Data-Driven Imputation of Miscibility of Aqueous Solutions via Graph-Regularized Logistic Matrix Factorization.
The journal of physical chemistry. B(2023)
摘要
Aqueous, two-phase systems (ATPSs) may form upon mixing two solutions of independently water-soluble compounds. Many separation, purification, and extraction processes rely on ATPSs. Predicting the miscibility of solutions can accelerate and reduce the cost of the discovery of new ATPSs for these applications. Whereas previous machine learning approaches to ATPS prediction used physicochemical properties of each solute as a descriptor, in this work, we show how to impute missing miscibility outcomes directly from an incomplete collection of pairwise miscibility experiments. We use graph-regularized logistic matrix factorization (GR-LMF) to learn a latent vector of each solution from (i) the observed entries in the pairwise miscibility matrix and (ii) a graph where each node is a solution and edges are relationships indicating the general category of the solute (i.e., polymer, surfactant, salt, protein). For an experimental data set of the pairwise miscibility of 68 solutions from Peacock et al. [ , , 11449-11460], we find that GR-LMF more accurately predicts missing (im)miscibility outcomes of pairs of solutions than ordinary logistic matrix factorization and random forest classifiers that use physicochemical features of the solutes. GR-LMF obviates the need for features of the solutions and solutions to impute missing miscibility outcomes, but it cannot predict the miscibility of a new solution without some observations of its miscibility with other solutions in the training data set.
更多查看译文
关键词
aqueous solutions,imputation,factorization,miscibility,data-driven,graph-regularized
AI 理解论文
溯源树
样例
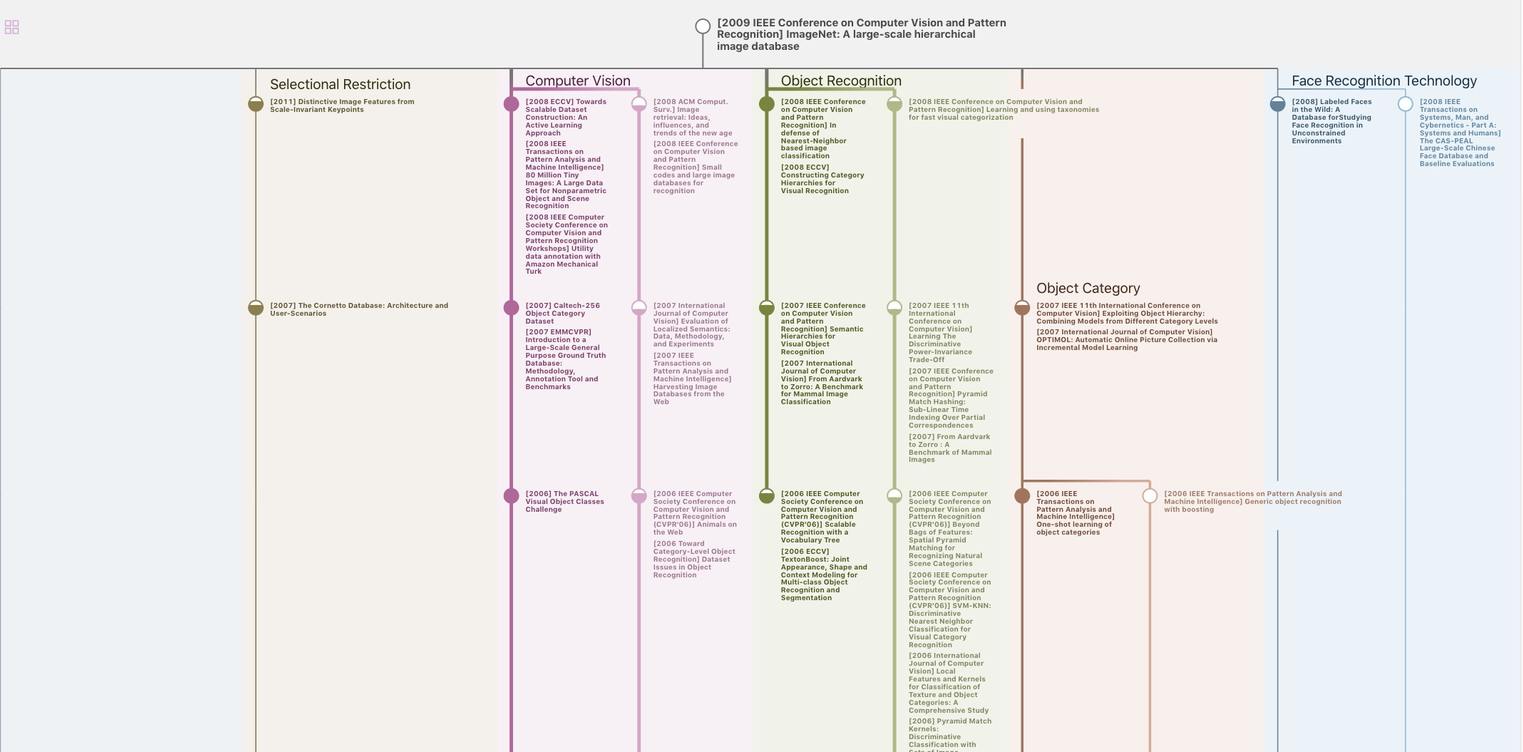
生成溯源树,研究论文发展脉络
Chat Paper
正在生成论文摘要