Improving extraction phenology accuracy using SIF coupled with the vegetation index and mapping the spatiotemporal pattern of bamboo forest phenology
Remote Sensing of Environment(2023)
摘要
Monitoring plant phenology is vital to maintaining the global carbon balance and management under climate change. Bamboo forest is an essential forest type in subtropical China with a strong carbon sequestration capacity. In recent years, vegetation indices (VIs), which characterize canopy structural parameters, and solar-induced chlorophyll fluorescence (SIF), indicating the photosynthetic activity of vegetation, have provided new perspectives on plant phenology at regional and global scales. However, the best data sources and methods for extracting the phenology of bamboo forests remain to be explored. In this study, new vegetation indices were innovatively constructed by normalizing the VIs (enhanced vegetation index (EVI), two-band enhanced vegetation index (EVI2), and near-infrared reflectance of vegetation (NIRv)) based on Moderate Resolution Imaging Spectroradiometer (MODIS) products and SIF products (GOSIF) based on OCO-2 satellites and then taking the mean values of the normalized VIs (EVI, EVI2 and NIRv) and SIF. We called the new indices SVs (SIF and VIs combined indices, including Se (SIF and EVI combined index), Se2 (SIF and EVI2 combined index), or Sn (SIF and NIRv combined index)). Two time series reconstruction methods (asymmetric Gaussian (AG) function fitting and double logistic (DL) function) and two extractive phenology parameter methods (dynamic threshold method (DT) and comparative threshold method (CT)) were employed to extract phenological information. The advantages of SVs for extracting bamboo forest phenology (BFP) were verified by comparing the extraction performance of VIs, SIF, and SVs on the SOS and EOS of bamboo forests. Thus, the best way to extract BFP was explored, and the spatial distribution and spatial-temporal variation characteristics of BFP in China from 2011 to 2020 were analyzed. The results are described as follows: (1) SVs are better able to extract BFP parameters compared with VIs and SIF, especially in bamboo forest-specific off-years and on-years; (2) SIF has better accuracy than VIs in extracting BFP, where both SOS and EOS values obtained from VIs are overestimated, and SIF can reflect BFP information earlier; and (3) the best data sources for extracting SOS and EOS in bamboo forests are Sn and Se, respectively, and the optimal methods are AG_CT and DL_DT, respectively. Compared with SIF, the R2 values of Sn and Se extracted SOS and EOS are improved by 40.7% and 7.7%, and the RMSE values are reduced by 24.7% and 0.7%, respectively; and (4) the SOS for bamboo forests in China from 2011 to 2020 was mainly concentrated in 80–100 days, with an overall advancing trend; the EOS was mainly concentrated in 300–320 days, with an overall delay. The results show that the SVs obtained by coupling VIs and SIF can better track the BFP information, providing a practical reference for macroscopic monitoring of BFP based on medium-resolution time series data.
更多查看译文
关键词
extraction phenology accuracy,vegetation index,forest
AI 理解论文
溯源树
样例
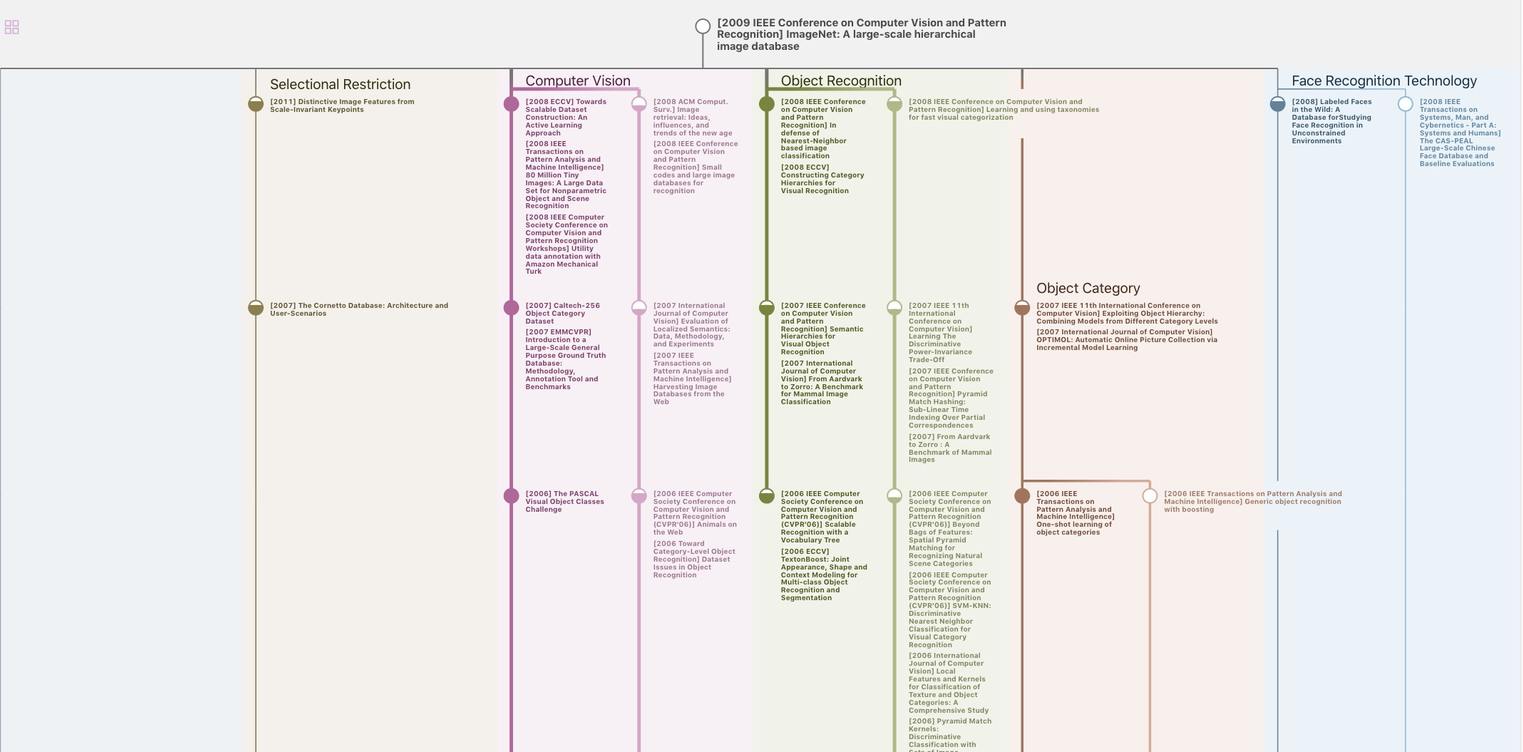
生成溯源树,研究论文发展脉络
Chat Paper
正在生成论文摘要