Text-to-feature diffusion for audio-visual few-shot learning
arXiv (Cornell University)(2023)
摘要
Training deep learning models for video classification from audio-visual data commonly requires immense amounts of labeled training data collected via a costly process. A challenging and underexplored, yet much cheaper, setup is few-shot learning from video data. In particular, the inherently multi-modal nature of video data with sound and visual information has not been leveraged extensively for the few-shot video classification task. Therefore, we introduce a unified audio-visual few-shot video classification benchmark on three datasets, i.e. the VGGSound-FSL, UCF-FSL, ActivityNet-FSL datasets, where we adapt and compare ten methods. In addition, we propose AV-DIFF, a text-to-feature diffusion framework, which first fuses the temporal and audio-visual features via cross-modal attention and then generates multi-modal features for the novel classes. We show that AV-DIFF obtains state-of-the-art performance on our proposed benchmark for audio-visual (generalised) few-shot learning. Our benchmark paves the way for effective audio-visual classification when only limited labeled data is available. Code and data are available at https://github.com/ExplainableML/AVDIFF-GFSL.
更多查看译文
关键词
learning,diffusion,text-to-feature,audio-visual,few-shot
AI 理解论文
溯源树
样例
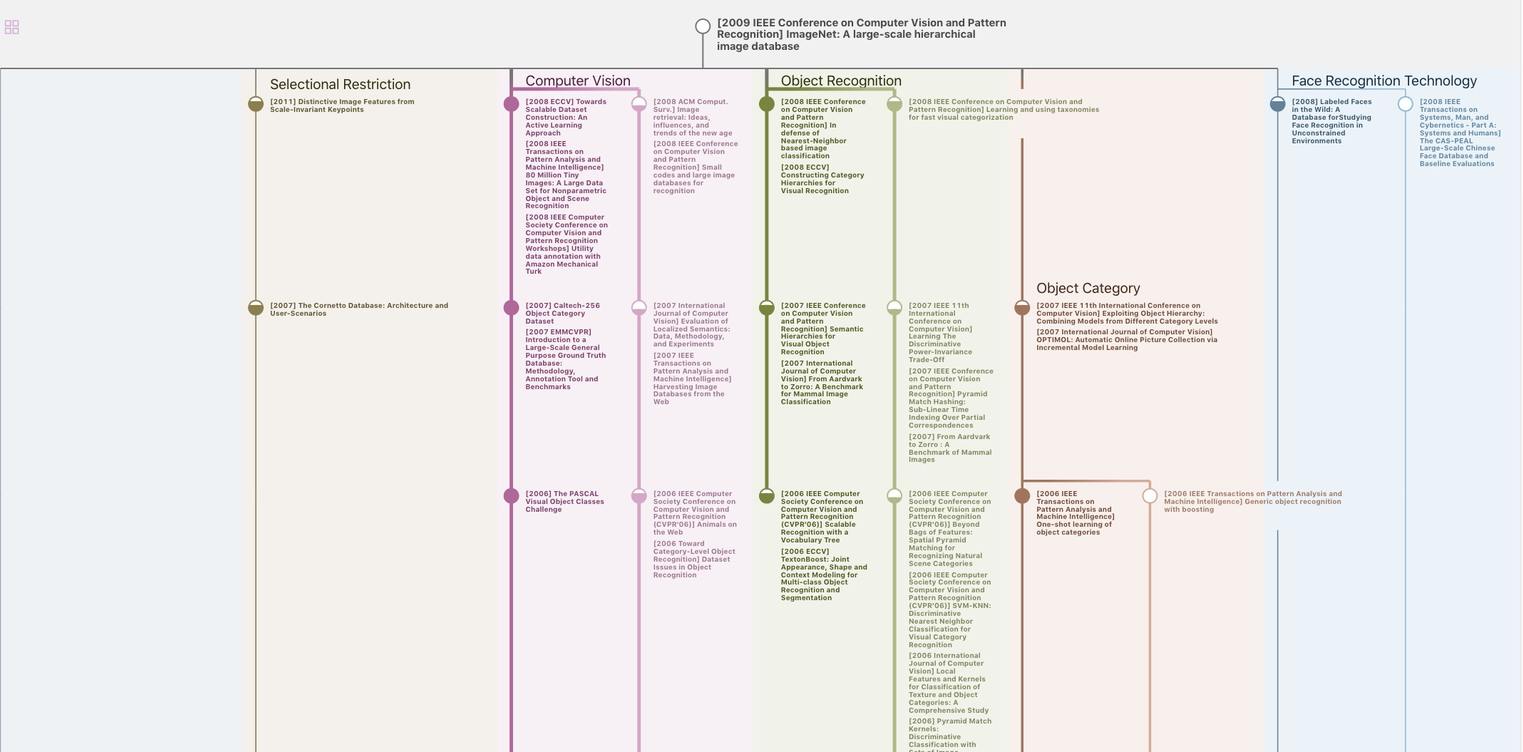
生成溯源树,研究论文发展脉络
Chat Paper
正在生成论文摘要