Multivariate and machine learning approaches for prediction of antioxidant potential in Bertholletia excelsa barks
JOURNAL OF KING SAUD UNIVERSITY SCIENCE(2023)
摘要
Objectives: Specialised metabolites in plants are essential in developing new products since these compounds with antioxidant activity can be incorporated into pharmaceutical and food matrices. However, optimising the extraction processes of these bioactive compounds is necessary for a practical application. The current study aims to obtain optimal models to predict the total phenolic content (TPC) and antioxidant activity (AA) of Bertholletia excelsa barks by the ABTS, DPPH and FRAP methods. Methods: Different algorithms were applied by Machine-Learning (ML), such as Random Forest (RF) and Partial Least-Square Regression (PLSR). Central composite rotational design (CCRD) and response surface methodology (RSM) were used to analyse the ethanol concentration, time and temperature effect on the extraction. This research used five levels for each factor to evaluate the assays. These response variables were used to generate second-order predictive models, and the best conditions for the extraction of bioactive compounds were established. A matrix correlated with the TPC and AA responses was used to generate predictive models by machine learning. Results: The best performance to extract phenolic compounds with antioxidant activity by the ABTS method was achieved when ethanol (50%) at a temperature of 60 degrees C was used for 30 min of extraction. The coefficient of determination of the generated models using RF varied from 85% to 99%, while for the PLSR, the variation was from 85% to 96%. The composition of RGB (red, green, blue) images can be used to determine the TPC and AA in extracts of B. excelsa. Conclusion: The results indicated that the current method was a powerful and effective tool for determining the AA and TPC on B. excelsa barks. In addition, this study permitted to development of a low operational cost method that can help determine the antioxidant activity of plant extracts by digital images. (c) 2023 The Authors. Published by Elsevier B.V. on behalf of King Saud University. This is an open access article under the CC BY-NC-ND license (http://creativecommons.org/licenses/by-nc-nd/4.0/).
更多查看译文
关键词
Digital images,Antioxidant,Computer vision
AI 理解论文
溯源树
样例
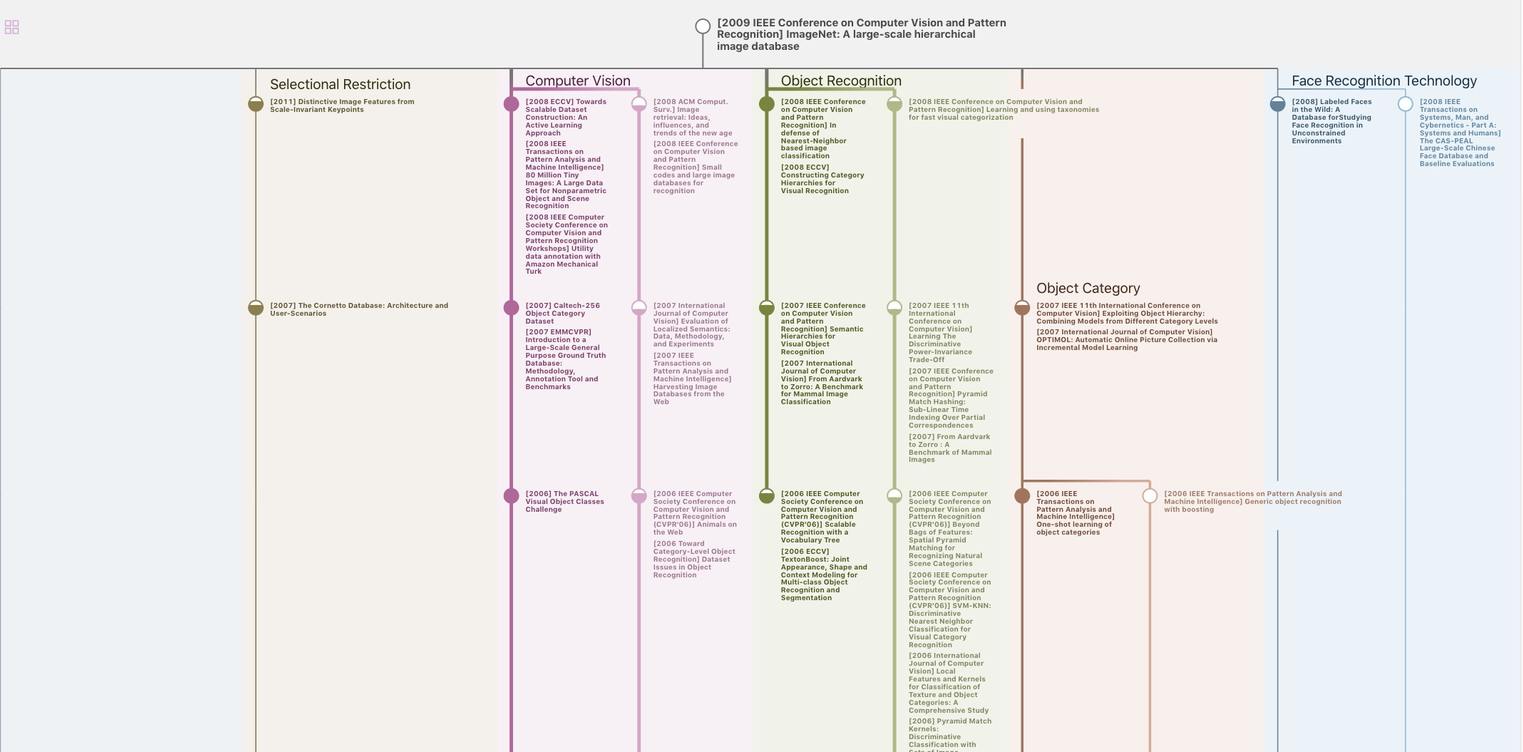
生成溯源树,研究论文发展脉络
Chat Paper
正在生成论文摘要