Random expert sampling for deep learning segmentation of acute ischemic stroke on non-contrast CT
JOURNAL OF NEUROINTERVENTIONAL SURGERY(2024)
摘要
Background Outlining acutely infarcted tissue on non-contrast CT is a challenging task for which human inter-reader agreement is limited. We explored two different methods for training a supervised deep learning algorithm: one that used a segmentation defined by majority vote among experts and another that trained randomly on separate individual expert segmentations. Methods The data set consisted of 260 non-contrast CT studies in 233 patients with acute ischemic stroke recruited from the multicenter DEFUSE 3 (Endovascular Therapy Following Imaging Evaluation for Ischemic Stroke 3) trial. Additional external validation was performed using 33 patients with matched stroke onset times from the University Hospital Lausanne. A benchmark U-Net was trained on the reference annotations of three experienced neuroradiologists to segment ischemic brain tissue using majority vote and random expert sampling training schemes. The median of volume, overlap, and distance segmentation metrics were determined for agreement in lesion segmentations between (1) three experts, (2) the majority model and each expert, and (3) the random model and each expert. The two sided Wilcoxon signed rank test was used to compare performances (1) to 2) and (1) to (3). We further compared volumes with the 24 hour follow-up diffusion weighted imaging (DWI, final infarct core) and correlations with clinical outcome (modified Rankin Scale (mRS) at 90 days) with the Spearman method. Results The random model outperformed the inter-expert agreement ((1) to (2)) and the majority model ((1) to (3)) (dice 0.51 +/- 0.04 vs 0.36 +/- 0.05 (P<0.0001) vs 0.45 +/- 0.05 (P<0.0001)). The random model predicted volume correlated with clinical outcome (0.19, P<0.05), whereas the median expert volume and majority model volume did not. There was no significant difference when comparing the volume correlations between random model, median expert volume, and majority model to 24 hour follow-up DWI volume (P>0.05, n=51). Conclusion The random model for ischemic injury delineation on non-contrast CT surpassed the inter-expert agreement ((1) to (2)) and the performance of the majority model ((1) to (3)). We showed that the random model volumetric measures of the model were consistent with 24 hour follow-up DWI.
更多查看译文
关键词
thrombectomy,stroke,CT
AI 理解论文
溯源树
样例
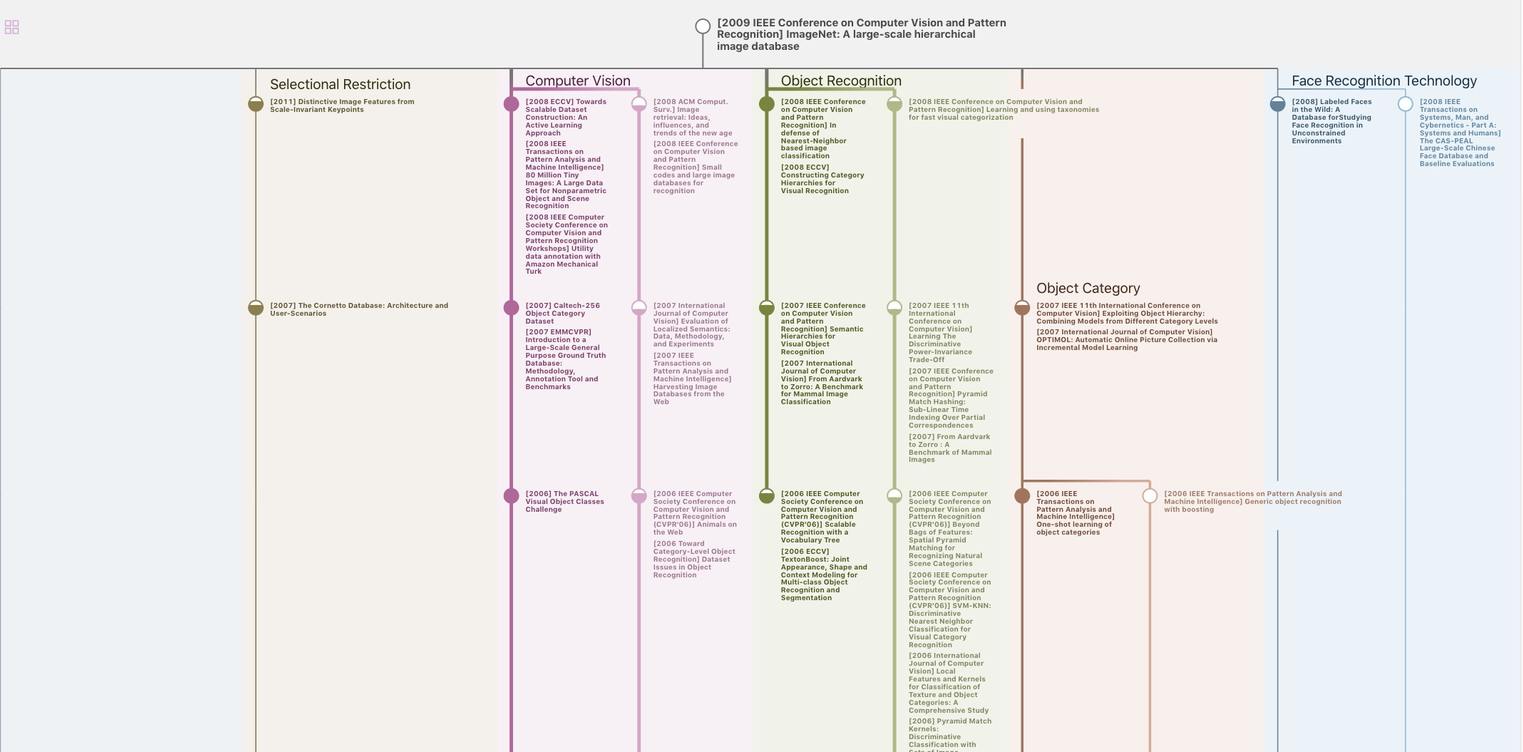
生成溯源树,研究论文发展脉络
Chat Paper
正在生成论文摘要