A self-sustained EV charging framework with N-step deep reinforcement learning
Sustainable Energy, Grids and Networks(2023)
摘要
Decarbonization of the transport sector is a major challenge in the transition towards net-zero emissions. Even though the penetration of electric vehicles (EV) in the passenger vehicle fleet is increasing, the energy mix is not yet dominated by renewables. This leads to the utilization of fossil-based power generation for EV charging, especially during peak hours. In this work, we introduce a residential smart EV charging framework that prioritizes solar photovoltaic (PV) power self-consumption to accelerate the transition to a carbon neutral passenger vehicle fleet. Our approach employs N-Step Deep Reinforcement Learning to charge the EV with clean energy from a PV, without neglecting other major factors that influence end users’ behavior, such as electricity cost or EV charging tendencies. Historical smart-meter data from the Pecan Street dataset on total consumption, EV demand and solar generation has been utilized as input features to train the Deep RL method so that it decides on a real-time basis whether to charge or not the EV, without the need for foresight of future observations. Experimental results on six residential houses validate that, compared to uncontrolled EV charging, the proposed method can increase the average self-consumption of solar energy for EV charging by 19.66%, as well as reduce network stress by 7% and electricity bill by 10.3%.
更多查看译文
关键词
Smart grid, Smart charging, Demand response, Electric vehicle, Solar power, Self-consumption
AI 理解论文
溯源树
样例
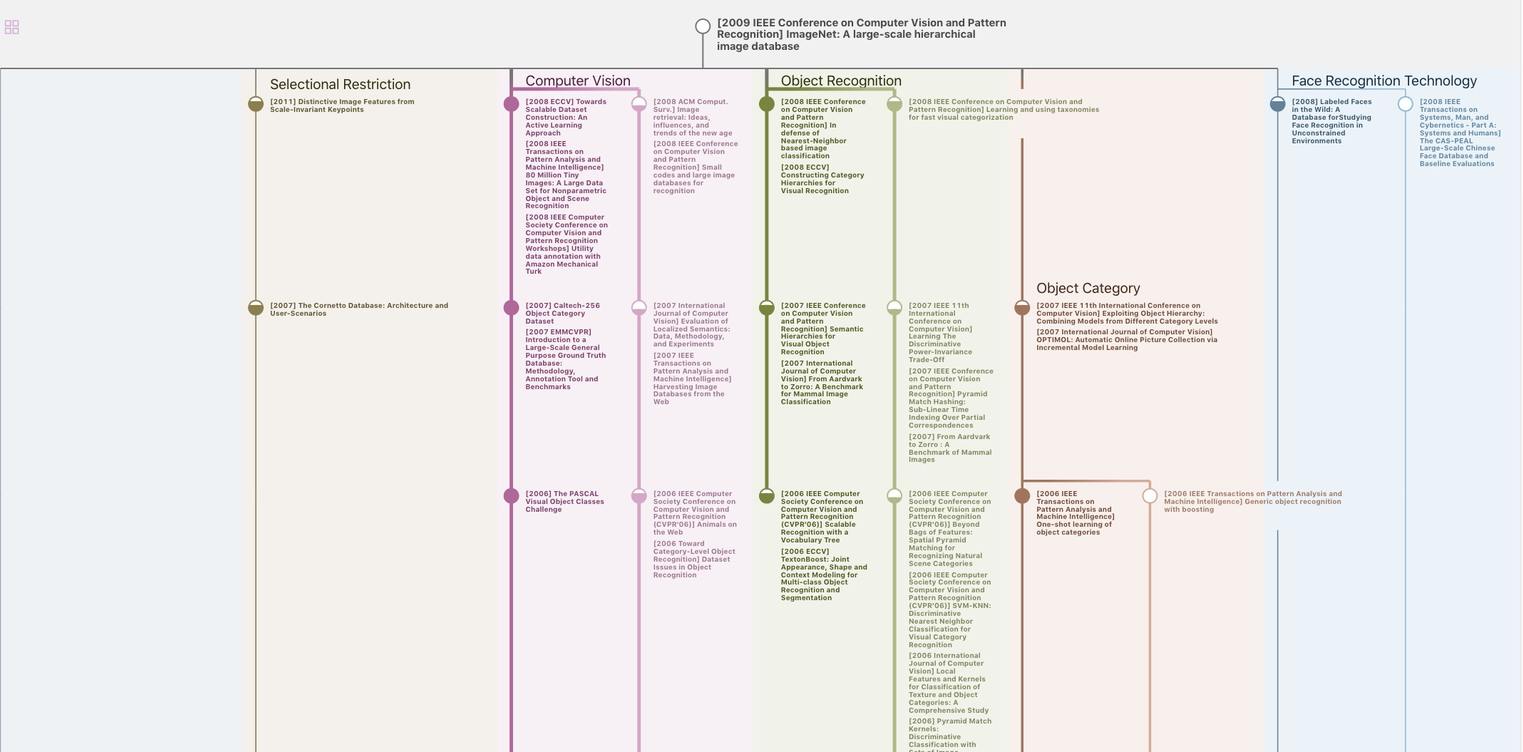
生成溯源树,研究论文发展脉络
Chat Paper
正在生成论文摘要