Sample-Efficient Co-Design of Robotic Agents Using Multi-fidelity Training on Universal Policy Network
CoRR(2023)
摘要
Co-design involves simultaneously optimizing the controller and agents physical design. Its inherent bi-level optimization formulation necessitates an outer loop design optimization driven by an inner loop control optimization. This can be challenging when the design space is large and each design evaluation involves data-intensive reinforcement learning process for control optimization. To improve the sample-efficiency we propose a multi-fidelity-based design exploration strategy based on Hyperband where we tie the controllers learnt across the design spaces through a universal policy learner for warm-starting the subsequent controller learning problems. Further, we recommend a particular way of traversing the Hyperband generated design matrix that ensures that the stochasticity of the Hyperband is reduced the most with the increasing warm starting effect of the universal policy learner as it is strengthened with each new design evaluation. Experiments performed on a wide range of agent design problems demonstrate the superiority of our method compared to the baselines. Additionally, analysis of the optimized designs shows interesting design alterations including design simplifications and non-intuitive alterations that have emerged in the biological world.
更多查看译文
关键词
robotic agents,training,sample-efficient,co-design,multi-fidelity
AI 理解论文
溯源树
样例
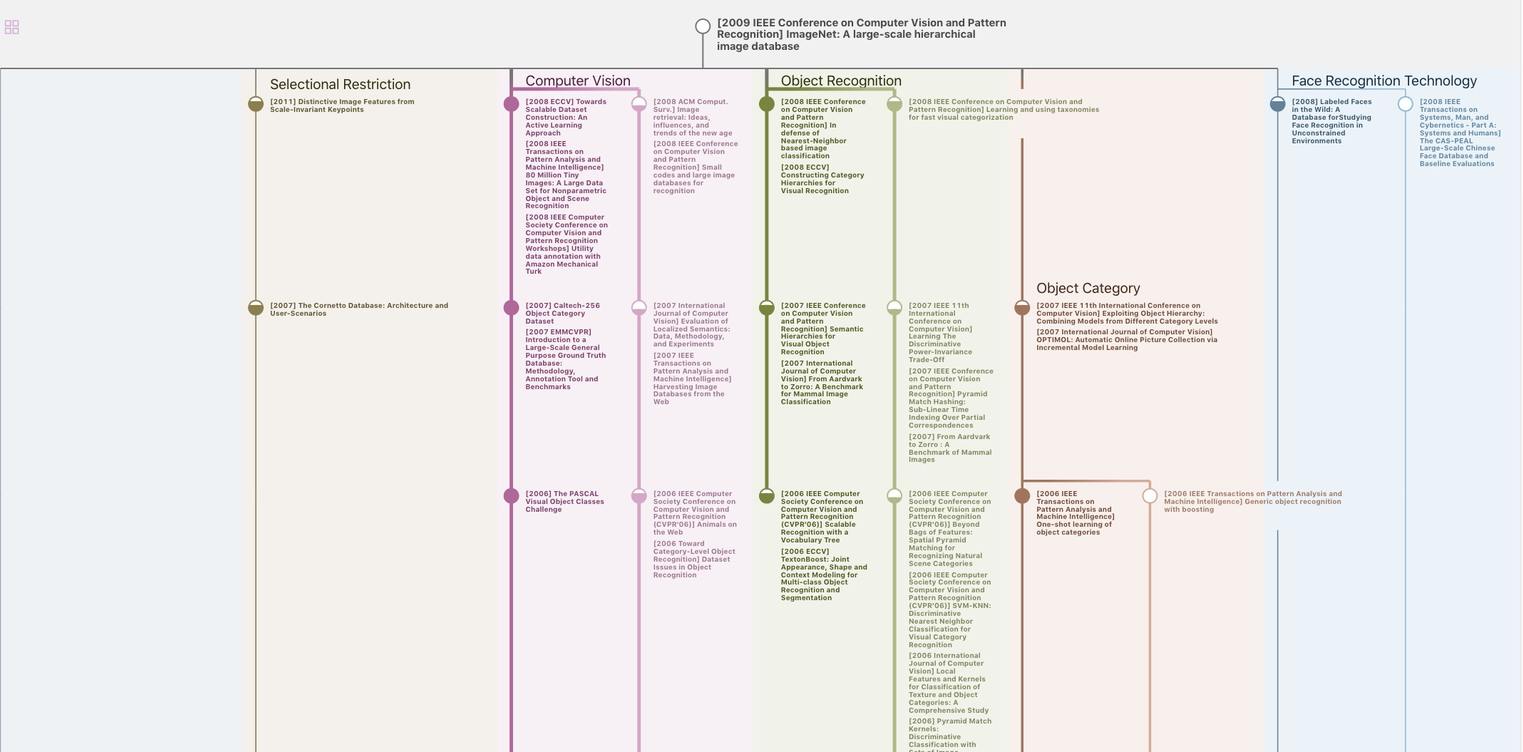
生成溯源树,研究论文发展脉络
Chat Paper
正在生成论文摘要