ScatterNet for projection-based 4D cone-beam computed tomography intensity correction of lung cancer patients.
Physics and imaging in radiation oncology(2023)
摘要
In radiotherapy, dose calculations based on 4D cone beam CTs (4DCBCTs) require image intensity corrections. This retrospective study compared the dose calculation accuracy of a deep learning, projection-based scatter correction workflow (ScatterNet), to slower workflows: conventional 4D projection-based scatter correction (CBCT) and a deformable image registration (DIR)-based method (4DvCT). For 26 lung cancer patients, planning CTs (pCTs), 4DCTs and CBCT projections were available. ScatterNet was trained with pairs of raw and corrected CBCT projections. Corrected projections from ScatterNet and the conventional workflow were reconstructed using MA-ROOSTER, yielding 4DCBCT and 4DCBCT. The 4DvCT was generated by 4DCT to 4DCBCT DIR, as part of the 4DCBCT workflow. Robust intensity modulated proton therapy treatment plans were created on free-breathing pCTs. 4DCBCT was compared to 4DCBCT and the 4DvCT in terms of image quality and dose calculation accuracy (dose-volume-histogram parameters and / gamma analysis). 4DCBCT resulted in an average mean absolute error of and when compared to 4DCBCT and 4DvCT respectively. High agreement was observed in targets with median dose differences of (4DCBCT-4DCBCT) and (4DCBCT-4DvCT). The gamma analysis showed high average / pass rates of for both 4DCBCT vs. 4DCBCT and 4DCBCT vs. 4DvCT. Accurate 4D dose calculations are feasible for lung cancer patients using ScatterNet for 4DCBCT correction. Average scatter correction times could be reduced from (4DCBCT) to , showing the clinical suitability of the proposed deep learning-based method.
更多查看译文
关键词
Proton therapy,4DCBCT,Projection-based,Deep learning,Lung cancer,Scatter correction
AI 理解论文
溯源树
样例
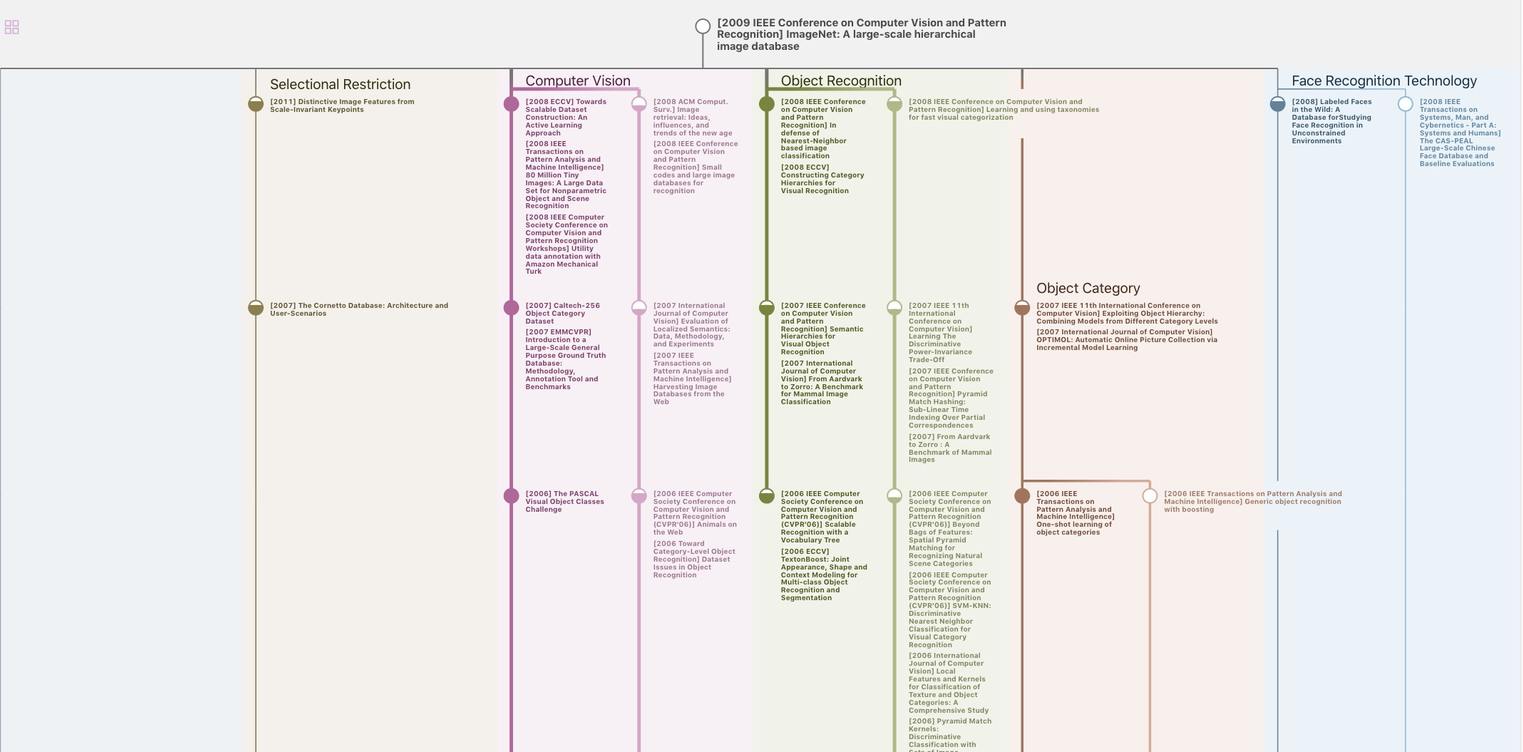
生成溯源树,研究论文发展脉络
Chat Paper
正在生成论文摘要