the abnormal types after detecting the abnormal logs, helping the operation and maintenance personnel to quickly locate the fault. We compare our method with other methods on two public datasets, and the experimental results show that LogADCH has superior performance in anomaly detection as well as anomaly classification.
更多查看译文
关键词
log analysis,anomaly identification,anomaly classification
AI 理解论文
溯源树
样例
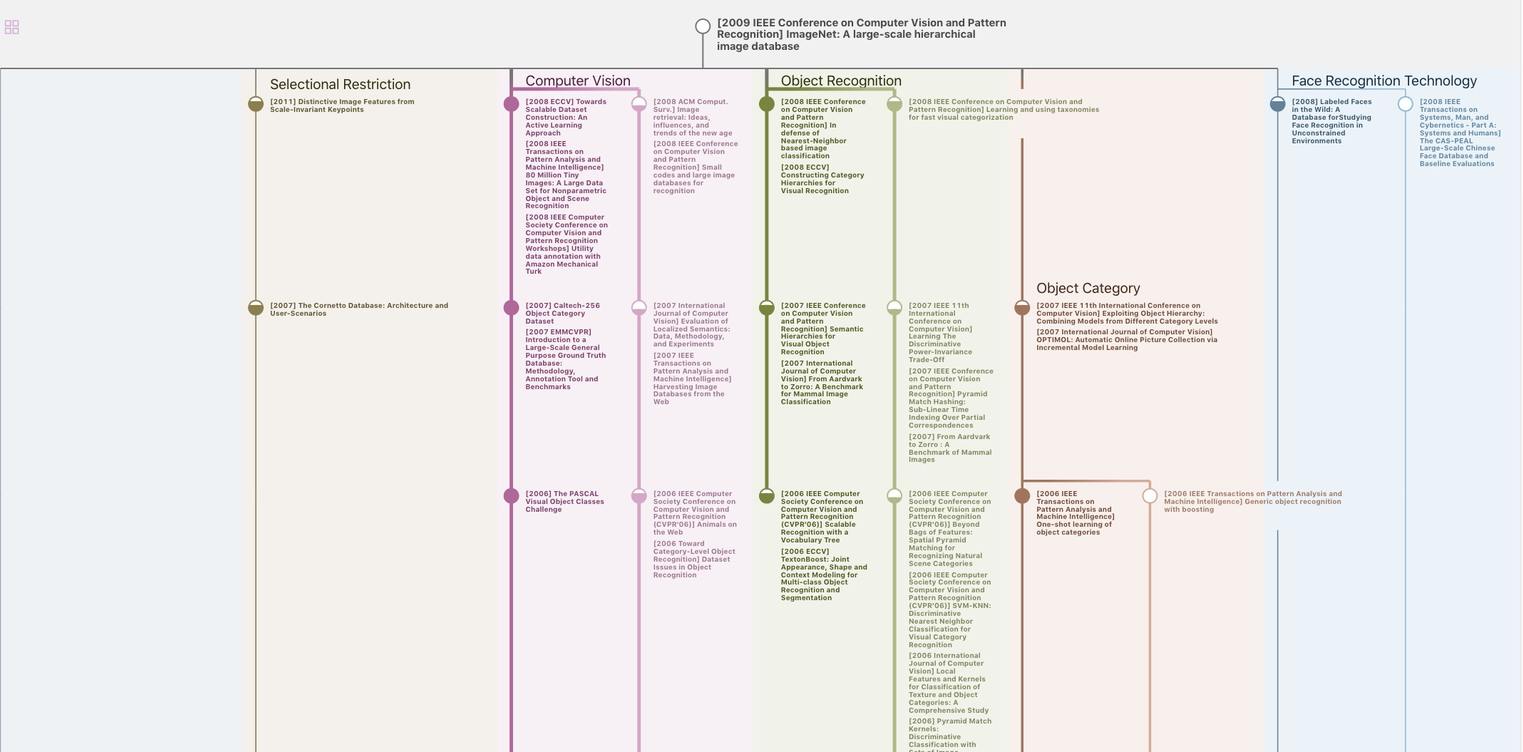
生成溯源树,研究论文发展脉络
Chat Paper
正在生成论文摘要