Exploring Robust Features for Improving Adversarial Robustness.
IEEE transactions on cybernetics(2024)
摘要
While deep neural networks (DNNs) have revolutionized many fields, their fragility to carefully designed adversarial attacks impedes the usage of DNNs in safety-critical applications. In this article, we strive to explore the robust features that are not affected by the adversarial perturbations, that is, invariant to the clean image and its adversarial examples (AEs), to improve the model's adversarial robustness. Specifically, we propose a feature disentanglement model to segregate the robust features from nonrobust features and domain-specific features. The extensive experiments on five widely used datasets with different attacks demonstrate that robust features obtained from our model improve the model's adversarial robustness compared to the state-of-the-art approaches. Moreover, the trained domain discriminator is able to identify the domain-specific features from the clean images and AEs almost perfectly. This enables AE detection without incurring additional computational costs. With that, we can also specify different classifiers for clean images and AEs, thereby avoiding any drop in clean image accuracy.
更多查看译文
关键词
Adversarial attacks,disentangle,robustness
AI 理解论文
溯源树
样例
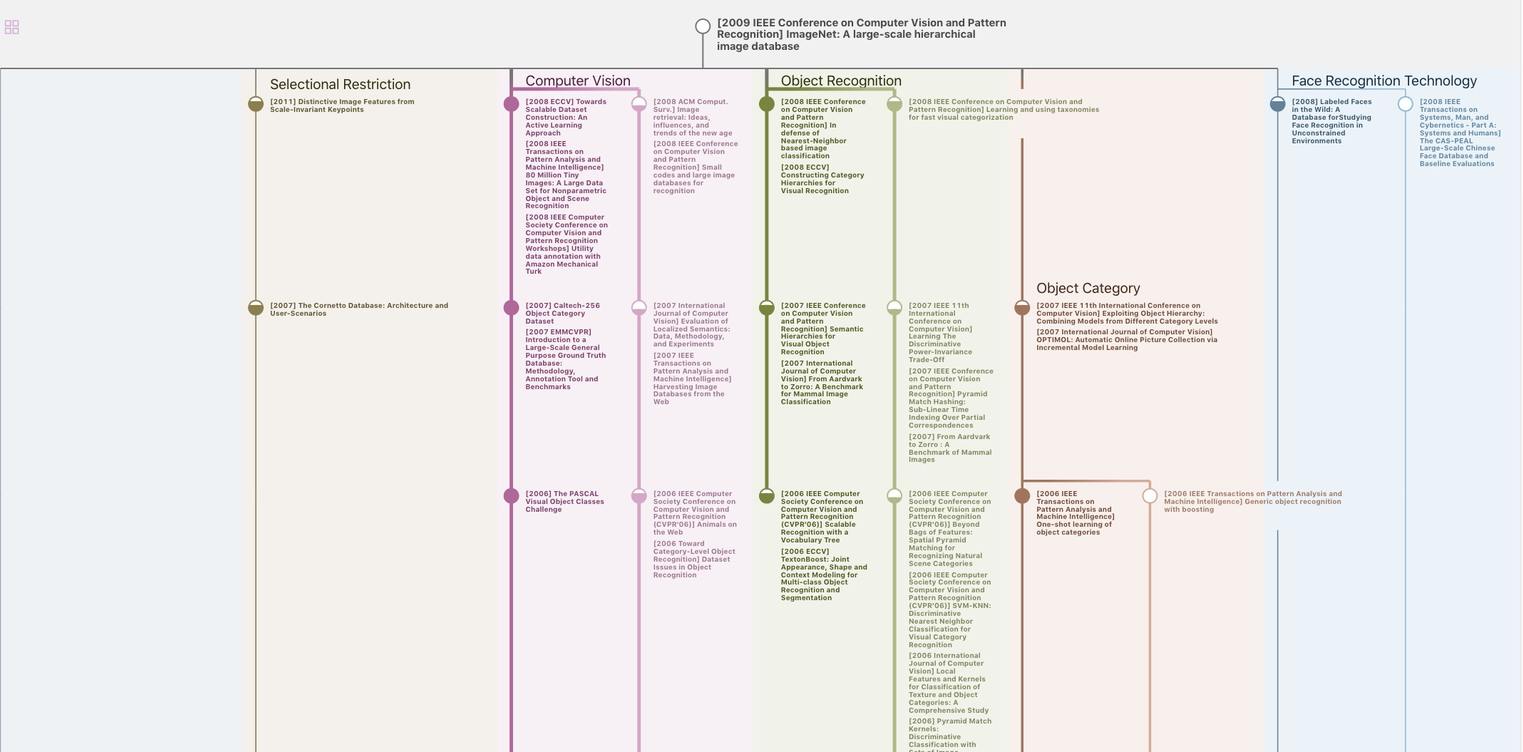
生成溯源树,研究论文发展脉络
Chat Paper
正在生成论文摘要