A study of crude oil futures price volatility based on multi-dimensional data from event-driven and deep learning perspectives
Applied Soft Computing(2023)
摘要
There are two unique challenges in current research of price volatility studies in the crude oil futures market, which is how to capture the impact of interactivity between data as much as possible when multi-dimensional data are fused, and how to solve the problem of temporal heterogeneity of research samples existing in multi-dimensional data. We proposed a tensor-based event-driven gated recurrent unit (Event-GRU) deep learning model that helps to solve the above dilemmas. Our model can effectively prevent the loss of interaction relationship of multi-dimensional data by taking the advantage of spatial data representation of tensor, as well as using news occurrence as a trigger to construct the event-driven mechanism based on GRU model, in order to overcome the feature space distortion between discrete news data and continuous trading fundamentals data due to the uneven sample time matching. Regarding the current insufficient research of crude oil prices in China, we addressed the crude oil futures trading data from the Shanghai Futures Exchange and 31,422 news data of the crude oil futures market from April 2018 to August 2020. We used the XGBoost algorithm (eXtreme Gradient Boosting) to evaluate and filter. Besides, we built a tensor-based event-GRU deep learning model to finally analyze the closing price on the day after. This research contributes to improving the accuracy of crude oil futures price analysis compared with using benchmark models such as DT, SVM, BP, LSTM, and GRU, and laying the foundation for the subsequent opening of corresponding quantitative investment strategies.
更多查看译文
关键词
Crude oil futures price,Deep learning,Event-driven mechanism,Multi-dimensional data,Tensor
AI 理解论文
溯源树
样例
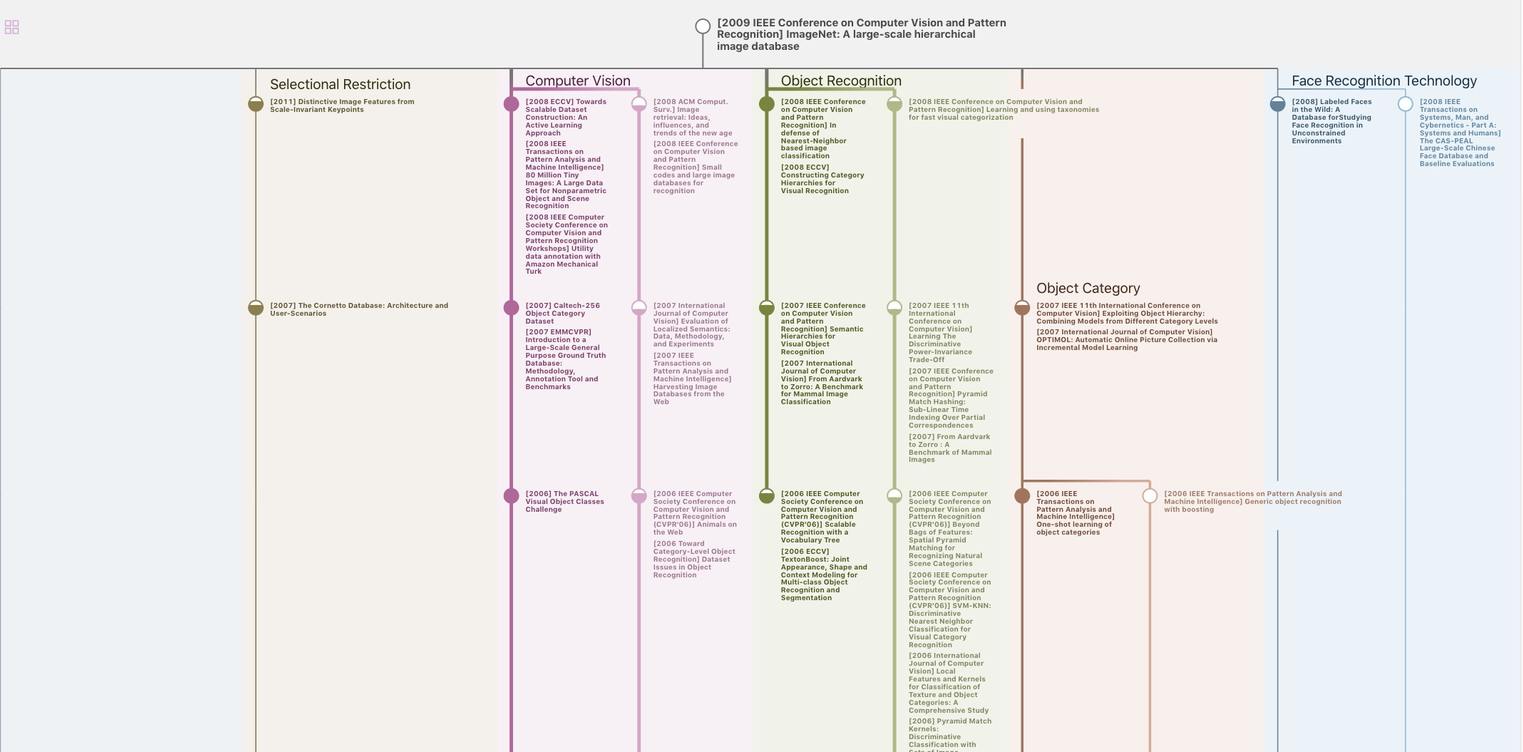
生成溯源树,研究论文发展脉络
Chat Paper
正在生成论文摘要