An adaptive Bayesian approach to gradient-free global optimization
NEW JOURNAL OF PHYSICS(2024)
摘要
Many problems in science and technology require finding global minima or maxima of complicated objective functions. The importance of global optimization has inspired the development of numerous heuristic algorithms based on analogies with physical, chemical or biological systems. Here we present a novel algorithm, SmartRunner, which employs a Bayesian probabilistic model informed by the history of accepted and rejected moves to make an informed decision about the next random trial. Thus, SmartRunner intelligently adapts its search strategy to a given objective function and moveset, with the goal of maximizing fitness gain (or energy loss) per function evaluation. Our approach is equivalent to adding a simple adaptive penalty to the original objective function, with SmartRunner performing hill ascent on the modified landscape. The adaptive penalty can be added to many other global optimization schemes, enhancing their ability to find high-quality solutions. We have explored SmartRunner's performance on a standard set of test functions, the Sherrington-Kirkpatrick spin glass model, and Kauffman's NK fitness model, finding that it compares favorably with several widely-used alternative approaches to gradient-free optimization.
更多查看译文
关键词
gradient-free global optimization,global optimization algorithms,spin glasses,fitness landscapes
AI 理解论文
溯源树
样例
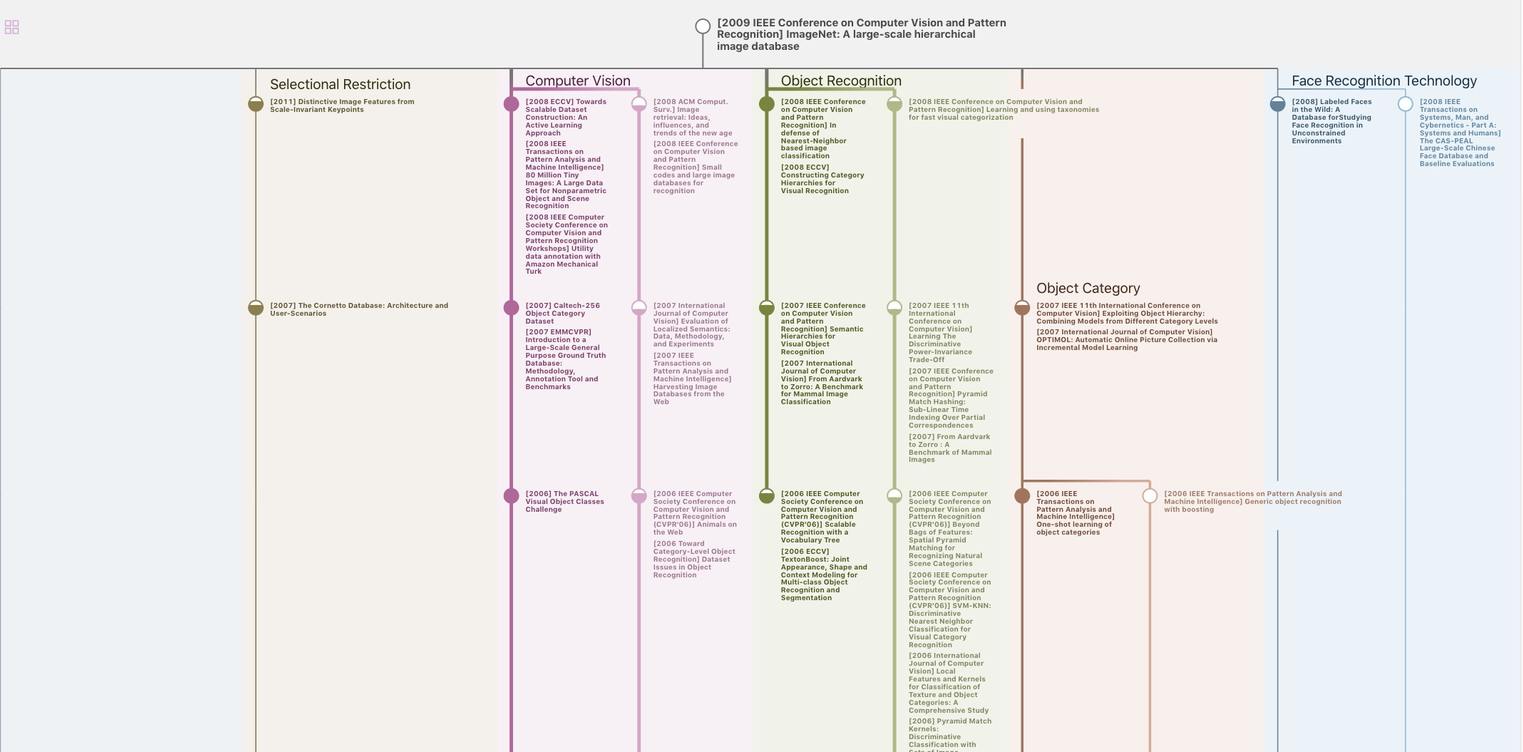
生成溯源树,研究论文发展脉络
Chat Paper
正在生成论文摘要