A term extraction algorithm based on machine learning and comprehensive feature strategy
Neural Computing and Applications(2024)
摘要
Manual term extraction is similar to literal meaning: A translator browses text, classifies words, and prepares for translation. Terminology, as a centralized carrier of expertise, creation, popularization, and disappearance, dynamically reflects the development and evolution of an industry. The automatic extraction of terminology is a key technology for creating a professional terminology database, and it is also a key topic in the field of natural language processing. The purpose of this paper is to study how to analyse a term extraction algorithm based on machine learning and a comprehensive feature strategy. Focusing on the problems of poor generality and single statistical features of current term extraction algorithms, this paper proposes an improved domain ontology term extraction algorithm based on a comprehensive feature strategy. Moreover, automatic term extraction experiments based on a word-based maximum entropy model and a conditional random field model based on machine learning are conducted in this paper. Its word-based conditional random field model outperforms the maximum entropy model. The experimental results show that the algorithm based on the comprehensive feature strategy improves the accuracy by 8.6% compared with the TF-IDF algorithm and the C -value term extraction algorithm. This algorithm can be used to effectively extract the terms in a text and has good generality.
更多查看译文
关键词
Term extraction,Comprehensive feature strategy,Rules and statistics
AI 理解论文
溯源树
样例
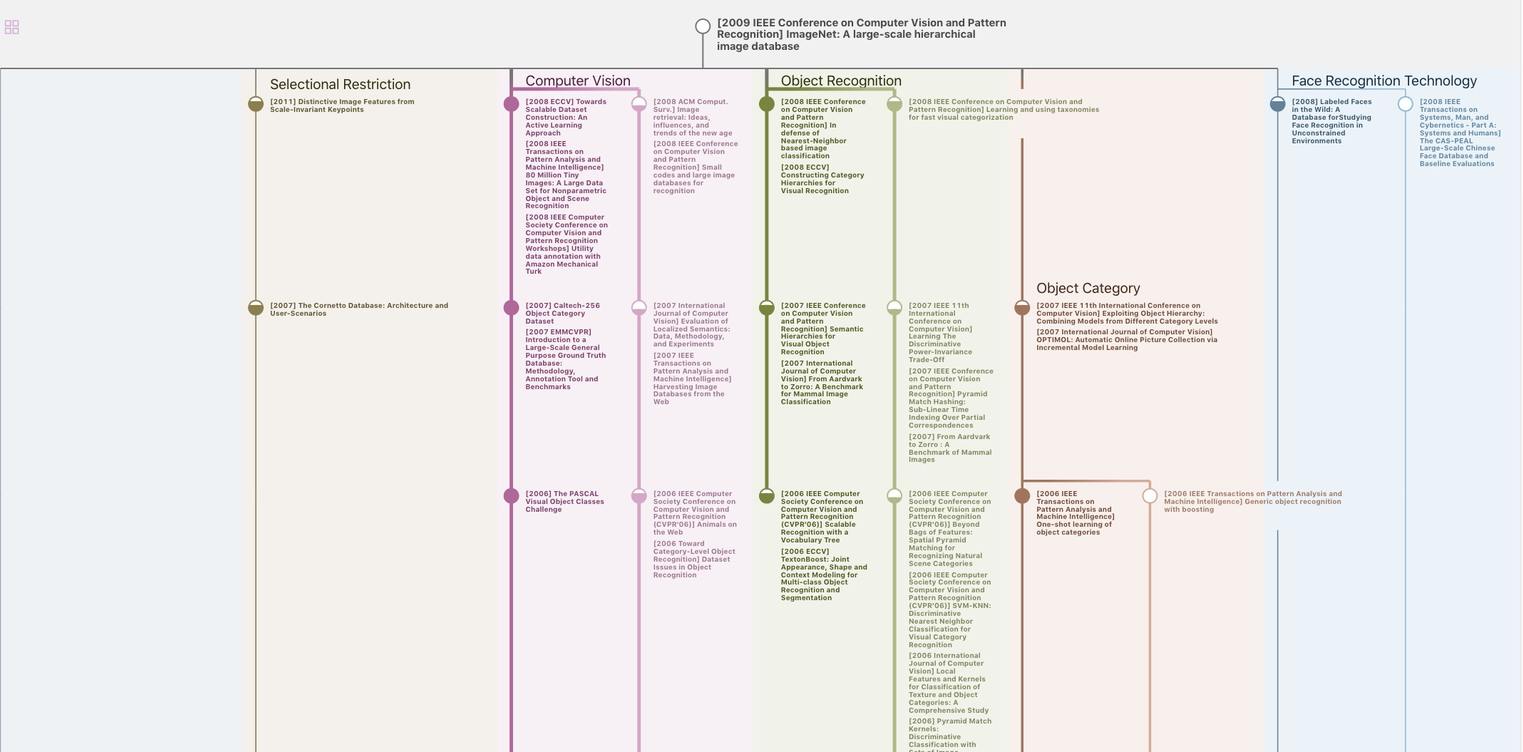
生成溯源树,研究论文发展脉络
Chat Paper
正在生成论文摘要