Design of New Inorganic Crystals with the Desired Composition Using Deep Learning.
Journal of chemical information and modeling(2023)
摘要
New solid-state materials have been discovered using various approaches from atom substitution in density functional theory (DFT) to generative models in machine learning. Recently, generative models have shown promising performance in finding new materials. Crystal generation with deep learning has been applied in various methods to discover new crystals. However, most generative models can only be applied to materials with specific elements or generate structures with random compositions. In this work, we developed a model that can generate crystals with desired compositions based on a crystal diffusion variational autoencoder. We generated crystal structures for 14 compositions of three types of materials in different applications. The generated structures were further stabilized using DFT calculations. We found the most stable structures in the existing database for all but one composition, even though eight compositions among them were not in the data set trained in a crystal diffusion variational autoencoder. This substantiates the prospect of the generation of an extensive range of compositions. Finally, 205 unique new crystal materials with energy above hull <100 meV/atom were generated. Moreover, we compared the average formation energy of the crystals generated from five compositions, two of which were hypothetical, with that of traditional methods like atom substitution and a generative model. The generated structures had lower formation energy than those of other models, except for one composition. These results demonstrate that our approach can be applied stably in various fields to design stable inorganic materials based on machine learning.
更多查看译文
关键词
new inorganic crystals,deep learning
AI 理解论文
溯源树
样例
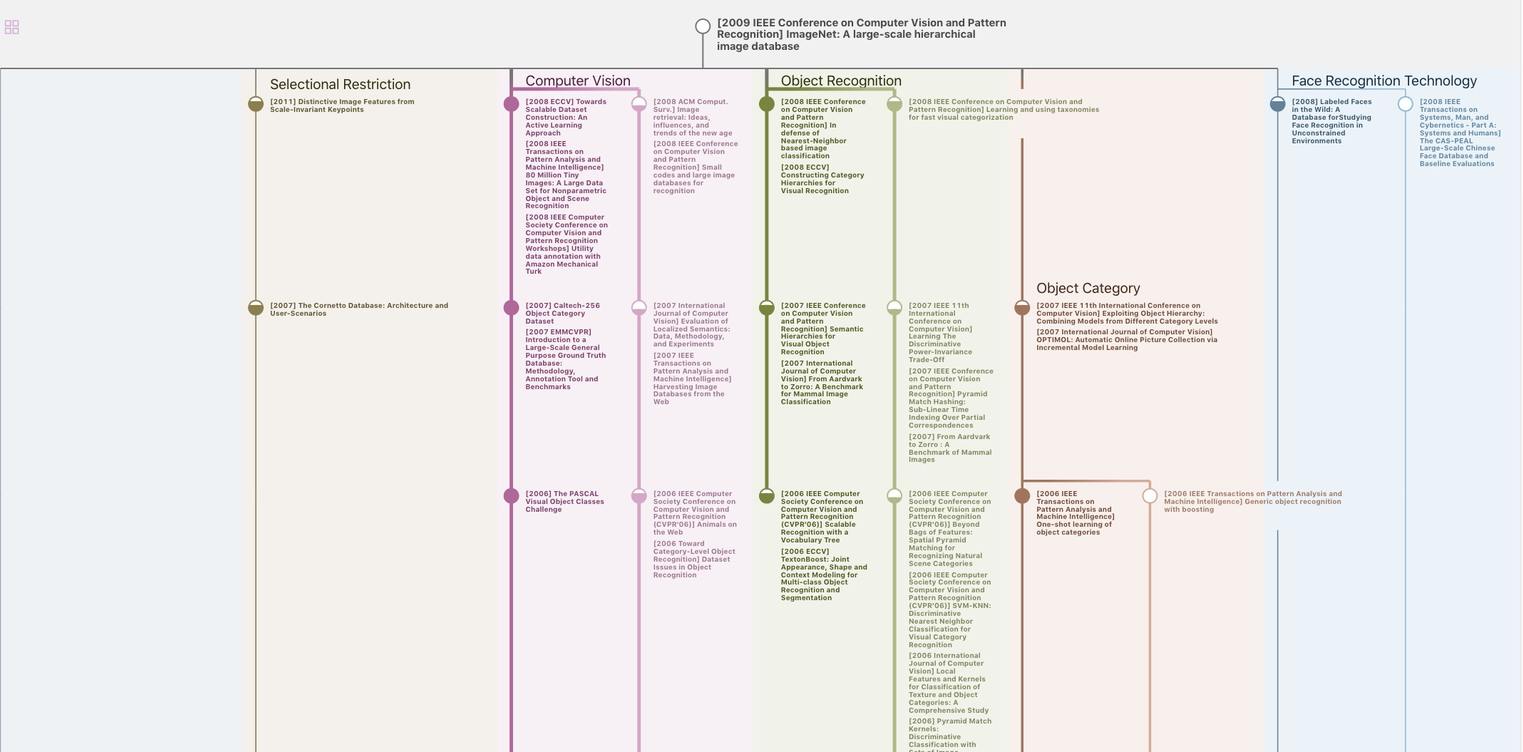
生成溯源树,研究论文发展脉络
Chat Paper
正在生成论文摘要