Interpretable visual question answering via reasoning supervision
2023 IEEE INTERNATIONAL CONFERENCE ON IMAGE PROCESSING, ICIP(2023)
摘要
Transformer-based architectures have recently demonstrated remarkable performance in the Visual Question Answering (VQA) task. However, such models are likely to disregard crucial visual cues and often rely on multimodal shortcuts and inherent biases of the language modality to predict the correct answer, a phenomenon commonly referred to as lack of visual grounding. In this work, we alleviate this shortcoming through a novel architecture for visual question answering that leverages common sense reasoning as a supervisory signal. Reasoning supervision takes the form of a textual justification of the correct answer, with such annotations being already available on large-scale Visual Common Sense Reasoning (VCR) datasets. The model's visual attention is guided toward important elements of the scene through a similarity loss that aligns the learned attention distributions guided by the question and the correct reasoning. We demonstrate both quantitatively and qualitatively that the proposed approach can boost the model's visual perception capability and lead to performance increase, without requiring training on explicit grounding annotations.
更多查看译文
关键词
Visual Question Answering,Visual Grounding,Interpretability,Attention Similarity
AI 理解论文
溯源树
样例
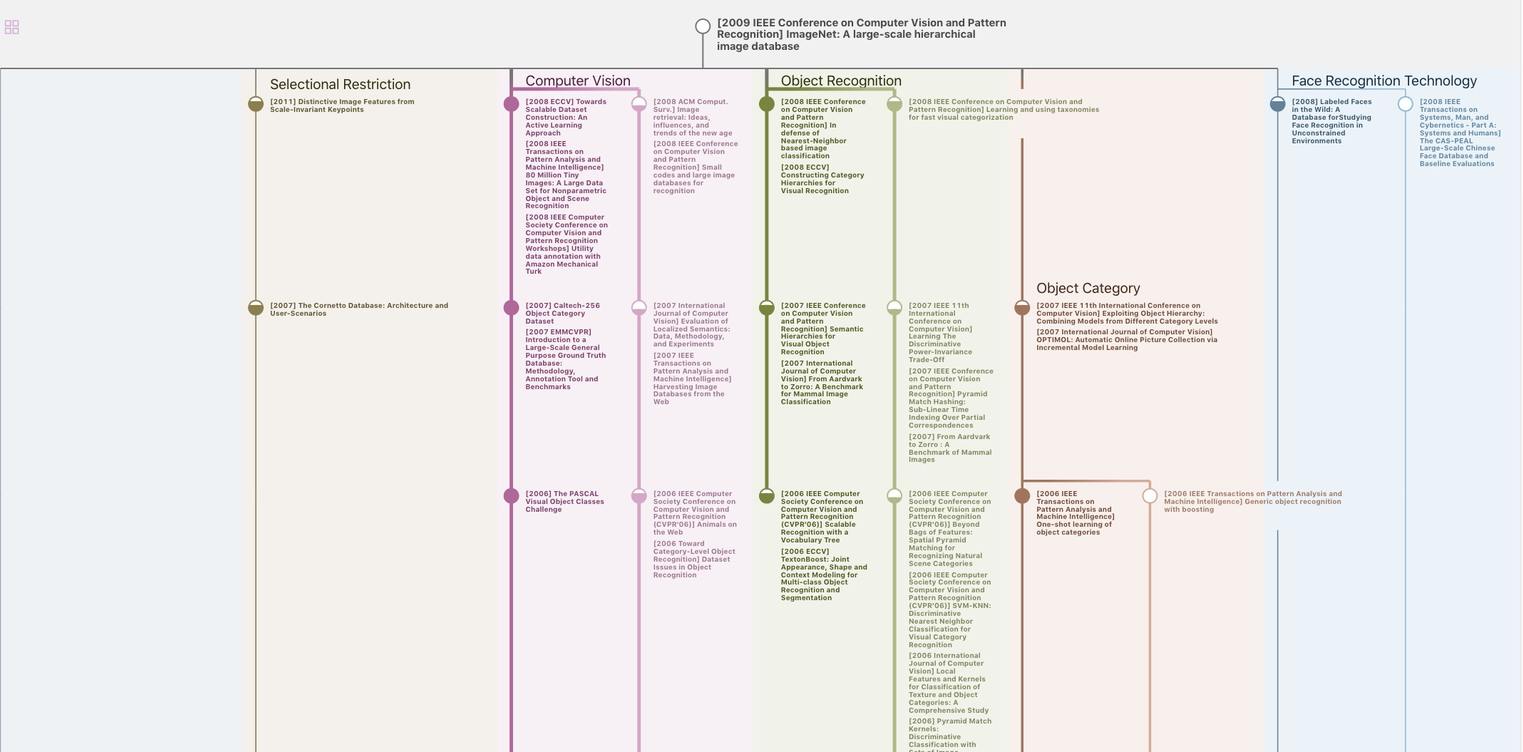
生成溯源树,研究论文发展脉络
Chat Paper
正在生成论文摘要