Myoformer: sEMG missing signal recovery for gesture recognition based on multi-channel self-attention mechanism
Biomedical Signal Processing and Control(2023)
摘要
The surface electromyography (sEMG) signal is easily lost or distorted in practice due to the influence of non-ideal factors, which decreases the robustness and accuracy of gesture recognition. We propose an end-to-end neural network architecture based on multi-channel self-attention mechanism, Myoformer, which is not only able to automatically detect and repair aberrant signals without features extraction, but also enables global signal filtering and gesture recognition. Myoformer consists of Recovery Encoder (ReEncoder), Recovery Decoder (ReDecoder), and classifier. Each single-channel signal is handled by the ReEncoder and restored in the time domain. The treated signals from all channels are then further repaired and processed by ReDecoder using mutual compensation and mutual suppression. On the basis of the signal reconstructed, the classifier recognizes gestures. The results reveal that the average similarity between the restored and ideal signals is 91.34%, and the accuracy of gesture recognition under non-ideal conditions (signal deficiency rate of 10%∼80%) is 97.62%, which is close to the accuracy under ideal conditions.
更多查看译文
关键词
Self-attention,Non-ideal conditions,Signal recovery,Surface electromyography,Gesture recognition,Robustness
AI 理解论文
溯源树
样例
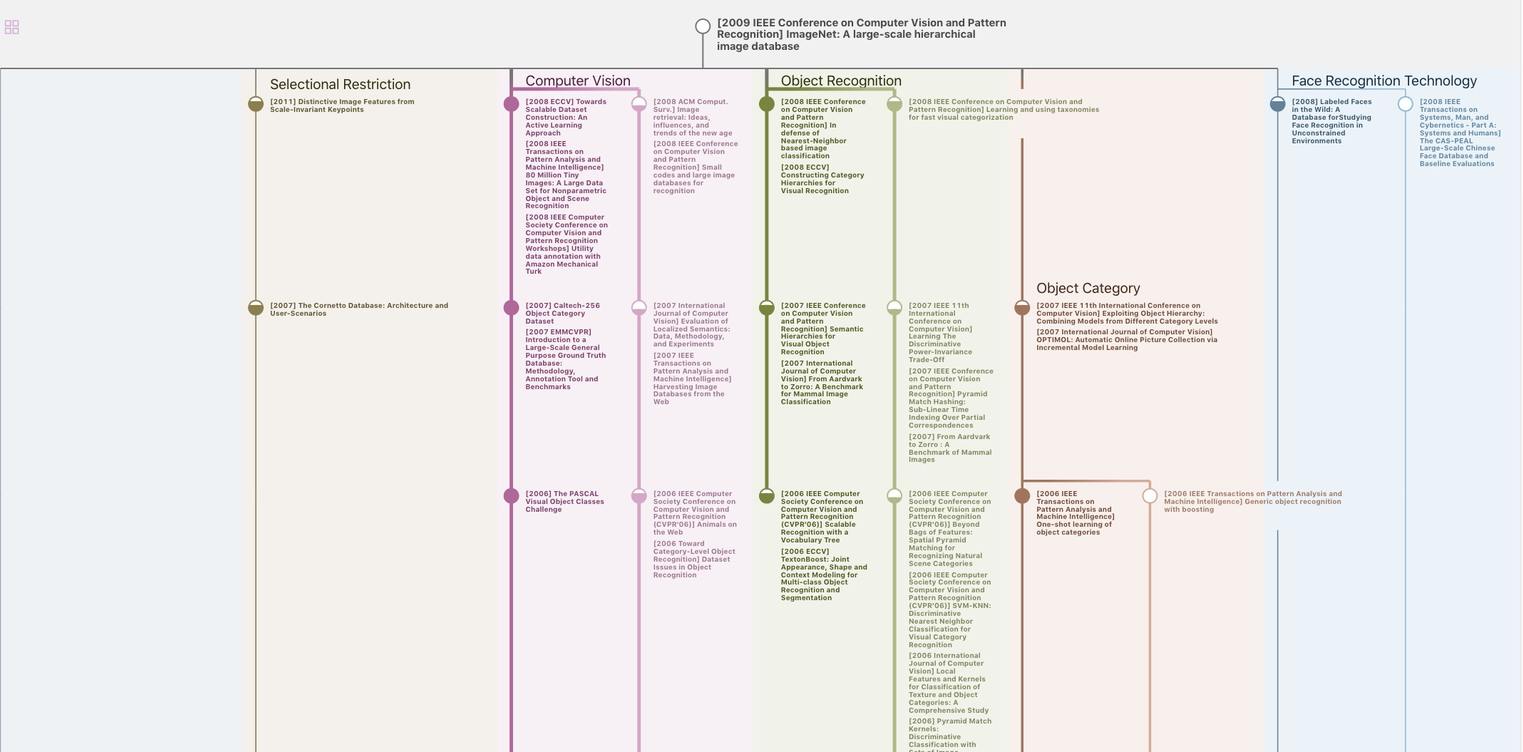
生成溯源树,研究论文发展脉络
Chat Paper
正在生成论文摘要