An optimization framework for RIS-based energy-efficient multi-cell NOMA systems
VEHICULAR COMMUNICATIONS(2023)
摘要
Non-orthogonal multiple access (NOMA) is a highly promising multiple access strategy for wideband communication systems due to its high spectral efficiency. However, in multi-cell multi-band systems, interference issues can arise from resource sharing among multiple users, which limits the potential of NOMA. To tackle these issues, in this paper, we study a NOMA system where reconfigurable intelligent surfaces (RIS) are employed across multiple cells with multiple users clusters. Specifically, we propose an optimization framework to maximize the system energy efficiency (EE) through jointly designing the transmission beamformer at each base station, the RIS phase shifts and the corresponding binary selection matrix indicating which elements of the RIS should be used to serve a specific cell. Our optimization framework takes into account the minimum rate requirements of users, the successive interference cancellation (SIC) decoding-based rate constraint, fairness among users as well as the maximum allowed transmission power. The resulting non-convex problem is solved by using an alternating optimization algorithm, wherein semidefinite programming (SDP), successive convex approximation (SCA), and penalty-based difference-of-convex (DC) programming are used to reach a solution. Simulation results show that the proposed system outperforms conventional orthogonal access in terms of EE performance, the achievable rate, and power consumption. Also, the results revealed the critical transmission power level for NOMA beyond which the EE starts decaying. Moreover, the proposed NOMA system shows a limited EE performance compared to the conventional orthogonal access as the number of clusters exceeds a certain limit.(c) 2023 Elsevier Inc. All rights reserved.
更多查看译文
关键词
Reconfigurable intelligent surfaces (RIS),Alternating optimization,Energy efficiency (EE),Non-orthogonal multiple access (NOMA)
AI 理解论文
溯源树
样例
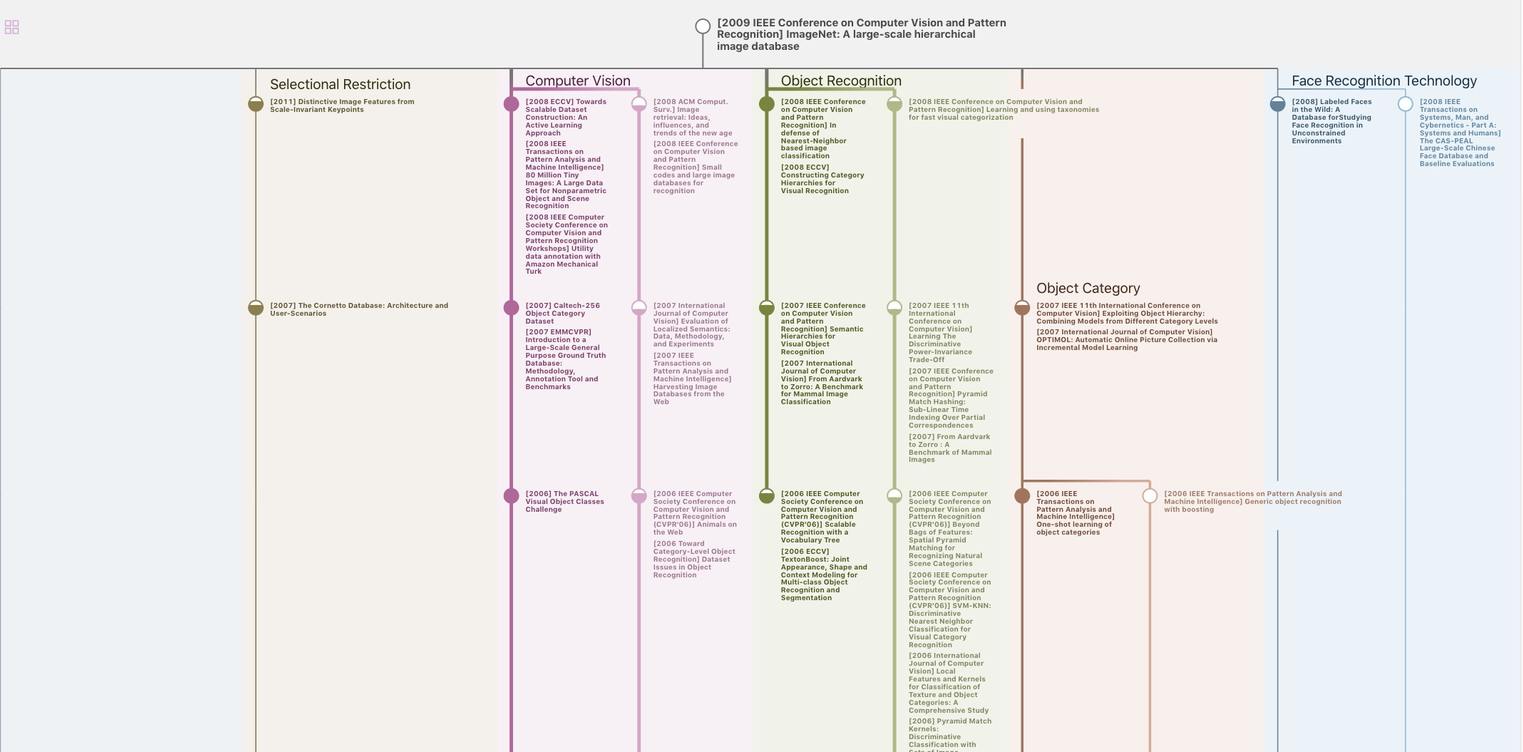
生成溯源树,研究论文发展脉络
Chat Paper
正在生成论文摘要