Advantage Actor-Critic with Reasoner: Explaining the Agent's Behavior from an Exploratory Perspective
CoRR(2023)
摘要
Reinforcement learning (RL) is a powerful tool for solving complex decision-making problems, but its lack of transparency and interpretability has been a major challenge in domains where decisions have significant real-world consequences. In this paper, we propose a novel Advantage Actor-Critic with Reasoner (A2CR), which can be easily applied to Actor-Critic-based RL models and make them interpretable. A2CR consists of three interconnected networks: the Policy Network, the Value Network, and the Reasoner Network. By predefining and classifying the underlying purpose of the actor's actions, A2CR automatically generates a more comprehensive and interpretable paradigm for understanding the agent's decision-making process. It offers a range of functionalities such as purpose-based saliency, early failure detection, and model supervision, thereby promoting responsible and trustworthy RL. Evaluations conducted in action-rich Super Mario Bros environments yield intriguing findings: Reasoner-predicted label proportions decrease for ``Breakout" and increase for ``Hovering" as the exploration level of the RL algorithm intensifies. Additionally, purpose-based saliencies are more focused and comprehensible.
更多查看译文
关键词
agent,reasoner,advantage,behavior
AI 理解论文
溯源树
样例
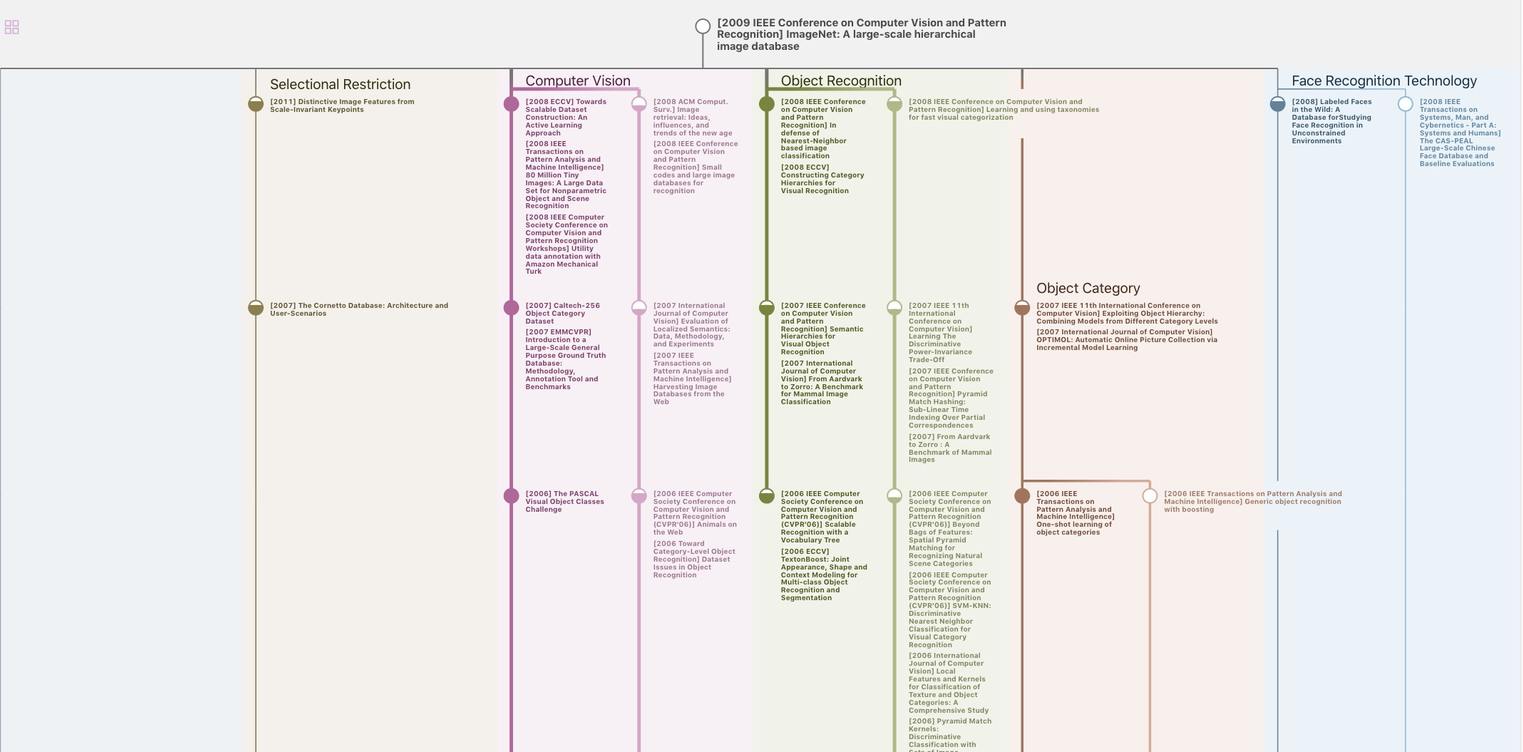
生成溯源树,研究论文发展脉络
Chat Paper
正在生成论文摘要