Neural Koopman prior for data assimilation
CoRR(2023)
摘要
With the increasing availability of large scale datasets, computational power and tools like automatic differentiation and expressive neural network architectures, sequential data are now often treated in a data-driven way, with a dynamical model trained from the observation data. While neural networks are often seen as uninterpretable black-box architectures, they can still benefit from physical priors on the data and from mathematical knowledge. In this paper, we use a neural network architecture which leverages the long-known Koopman operator theory to embed dynamical systems in latent spaces where their dynamics can be described linearly, enabling a number of appealing features. We introduce methods that enable to train such a model for long-term continuous reconstruction, even in difficult contexts where the data comes in irregularly-sampled time series. The potential for self-supervised learning is also demonstrated, as we show the promising use of trained dynamical models as priors for variational data assimilation techniques, with applications to e.g. time series interpolation and forecasting.
更多查看译文
关键词
neural
AI 理解论文
溯源树
样例
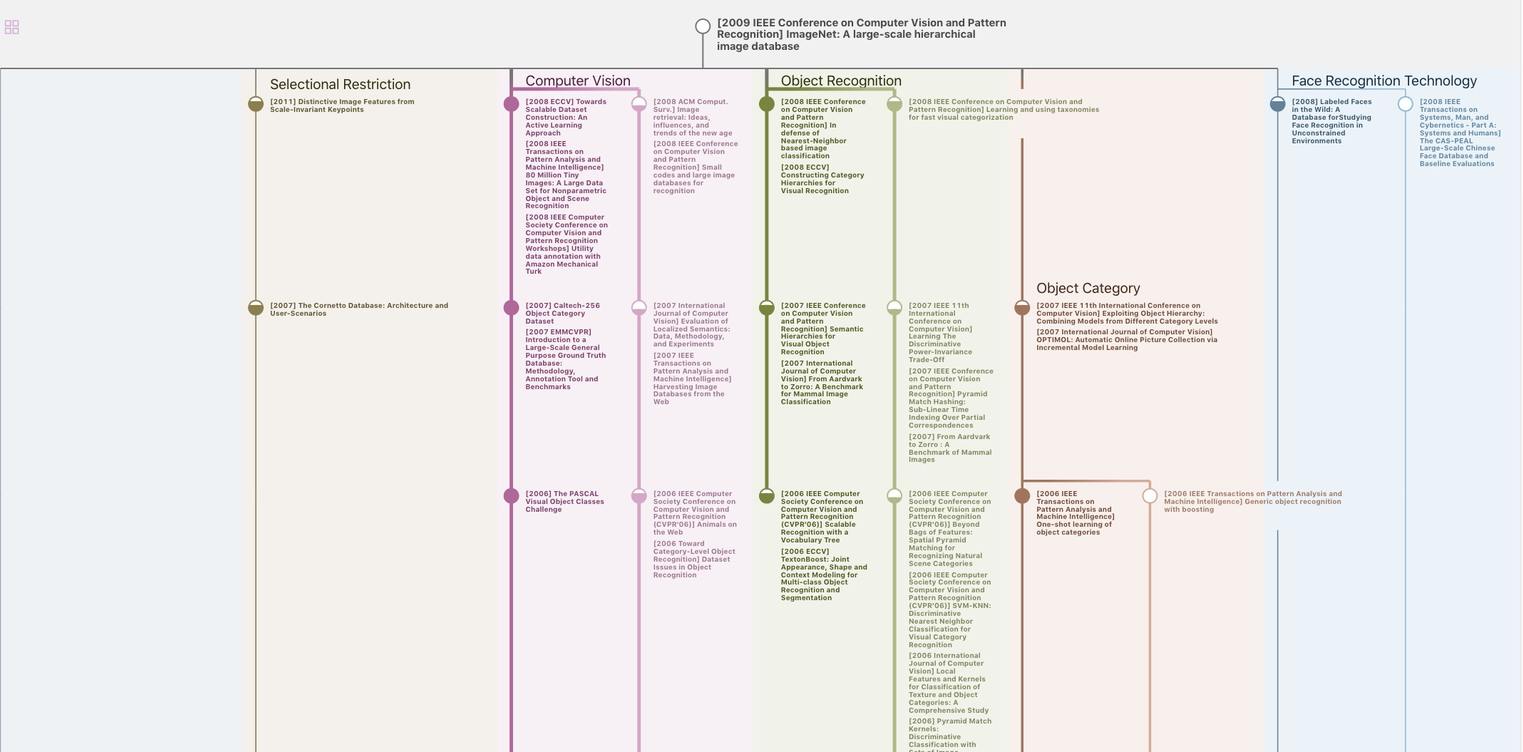
生成溯源树,研究论文发展脉络
Chat Paper
正在生成论文摘要