Codesigned Communication and Data Analytics for Condition-Based Maintenance in Smart Buildings
IEEE Internet of Things Journal(2023)
摘要
With the proliferation of cheap sensors and the ubiquity of cloud and edge computing, predictive/condition-based maintenance is expected to play an important role in smart homes and buildings. Nevertheless, a key difficulty is ensuring that sensors provide data of sufficient quality in order to reliably detect building (e.g., heating system) degradation in systems or comfort. At the same time, sensor utilization should be limited as much as possible in order to minimize power consumption, and increase the lifetimes of batteries. A solution to this problem requires careful codesign of sensor communication and data analytics. In this article, we introduce a formulation of this codesign problem, which is based on an optimization problem to jointly design how often data is collected and compression levels in order to balance the quality of fault detection with the quantity of transmitted data. To solve the optimization problem, we apply a differentiable search algorithm based on a variant of stochastic gradient descent for discrete optimization problems. We apply our codesign framework and solve the resulting optimization problem using data obtained from a building comfort experiment known as the Twin House Experiment. We also provide an extension of our algorithm to a dynamic variant of the codesign framework, where comfort levels and power consumption penalties are time varying. Numerical results show that our algorithm rapidly finds an efficient tradeoff between classifier accuracy and sensor power consumption.
更多查看译文
关键词
Origin-destination prediction,deep learning,spatiotemporal feature,temporal convolutional neural network
AI 理解论文
溯源树
样例
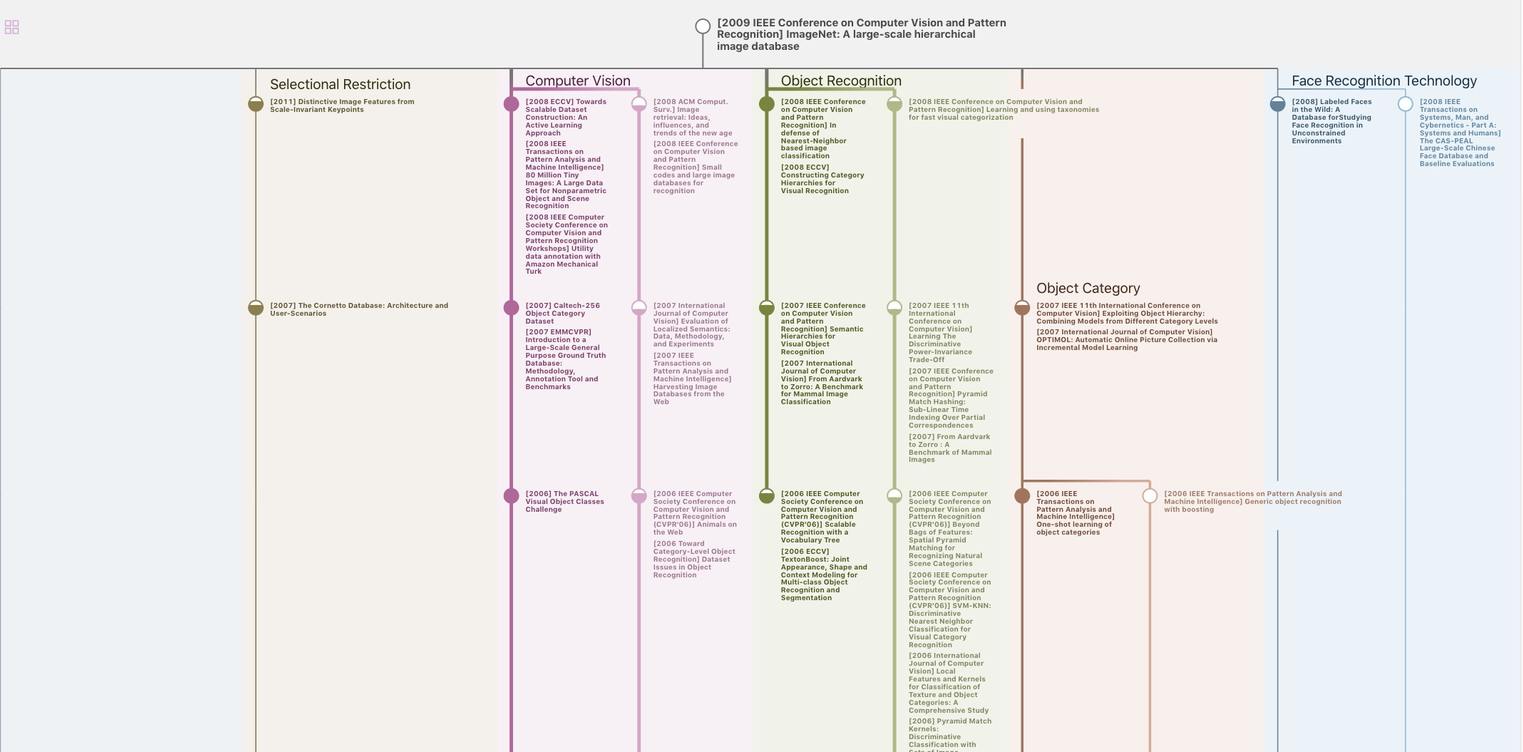
生成溯源树,研究论文发展脉络
Chat Paper
正在生成论文摘要