Compact Model Parameter Extraction using Bayesian Machine Learning
2023 IEEE Computer Society Annual Symposium on VLSI (ISVLSI)(2023)
摘要
Compact models are integral part of large-scale integrated circuit simulations and validation of new technologies. With technology scaling, however, compact models have become complex with lots of parameters involved. Hence, parameter extraction for new device technology is rather challenging. In this paper, we propose a probabilistic approach to compact model parameter extraction. We devise a Bayesian optimization technique which is specifically tailored for efficient extraction of BSIMCMG parameters for fitting nanowire junctionless transistors and 14nm FinFETs. The Bayesian optimization based extraction results show excellent fit to drain current data, with 6.5% normalized root-mean-square error for nanowire junctionless transistors. For a 14nm FinFET, the technique achieves 6.3% and 1.5% for drain current and capacitance data, respectively. This compares favourably to current tools available as well and improves on current tools available including industrial ones.
更多查看译文
关键词
Compact model, Parameter extraction, Bayesian optimization, Machine Learning
AI 理解论文
溯源树
样例
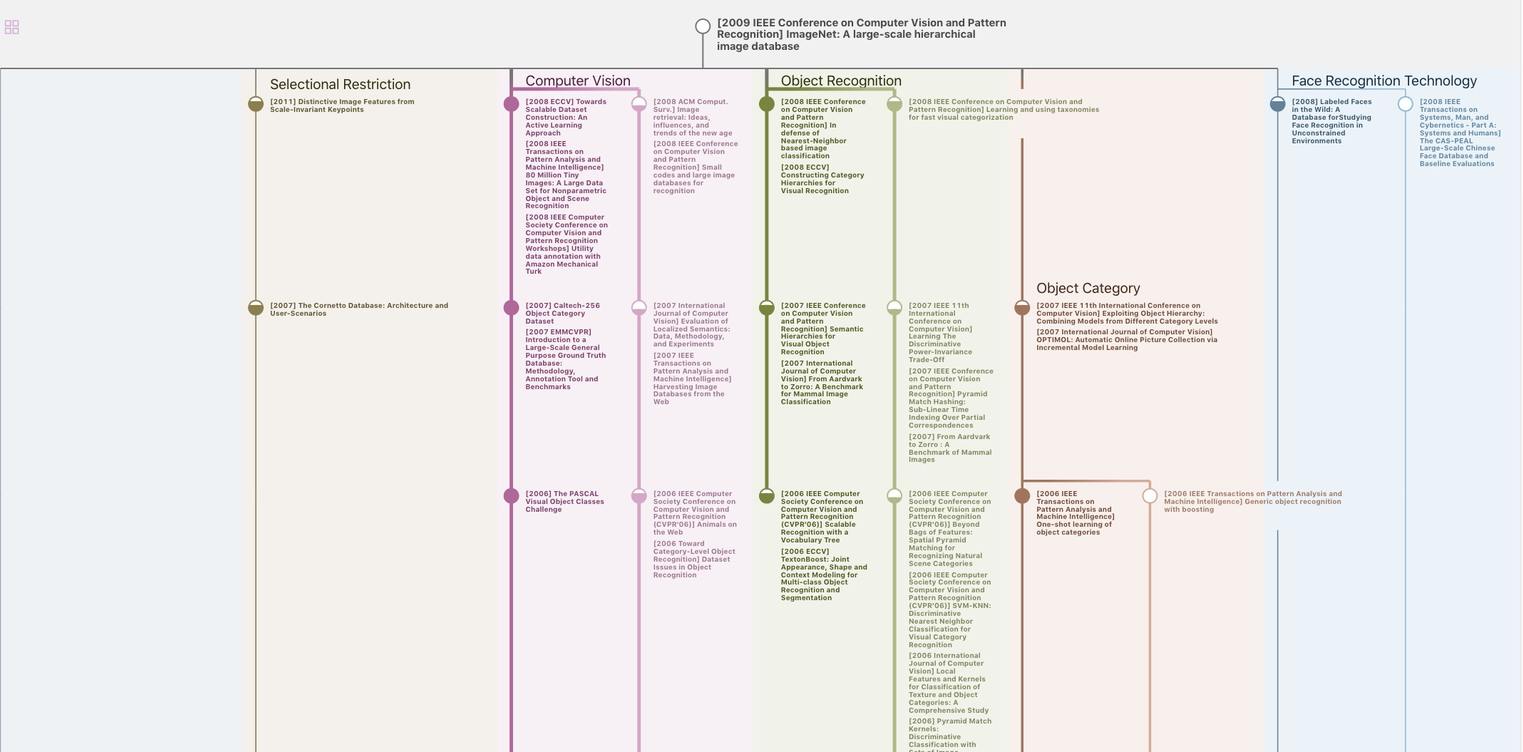
生成溯源树,研究论文发展脉络
Chat Paper
正在生成论文摘要