To see further: Knowledge graph-aware deep graph convolutional network for recommender systems
Information Sciences(2023)
摘要
Applying a graph convolutional network (GCN) or its variants to user-item interaction graphs is one of the most commonly used approaches for learning the representation of users and items in modern recommender systems. However, these models perform poorly in recommending high-order items due to the over-smoothing when the GCN deepens and the lack of connectivity between the cold-start items and the target user. To improve the ability to recommend higher-order items, we propose our Knowledge graph-aware Deep Graph Convolutional Network with Initial Residual Connection (KDGCN-IC) and Knowledge graph-aware Deep Graph Convolutional Network with Dense Connection (KDGCN-DC) methods. First, we introduce an item knowledge graph to rebuild the connectivity between nodes in the user-item graph, especially the users and the cold-start items. Then, we present our methods for improving the information flow by reusing features to alleviate the over-smoothing issue to deepen the GCN to capture the higher-order collaborative signal. More specifically, KDGCN-IC reuses the initial feature information, and KDGCN-DC reuses the feature information of each layer. Extensive experiments demonstrated that our models were able to achieve substantial improvement over state-of-the-art models in so far as their recommendation accuracy and higher-order recommendations.
更多查看译文
关键词
Recommender systems,Collaborative filtering,Knowledge graph,Deep graph convolutional network
AI 理解论文
溯源树
样例
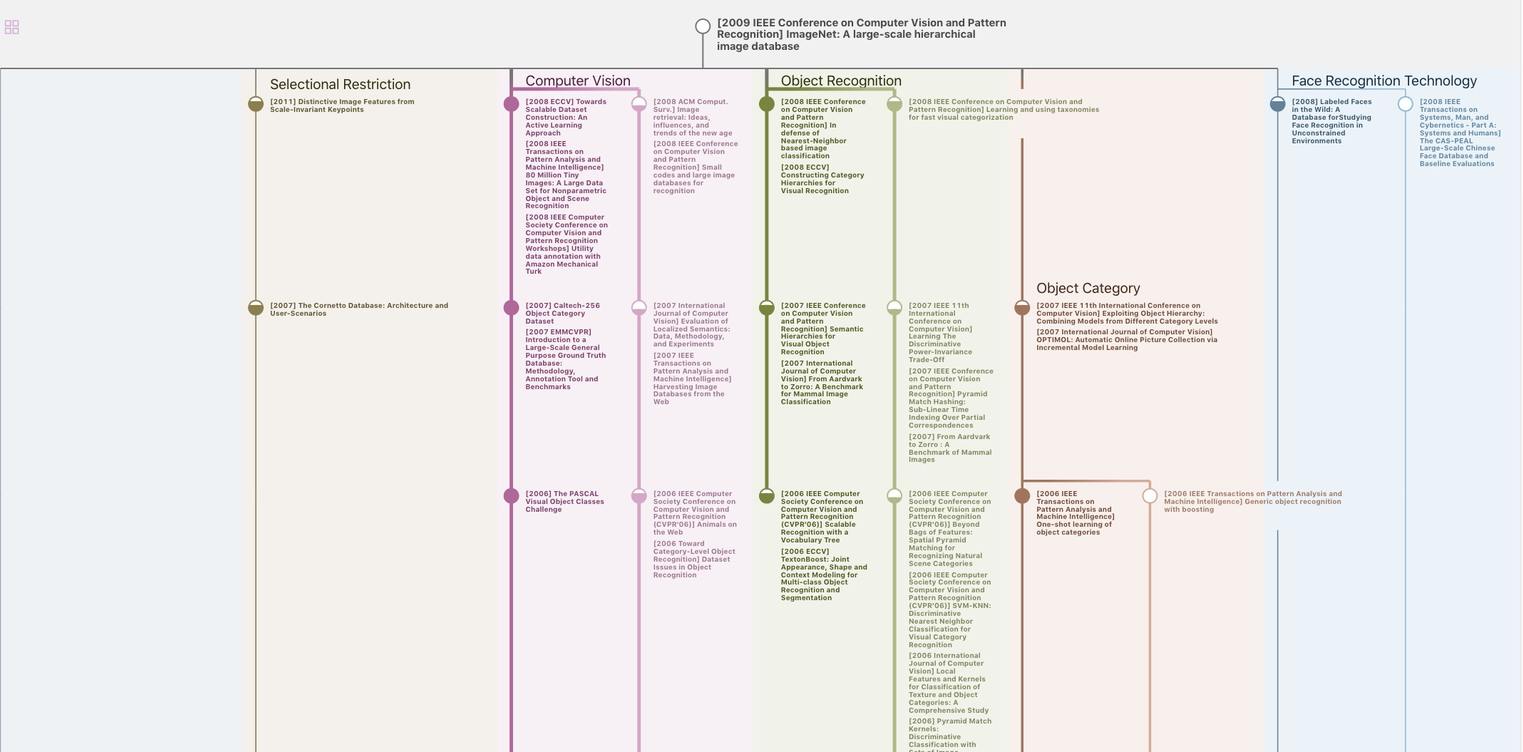
生成溯源树,研究论文发展脉络
Chat Paper
正在生成论文摘要