Learning Geometric Representations of Objects via Interaction
MACHINE LEARNING AND KNOWLEDGE DISCOVERY IN DATABASES: RESEARCH TRACK, ECML PKDD 2023, PT IV(2023)
摘要
We address the problem of learning representations from observations of a scene involving an agent and an external object the agent interacts with. To this end, we propose a representation learning framework extracting the location in physical space of both the agent and the object from unstructured observations of arbitrary nature. Our framework relies on the actions performed by the agent as the only source of supervision, while assuming that the object is displaced by the agent via unknown dynamics. We provide a theoretical foundation and formally prove that an ideal learner is guaranteed to infer an isometric representation, disentangling the agent from the object and correctly extracting their locations. We evaluate empirically our framework on a variety of scenarios, showing that it outperforms vision-based approaches such as a state-of-the-art keypoint extractor. We moreover demonstrate how the extracted representations enable the agent to solve downstream tasks via reinforcement learning in an efficient manner.
更多查看译文
关键词
Representation Learning,Equivariance,Interaction
AI 理解论文
溯源树
样例
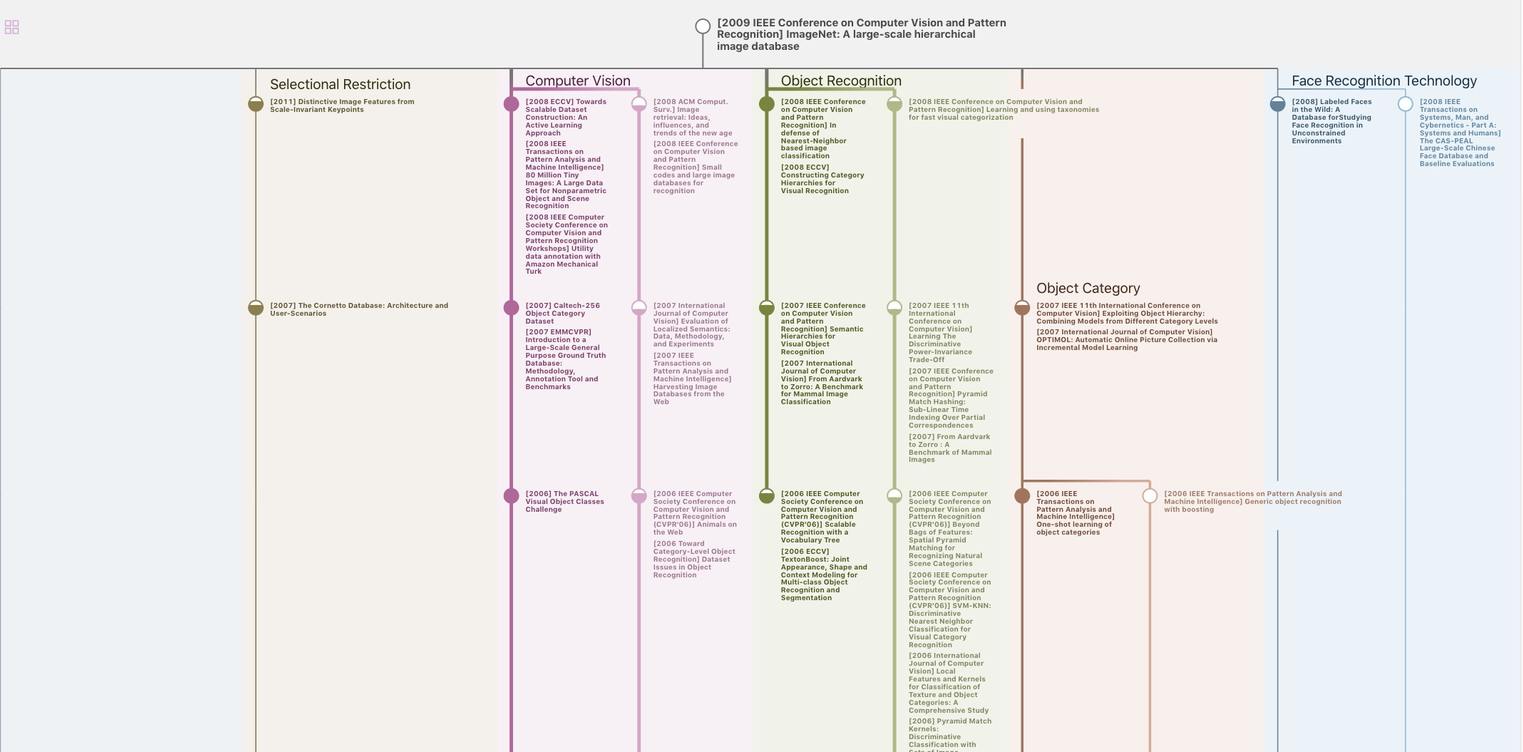
生成溯源树,研究论文发展脉络
Chat Paper
正在生成论文摘要