Uncovering Drift in Textual Data: An Unsupervised Method for Detecting and Mitigating Drift in Machine Learning Models
CoRR(2023)
摘要
Drift in machine learning refers to the phenomenon where the statistical properties of data or context, in which the model operates, change over time leading to a decrease in its performance. Therefore, maintaining a constant monitoring process for machine learning model performance is crucial in order to proactively prevent any potential performance regression. However, supervised drift detection methods require human annotation and consequently lead to a longer time to detect and mitigate the drift. In our proposed unsupervised drift detection method, we follow a two step process. Our first step involves encoding a sample of production data as the target distribution, and the model training data as the reference distribution. In the second step, we employ a kernel-based statistical test that utilizes the maximum mean discrepancy (MMD) distance metric to compare the reference and target distributions and estimate any potential drift. Our method also identifies the subset of production data that is the root cause of the drift. The models retrained using these identified high drift samples show improved performance on online customer experience quality metrics.
更多查看译文
关键词
drift,textual data,machine learning models,machine learning,unsupervised method
AI 理解论文
溯源树
样例
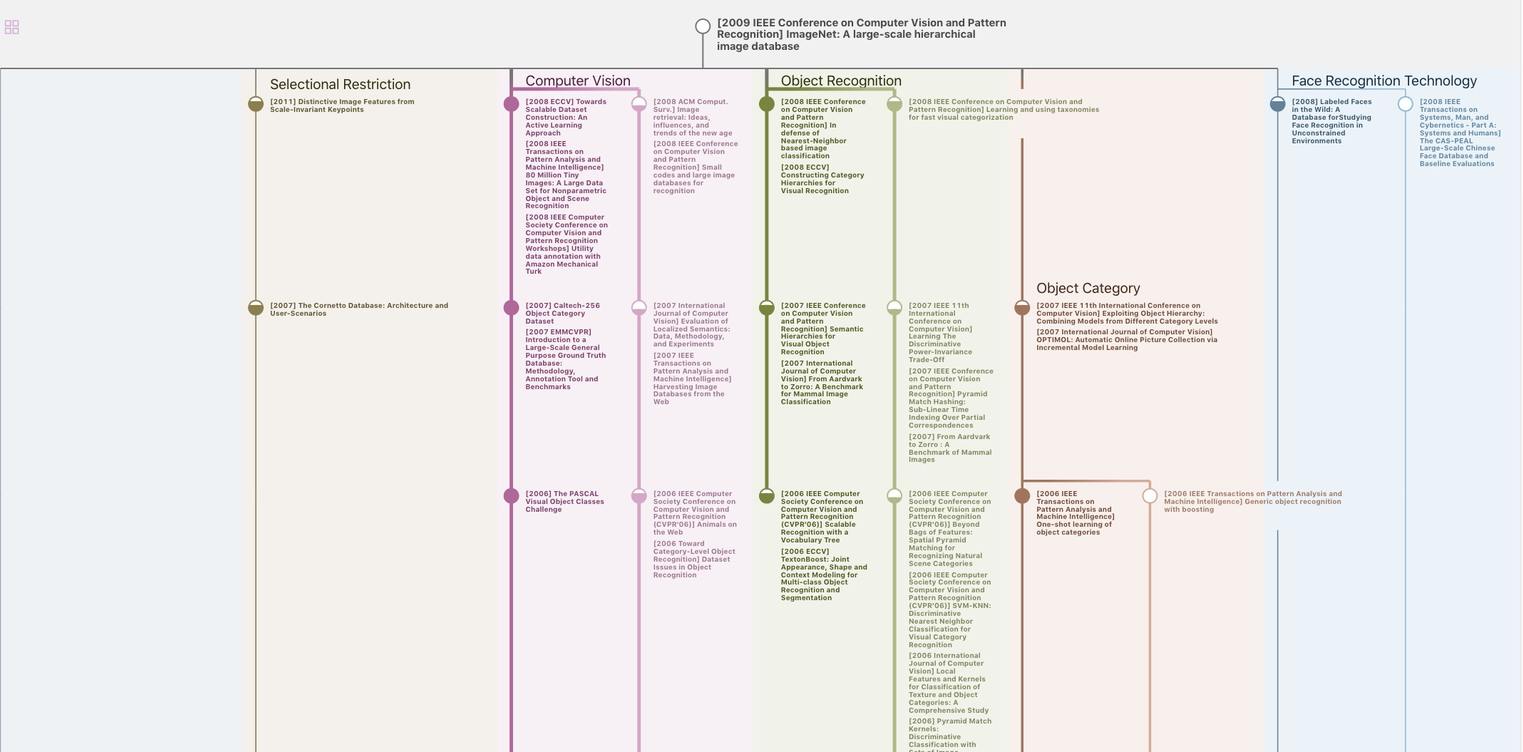
生成溯源树,研究论文发展脉络
Chat Paper
正在生成论文摘要