Unsupervised Gaze-aware Contrastive Learning with Subject-specific Condition
CoRR(2023)
摘要
Appearance-based gaze estimation has shown great promise in many applications by using a single general-purpose camera as the input device. However, its success is highly depending on the availability of large-scale well-annotated gaze datasets, which are sparse and expensive to collect. To alleviate this challenge we propose ConGaze, a contrastive learning-based framework that leverages unlabeled facial images to learn generic gaze-aware representations across subjects in an unsupervised way. Specifically, we introduce the gaze-specific data augmentation to preserve the gaze-semantic features and maintain the gaze consistency, which are proven to be crucial for effective contrastive gaze representation learning. Moreover, we devise a novel subject-conditional projection module that encourages a share feature extractor to learn gaze-aware and generic representations. Our experiments on three public gaze estimation datasets show that ConGaze outperforms existing unsupervised learning solutions by 6.7% to 22.5%; and achieves 15.1% to 24.6% improvement over its supervised learning-based counterpart in cross-dataset evaluations.
更多查看译文
关键词
learning,condition,gaze-aware,subject-specific
AI 理解论文
溯源树
样例
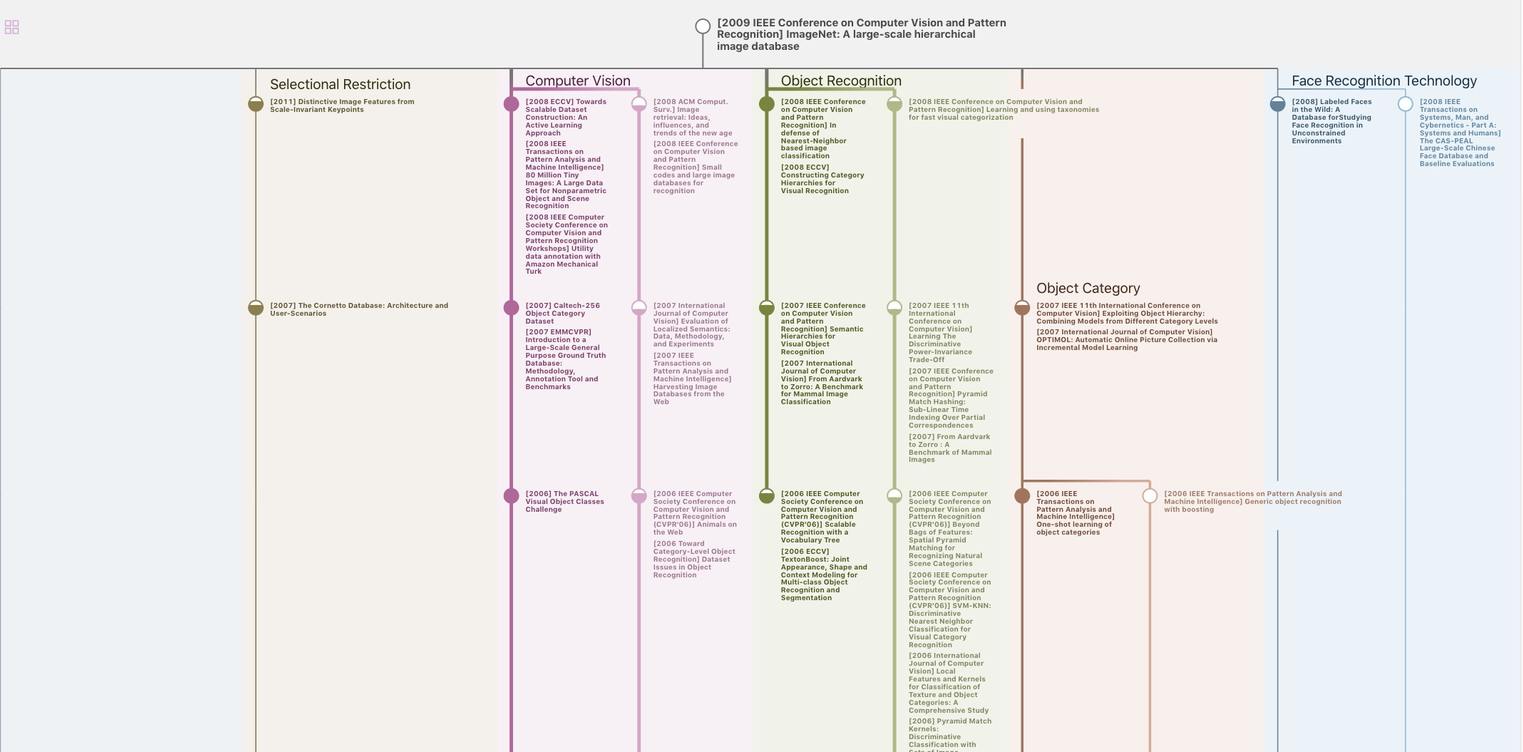
生成溯源树,研究论文发展脉络
Chat Paper
正在生成论文摘要